Representation Consolidation for Training Expert Students
arxiv(2021)
摘要
Traditionally, distillation has been used to train a student model to emulate the input/output functionality of a teacher. A more useful goal than emulation, yet under-explored, is for the student to learn feature representations that transfer well to future tasks. However, we observe that standard distillation of task-specific teachers actually *reduces* the transferability of student representations to downstream tasks. We show that a multi-head, multi-task distillation method using an unlabeled proxy dataset and a generalist teacher is sufficient to consolidate representations from task-specific teacher(s) and improve downstream performance, outperforming the teacher(s) and the strong baseline of ImageNet pretrained features. Our method can also combine the representational knowledge of multiple teachers trained on one or multiple domains into a single model, whose representation is improved on all teachers' domain(s).
更多查看译文
关键词
training expert students,representation
AI 理解论文
溯源树
样例
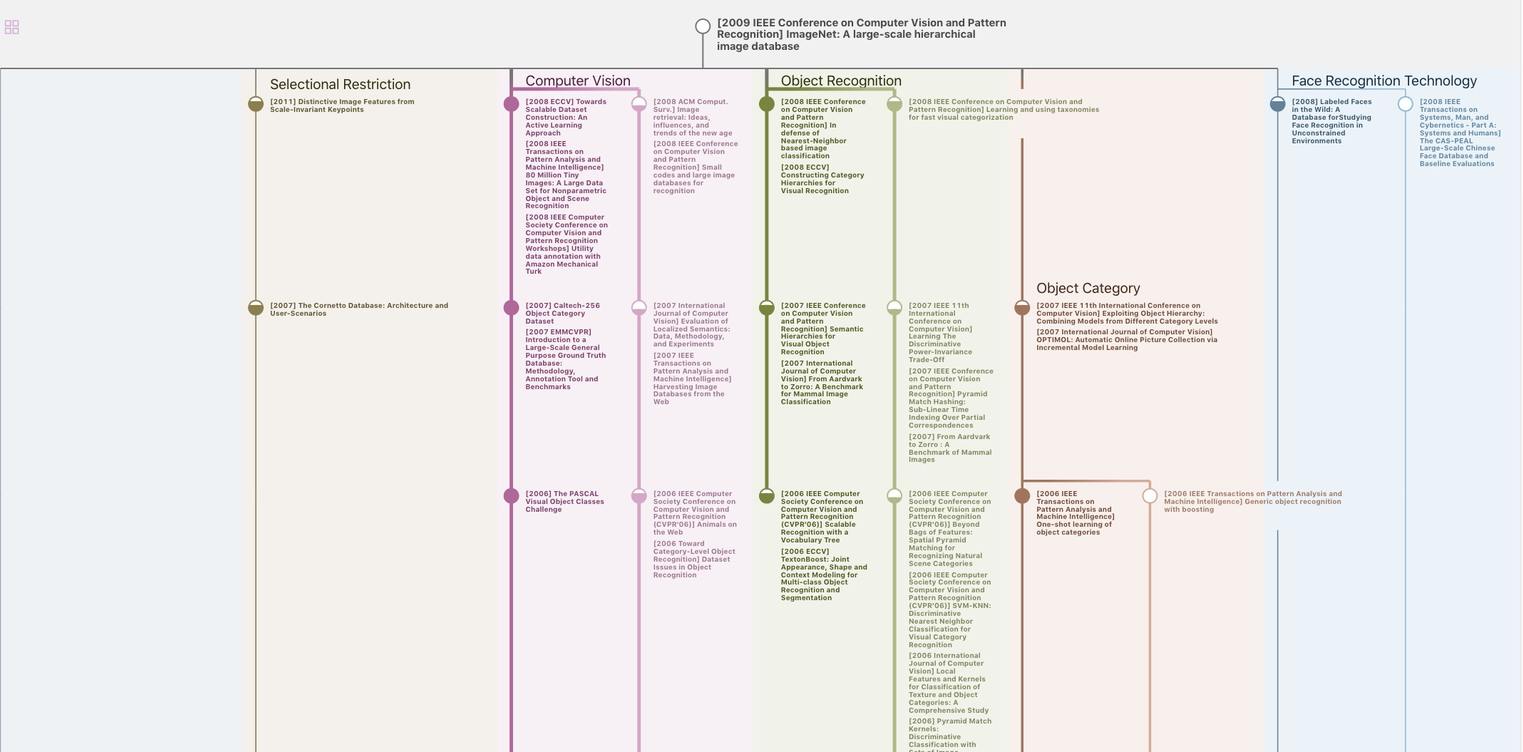
生成溯源树,研究论文发展脉络
Chat Paper
正在生成论文摘要