Permutation-based multiple testing corrections for P $$ P $$ -values and confidence intervals for cluster randomized trials.
Statistics in medicine(2023)
摘要
In this article, we derive and compare methods to derive P-values and sets of confidence intervals with strong control of the family-wise error rates and coverage for estimates of treatment effects in cluster randomized trials with multiple outcomes. There are few methods for P-value corrections and deriving confidence intervals, limiting their application in this setting. We discuss the methods of Bonferroni, Holm, and Romano and Wolf and adapt them to cluster randomized trial inference using permutation-based methods with different test statistics. We develop a novel search procedure for confidence set limits using permutation tests to produce a set of confidence intervals under each method of correction. We conduct a simulation-based study to compare family-wise error rates, coverage of confidence sets, and the efficiency of each procedure in comparison to no correction using both model-based standard errors and permutation tests. We show that the Romano-Wolf type procedure has nominal error rates and coverage under non-independent correlation structures and is more efficient than the other methods in a simulation-based study. We also compare results from the analysis of a real-world trial.
更多查看译文
关键词
cluster randomized trial,coverage,inference,multiple testing
AI 理解论文
溯源树
样例
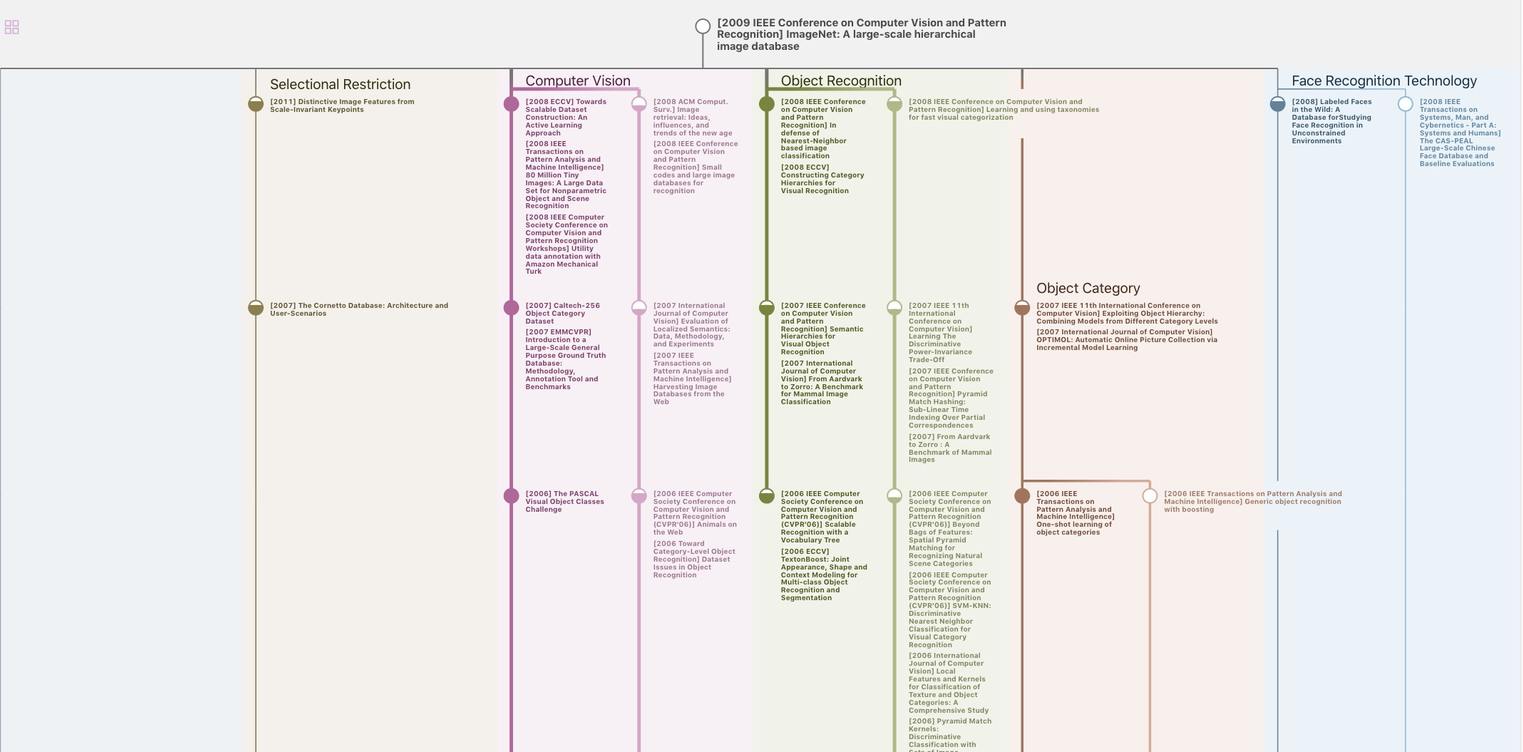
生成溯源树,研究论文发展脉络
Chat Paper
正在生成论文摘要