Improving detection accuracy of perfusion defect in standard dose SPECT-myocardial perfusion imaging by deep-learning denoising
Journal of Nuclear Cardiology(2021)
Abstract
Background We previously developed a deep-learning (DL) network for image denoising in SPECT-myocardial perfusion imaging (MPI). Here we investigate whether this DL network can be utilized for improving detection of perfusion defects in standard-dose clinical acquisitions. Methods To quantify perfusion-defect detection accuracy, we conducted a receiver-operating characteristic (ROC) analysis on reconstructed images with and without processing by the DL network using a set of clinical SPECT-MPI data from 190 subjects. For perfusion-defect detection hybrid studies were used as ground truth, which were created from clinically normal studies with simulated realistic lesions inserted. We considered ordered-subset expectation-maximization (OSEM) reconstruction with corrections for attenuation, resolution, and scatter and with 3D Gaussian post-filtering. Total perfusion deficit (TPD) scores, computed by Quantitative Perfusion SPECT (QPS) software, were used to evaluate the reconstructed images. Results Compared to reconstruction with optimal Gaussian post-filtering (sigma = 1.2 voxels), further DL denoising increased the area under the ROC curve (AUC) from 0.80 to 0.88 ( P -value < 10 −4 ). For reconstruction with less Gaussian post-filtering (sigma = 0.8 voxels), thus better spatial resolution, DL denoising increased the AUC value from 0.78 to 0.86 ( P -value < 10 −4 ) and achieved better spatial resolution in reconstruction. Conclusions DL denoising can effectively improve the detection of abnormal defects in standard-dose SPECT-MPI images over conventional reconstruction.
MoreTranslated text
Key words
SPECT-MPI, post-reconstruction filtering, deep learning, noise-to-noise training
AI Read Science
Must-Reading Tree
Example
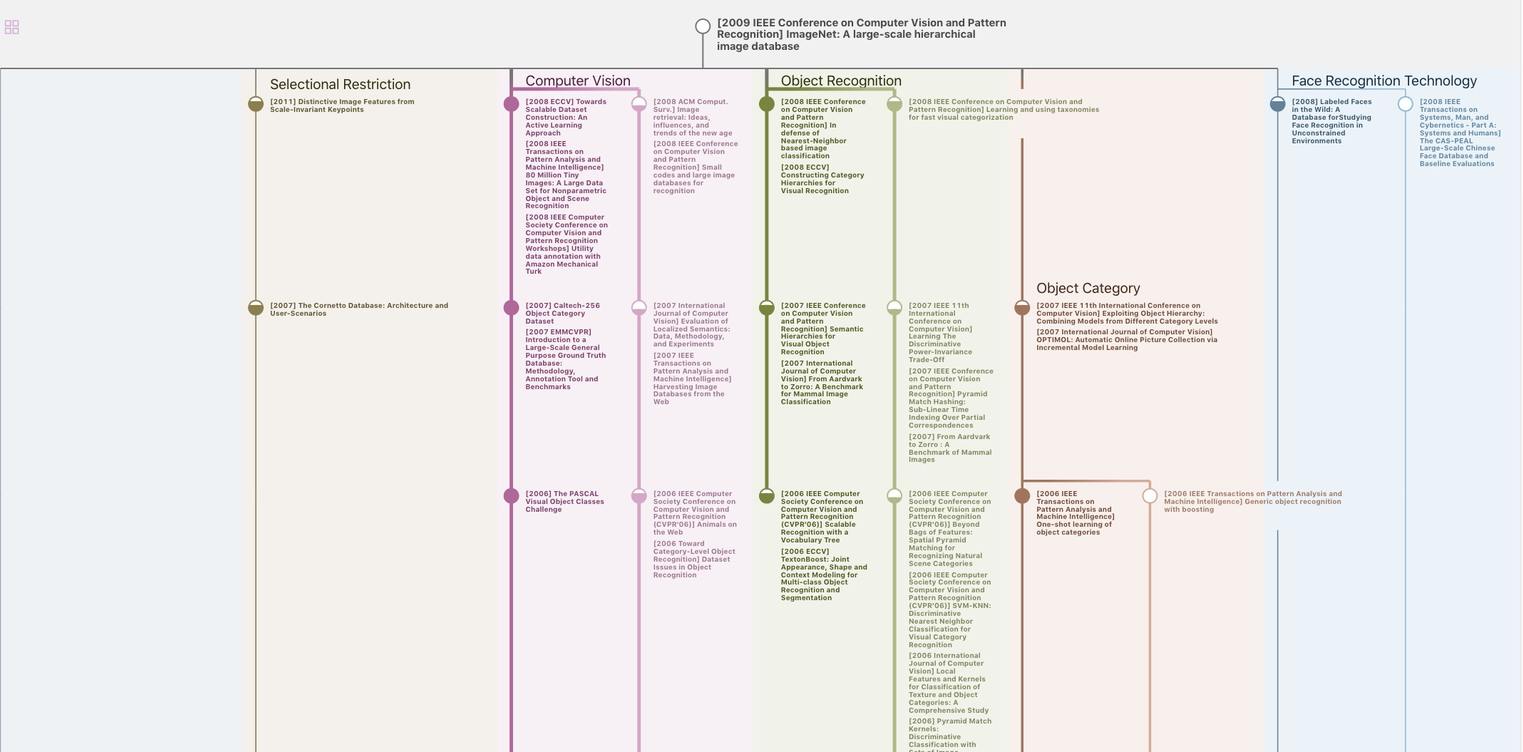
Generate MRT to find the research sequence of this paper
Chat Paper
Summary is being generated by the instructions you defined