DeepRAT - A DRL-Based Framework for Multi-RAT Assignment and Power Allocation in HetNets.
ICC Workshops(2021)
摘要
Wireless heterogeneous networks (HetNets), where several systems with multi-radio access technologies (multi-RATs) coexist for massive multi-connectivity networks, are in service nowadays and are expected to be a main enabling technology in the future wireless networks. The extensive heterogeneity of such networks in terms of RATs architectures and their supported applications poses extra challenges on efficiently managing networks' communication resources, such as power and spectrum. In this paper, we propose a non-cooperative multi-agent deep reinforcement learning (DRL)-based framework, called DeepRAT, to address the problem of joint multi-RAT assignment and dynamic power allocation in the downlink of multi-connectivity edge devices (EDs) in future HetNets. In particular, the problem is formulated as a partially observable Markov decision process (POMDP), and the DeepRAT algorithm solves this computationally-expensive problem hierarchically by decomposing it into two stages; a multi-RAT assignment stage and a power allocation stage. The first stage utilizes the Deep Q-Network (DQN) algorithm to learn the optimal policy for RAT assignment of EDs. The second stage employs the Deep Deterministic Policy Gradient (DDPG) algorithm to solve the power allocation problem for RATs' assigned EDs. Simulation results show that the DeepRAT algorithm's performance is about 98.1% and 95.6% compared to the state-of-the-art methods that assume perfect information of the HetNet dynamics.
更多查看译文
关键词
DRL,DQN,DDPG,Resource Allocation,Multi-RAT,Heterogenous Networks
AI 理解论文
溯源树
样例
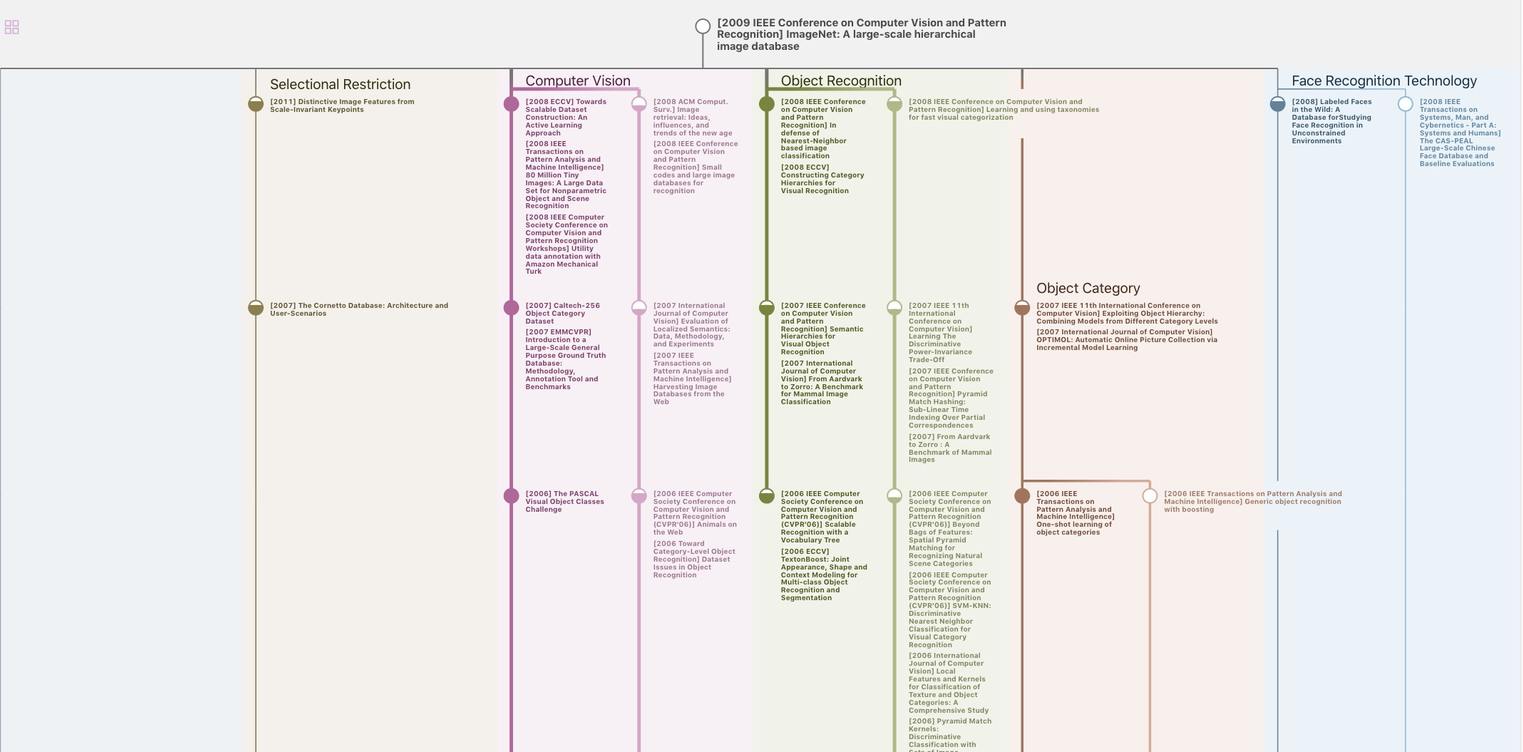
生成溯源树,研究论文发展脉络
Chat Paper
正在生成论文摘要