The Use Of Explainable Artificial Intelligence To Explore Types Of Fenestral Otosclerosis Misdiagnosed When Using Temporal Bone High-Resolution Computed Tomography
ANNALS OF TRANSLATIONAL MEDICINE(2021)
摘要
Background: The purpose of this study was to explore the common characteristics of fenestral otosclerosis (OS) which are misdiagnosed, and develop a deep learning model for the diagnosis of fenestral OS based on temporal bone high-resolution computed tomography scans. Methods: We conducted a study to explicitly analyze the clinical performance of otolaryngologists in diagnosing fenestral OS and developed an explainable deep learning model using 134,574 temporal bone high-resolution computed tomography (HRCT) slices collected from 1,294 patients for the automatic diagnosis of fenestral OS. We prospectively created an external test set with 31,774 CT slices from 144 patients, which contained 86 fenestral OS ears and 202 normal ears and used it to evaluate the performance of our otosclerosis-Logical Neural Network (LNN) model to assess its potential clinical utility. In addition, we compared the diagnostic acumen of seven otolaryngologists with the otosclerosis-LNN approach in the clinical test set, which was mixed with 78 fenestral OS and 62 normal ears. Finally, to evaluate the assisting value of the model, the seven participants were again invited to classify all cases in the clinical test set after referring to the diagnostic results of the model, to which they were blinded. Results: The diagnostic performance of otologists was not satisfactory, and those CT samples which were misdiagnosed had similar characteristics. Based on this finding, we defined three subtypes of fenestral OS lesions that are suitable for clinical diagnosis guidance: "focal", " transitional", and "typical" fenestral OS. The most encouraging result is that the model achieved an area under the curve (AUC) of 99.5% (per-earsensitivity of 96.4%, per-ear-specificity of 98.9%) on the prospective unknown external test. Furthermore, we used this model to assist otologists and observed a consistent and significant improvement in diagnostic performance, especially for the newly defined focal and transitional fenestral OS, which led to the initial high misdiagnosis rate. Conclusions: Our findings of the fine-grained classification of fenestral OS could have implications for future diagnosis and prevention programs. In addition, our deep OS localization network is an effective approach providing assistance to otologists to deal with the significant challenge of the misdiagnosis of fenestral OS.
更多查看译文
关键词
Fenestral otosclerosis, high-resolution computed tomography, artificial intelligence (AI), deep learning
AI 理解论文
溯源树
样例
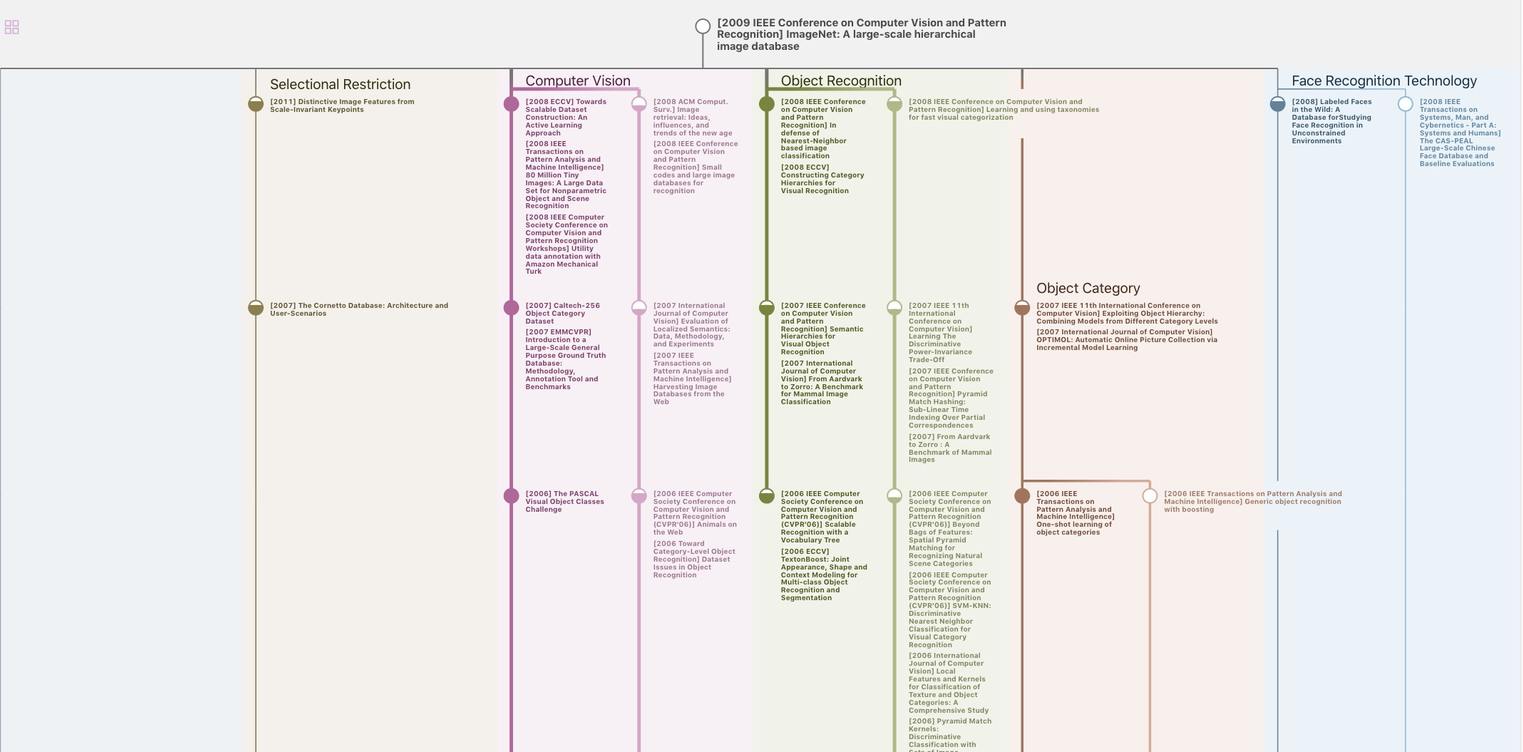
生成溯源树,研究论文发展脉络
Chat Paper
正在生成论文摘要