Parity And Time Reversal Elucidate Both Decision-Making In Empirical Models And Attractor Scaling In Critical Boolean Networks
SCIENCE ADVANCES(2021)
摘要
We present new applications of parity inversion and time reversal to the emergence of complex behavior from simple dynamical rules in stochastic discrete models. Our parity-based encoding of causal relationships and time-reversal construction efficiently reveal discrete analogs of stable and unstable manifolds. We demonstrate their predictive power by studying decision-making in systems biology and statistical physics models. These applications underpin a novel attractor identification algorithm implemented for Boolean networks under stochastic dynamics. Its speed enables resolving a long-standing open question of how attractor count in critical random Boolean networks scales with network size and whether the scaling matches biological observations. Via 80-fold improvement in probed network size (N = 16,384), we find the unexpectedly low scaling exponent of 0.12 +/- 0.05, approximately one-tenth the analytical upper bound. We demonstrate a general principle: A system's relationship to its time reversal and state-space inversion constrains its repertoire of emergent behaviors.
更多查看译文
关键词
attractor scaling,networks,parity,time-reversal,decision-making
AI 理解论文
溯源树
样例
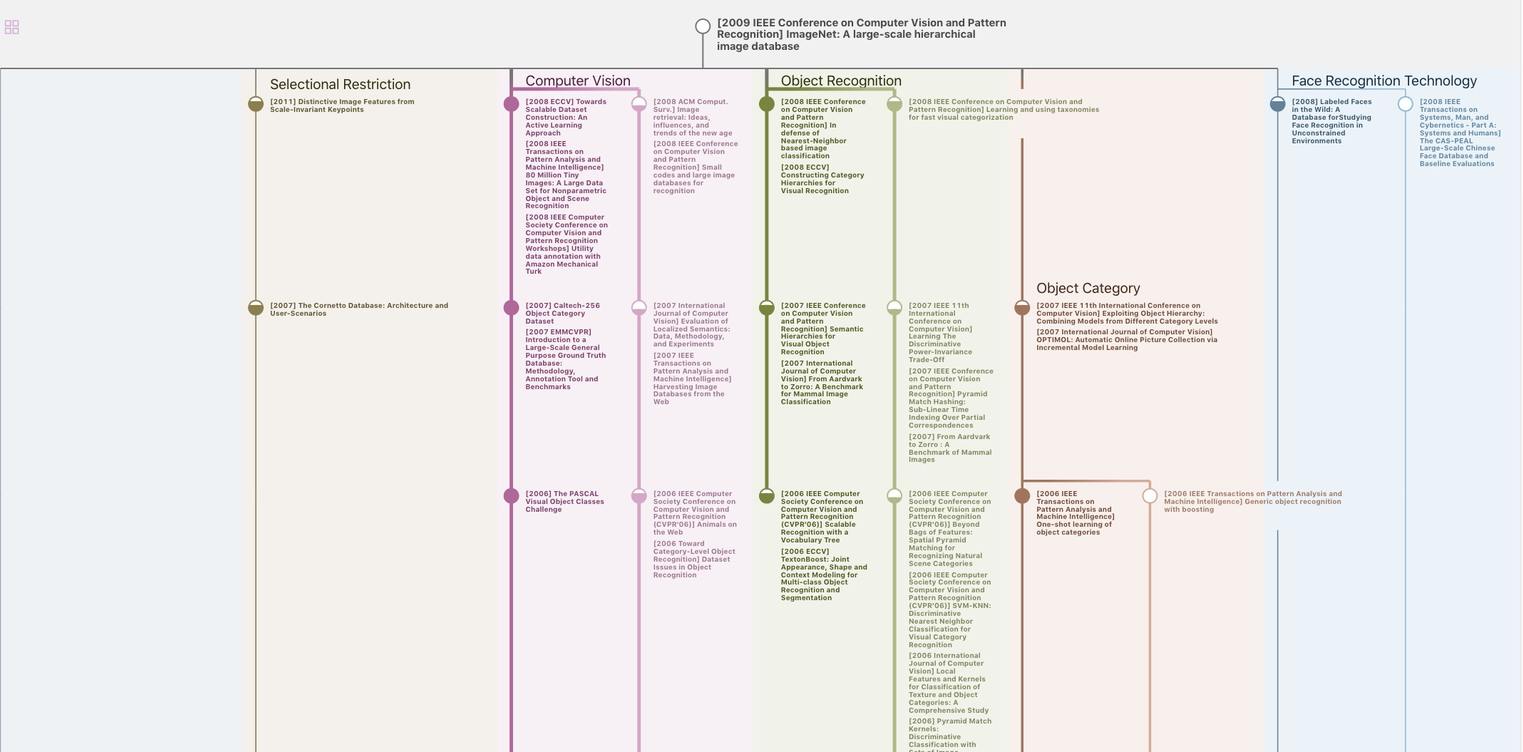
生成溯源树,研究论文发展脉络
Chat Paper
正在生成论文摘要