Regularized Classification-Aware Quantization
30th Biennial Symposium on Communications 2021(2022)
摘要
Traditionally, quantization is designed to minimize the reconstruction error of a data source. When considering downstream classification tasks, other measures of distortion can be of interest, such as the 0-1 classification loss. Furthermore, it is desirable that the performance of these quantizers does not deteriorate once they are deployed into production, as re-learning the scheme online is not always possible. In this chapter, we present a class of algorithms that learn distributed quantization schemes for binary classification tasks. Our method performs well on unseen data and is faster than previous methods proportional to a quadratic term of the dataset size. It works by regularizing the 0-1 loss with the reconstruction error. We present experiments on synthetic mixture and bivariate Gaussian data and compare training, testing, and generalization errors with a family of benchmark quantization schemes from the literature. Our method is called Regularized Classification-Aware Quantization.
更多查看译文
关键词
Distributed quantization, Generalization, Regularization, Classification
AI 理解论文
溯源树
样例
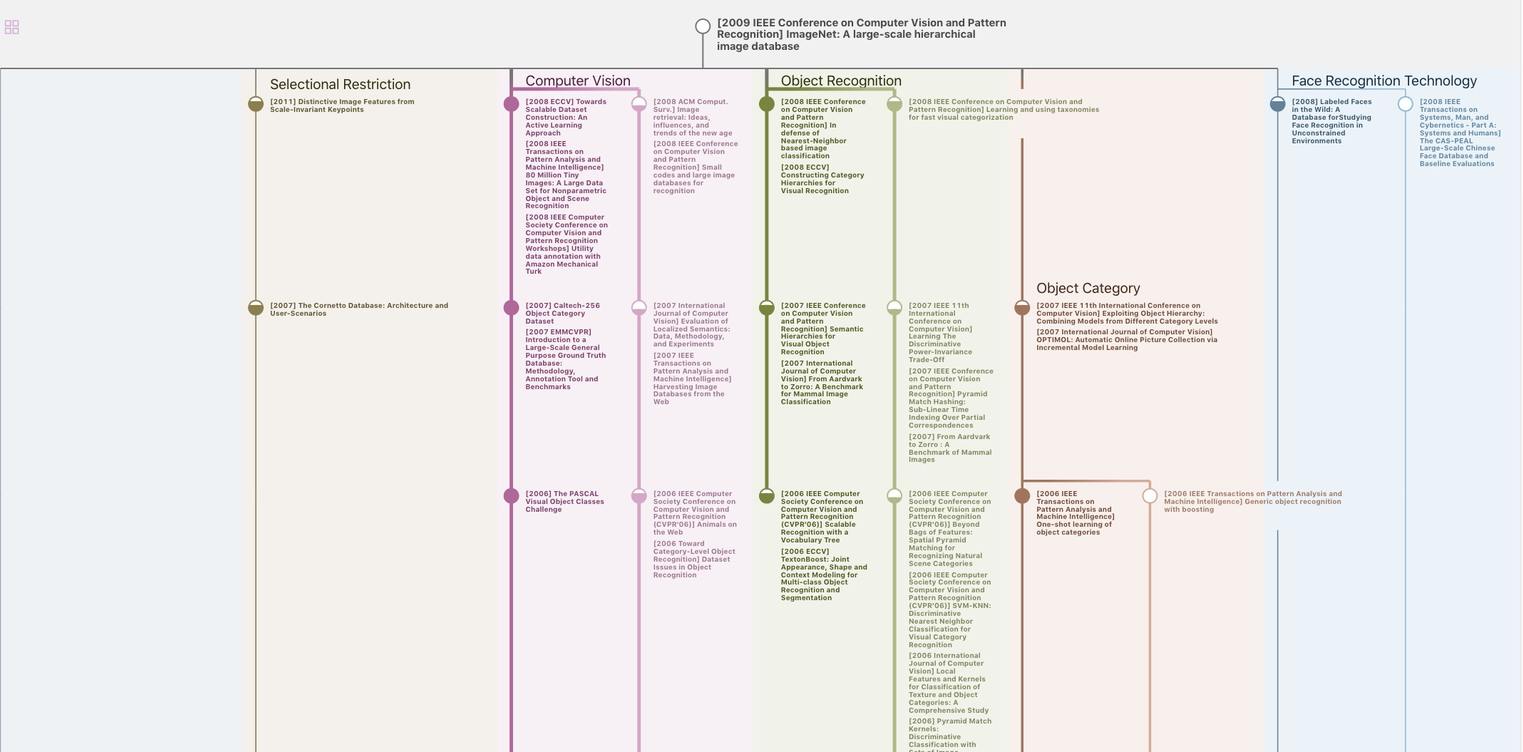
生成溯源树,研究论文发展脉络
Chat Paper
正在生成论文摘要