Equivariant Manifold Flows.
Annual Conference on Neural Information Processing Systems(2021)
Abstract
Tractably modelling distributions over manifolds has long been an important goal in the natural sciences. Recent work has focused on developing general machine learning models to learn such distributions. However, for many applications these distributions must respect manifold symmetries—a trait which most previous models disregard. In this paper, we lay the theoretical foundations for learning symmetry-invariant distributions on arbitrary manifolds via equivariant manifold flows. We demonstrate the utility of our approach by learning quantum field theory-motivated invariant SU(n) densities and by correcting meteor impact dataset bias.
MoreTranslated text
Key words
equivariant manifold flows
AI Read Science
Must-Reading Tree
Example
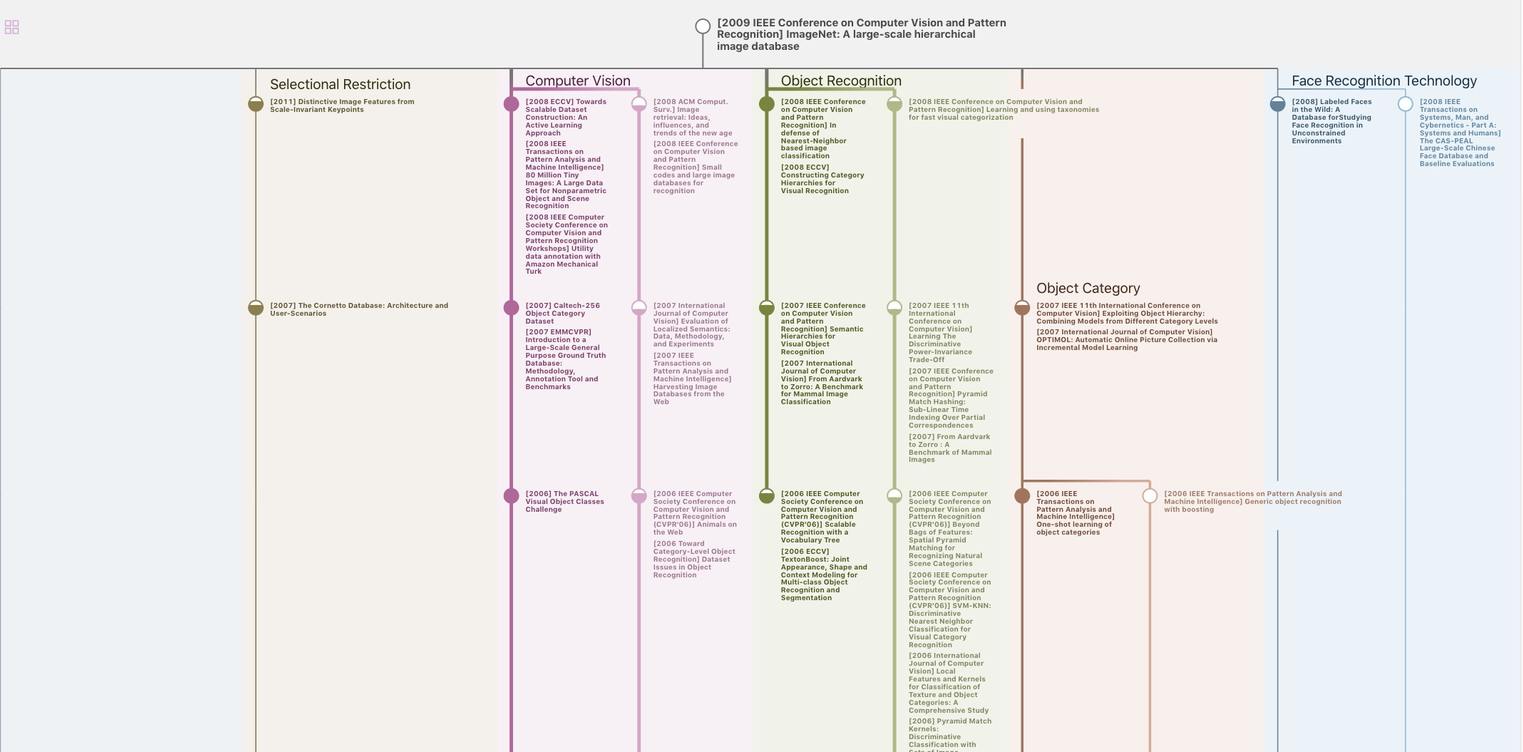
Generate MRT to find the research sequence of this paper
Chat Paper
Summary is being generated by the instructions you defined