Warped Input Gaussian Processes for Time Series Forecasting.
CSCML(2021)
摘要
Time series forecasting plays a vital role in system monitoring and novelty detection. However, commonly used forecasting methods are not suited for handling non-stationarity, while existing methods for forecasting in non-stationary time series are often complex to implement and involve expensive computations. We introduce a Gaussian process-based model for handling of non-stationarity. The warping is achieved non-parametrically, through imposing a prior on the relative change of distance between subsequent observation inputs. The model allows the use of general gradient optimization algorithms for training and incurs only a small computational overhead on training and prediction. The model finds its applications in forecasting in non-stationary time series with either gradually varying volatility, presence of change points, or a combination thereof. We implement the model in a probabilistic programming framework, evaluate on synthetic and real-world time series data comparing against both broadly used baselines and known state-of-the-art approaches and show that the model exhibits state-of-the-art forecasting performance at a lower implementation and computation cost, enabling efficient applications in diverse fields of system monitoring and novelty detection..
更多查看译文
关键词
Time series,Probabilistic programming,Non-stationarity,Gaussian processes
AI 理解论文
溯源树
样例
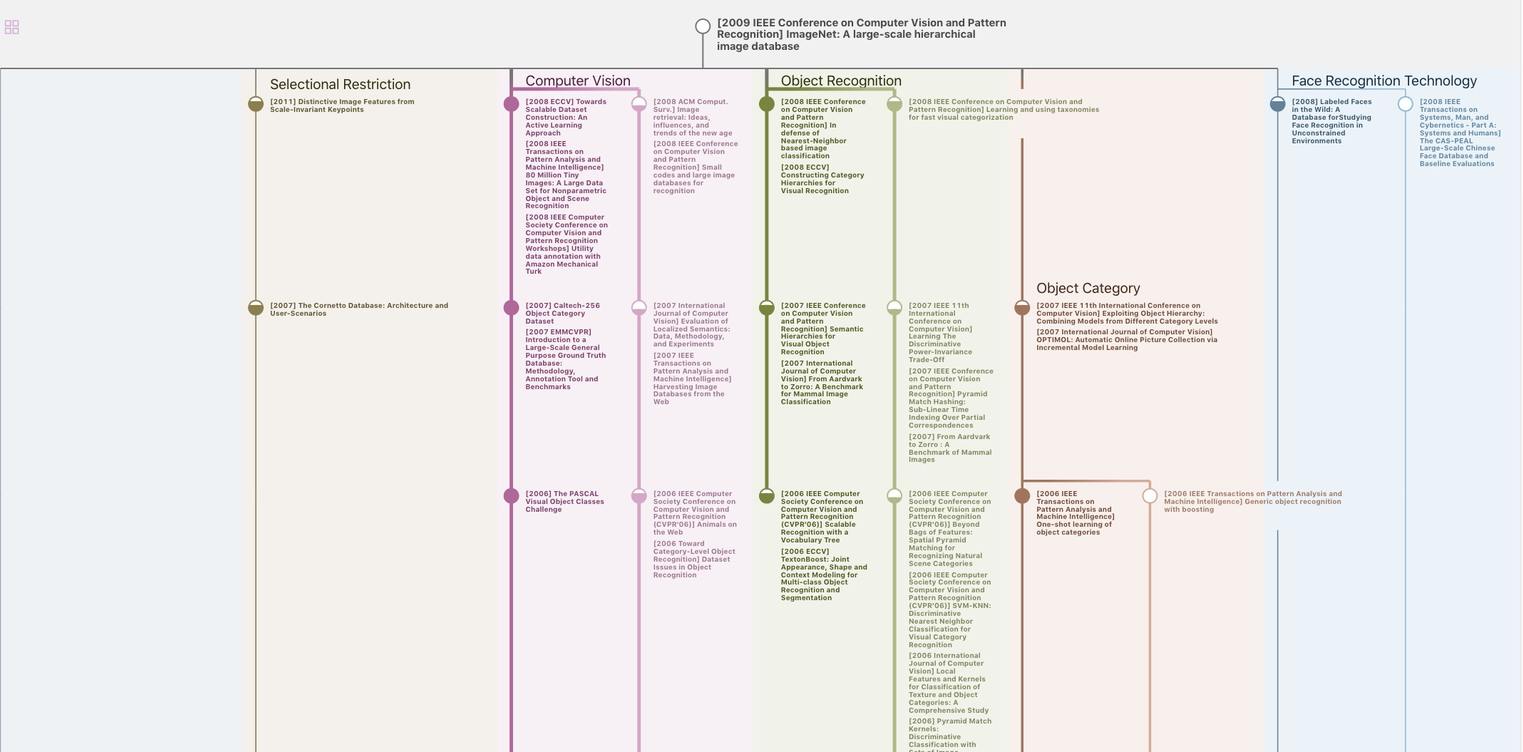
生成溯源树,研究论文发展脉络
Chat Paper
正在生成论文摘要