MemSum: Extractive Summarization of Long Documents Using Multi-Step Episodic Markov Decision Processes
PROCEEDINGS OF THE 60TH ANNUAL MEETING OF THE ASSOCIATION FOR COMPUTATIONAL LINGUISTICS (ACL 2022), VOL 1: (LONG PAPERS)(2022)
摘要
We introduce MemSum (Multi-step Episodic Markov decision process extractive SUMmarizer), a reinforcement-learning-based extractive summarizer enriched at each step with information on the current extraction history. When MemSum iteratively selects sentences into the summary, it considers a broad information set that would intuitively also be used by humans in this task: 1) the text content of the sentence, 2) the global text context of the rest of the document, and 3) the extraction history consisting of the set of sentences that have already been extracted. With a lightweight architecture, MemSum obtains state-of-the-art test-set performance (ROUGE) in summarizing long documents taken from PubMed, arXiv, and GovReport. Ablation studies demonstrate the importance of local, global, and history information. A human evaluation confirms the high quality and low redundancy of the generated summaries, stemming from MemSum's awareness of extraction history.
更多查看译文
关键词
extractive summarization,long documents,memsum,markov,multi-step
AI 理解论文
溯源树
样例
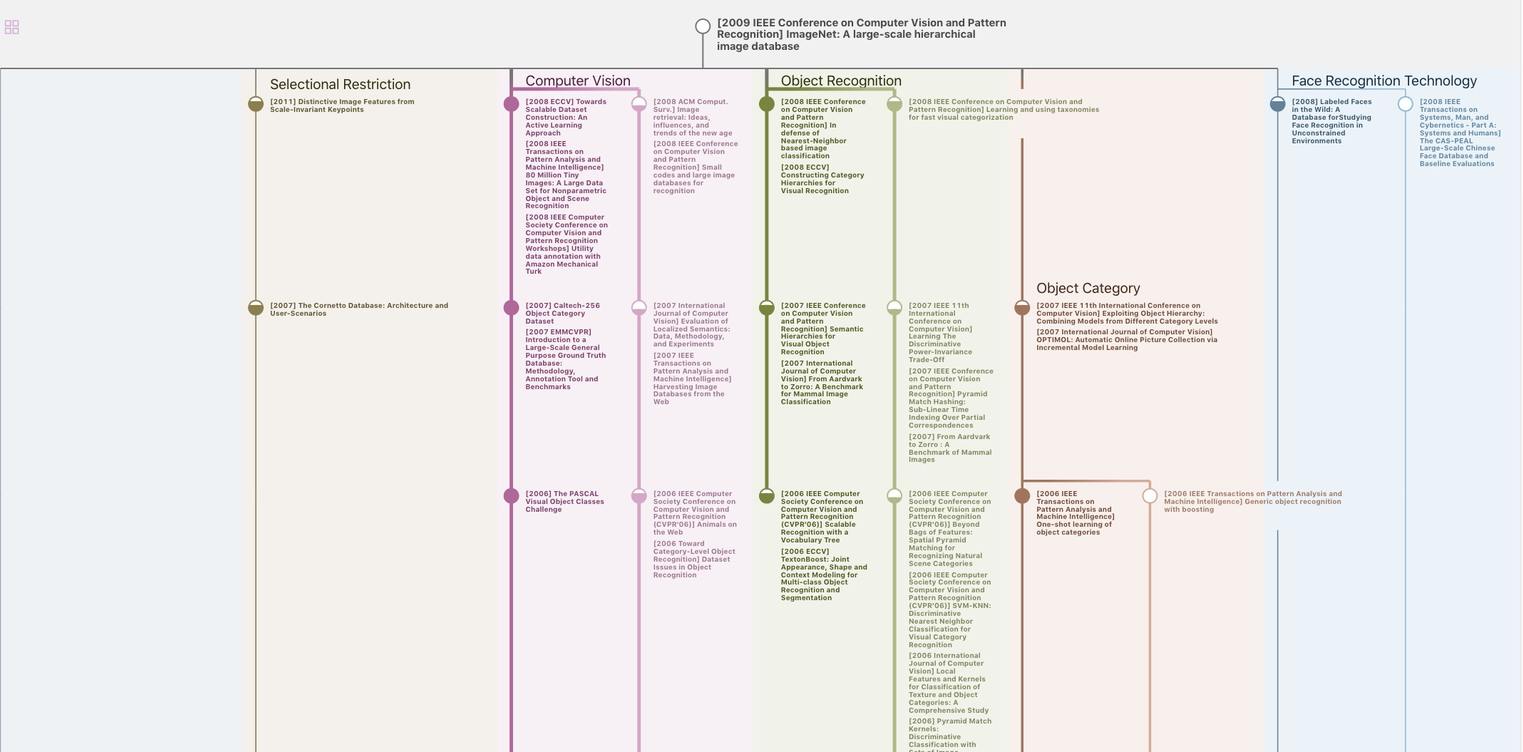
生成溯源树,研究论文发展脉络
Chat Paper
正在生成论文摘要