Assessing The Impact Of Expert Knowledge On Ich M7 (Q)Sar Predictions. Is Expert Review Still Needed?
REGULATORY TOXICOLOGY AND PHARMACOLOGY(2021)
摘要
The ICH M7 (R1) guideline recommends the use of complementary (Q)SAR models to assess the mutagenic potential of drug impurities as a state-of-the-art, high-throughput alternative to empirical testing. Additionally, it includes a provision for the application of expert knowledge to increase prediction confidence and resolve conflicting calls. Expert knowledge, which considers structural analogs and mechanisms of activity, has been valuable when models return an indeterminate (equivocal) result or no prediction (out-of-domain). A retrospective analysis of 1002 impurities evaluated in drug regulatory applications between April 2017 and March 2019 assessed the impact of expert review on (Q)SAR predictions. Expert knowledge overturned the default predictions for 26% of the impurities and resolved 91% of equivocal predictions and 75% of out-of-domain calls. Of the 261 overturned default predictions, 15% were upgraded to equivocal or positive and 79% were downgraded to equivocal or negative. Chemical classes with the most overturns were primary aromatic amines (46%), aldehydes (45%), Michael-reactive acceptors (37%), and non-primary alkyl halides (33%). Additionally, low confidence predictions were the most often overturned. Collectively, the results suggest that expert knowledge continues to play an important role in an ICH M7 (Q)SAR prediction workflow and triaging predictions based on chemical class and probability can improve (Q)SAR review efficiency.
更多查看译文
关键词
Bacterial mutagenicity, Computational toxicology, Drug impurities, ICH M7, (Q)SAR, Expert review, Structure-activity relationship, Ames
AI 理解论文
溯源树
样例
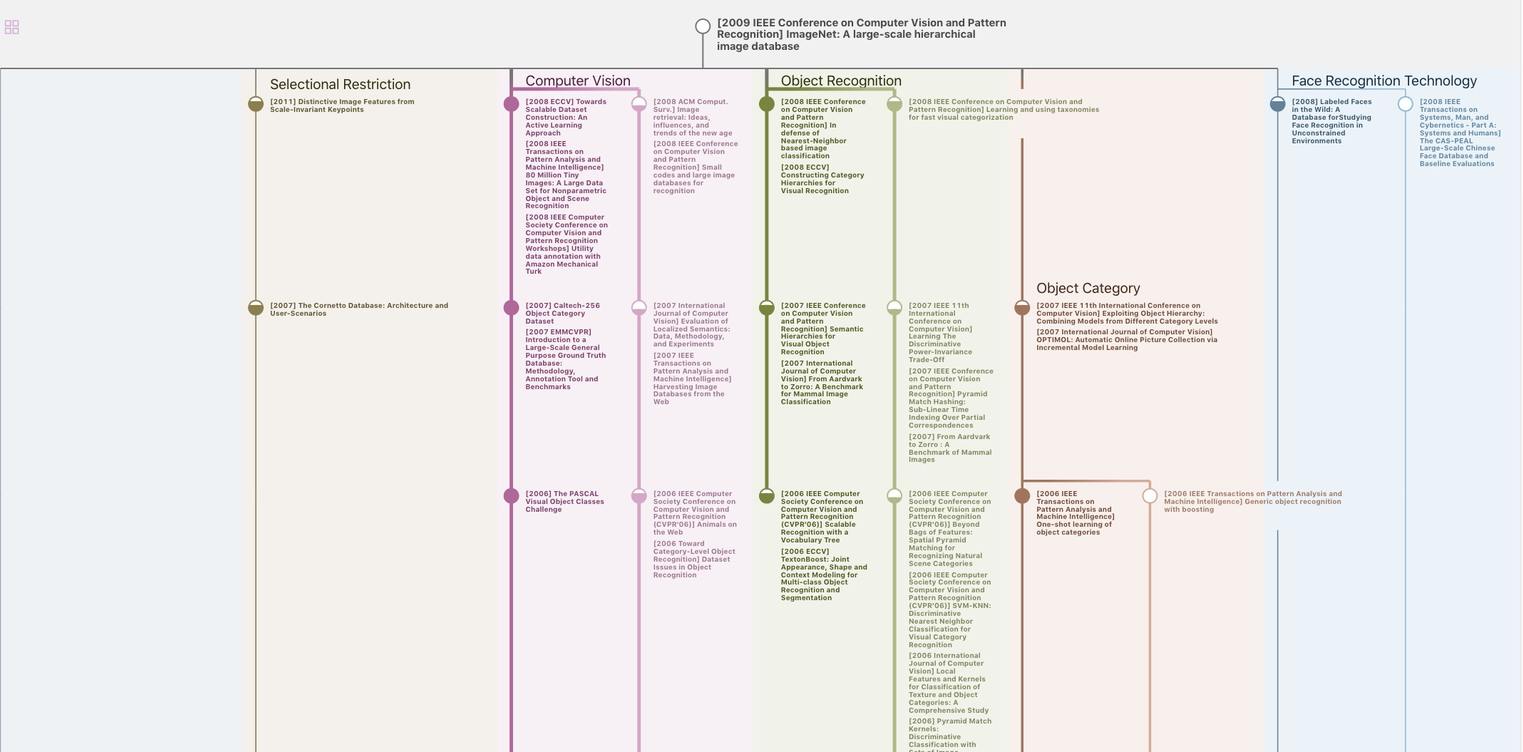
生成溯源树,研究论文发展脉络
Chat Paper
正在生成论文摘要