Fast Unsupervised Multi-Scale Characterization Of Urban Landscapes Based On Earth Observation Data
REMOTE SENSING(2021)
摘要
Most remote sensing studies of urban areas focus on a single scale, using supervised methodologies and very few analyses focus on the "neighborhood" scale. The lack of multi-scale analysis, together with the scarcity of training and validation datasets in many countries lead us to propose a single fast unsupervised method for the characterization of urban areas. With the FOTOTEX algorithm, this paper introduces a texture-based method to characterize urban areas at three nested scales: macro-scale (urban footprint), meso-scale ("neighbourhoods") and micro-scale (objects). FOTOTEX combines a Fast Fourier Transform and a Principal Component Analysis to convert texture into frequency signal. Several parameters were tested over Sentinel-2 and Pleiades imagery on Bouake and Brasilia. Results showed that a single Sentinel-2 image better assesses the urban footprint than the global products. Pleiades images allowed discriminating neighbourhoods and urban objects using texture, which is correlated with metrics such as building density, built-up and vegetation proportions. The best configurations for each scale of analysis were determined and recommendations provided to users. The open FOTOTEX algorithm demonstrated a strong potential to characterize the three nested scales of urban areas, especially when training and validation data are scarce, and computing resources limited.
更多查看译文
关键词
remote sensing, multi-scale, unsupervised, urban landscapes, texture
AI 理解论文
溯源树
样例
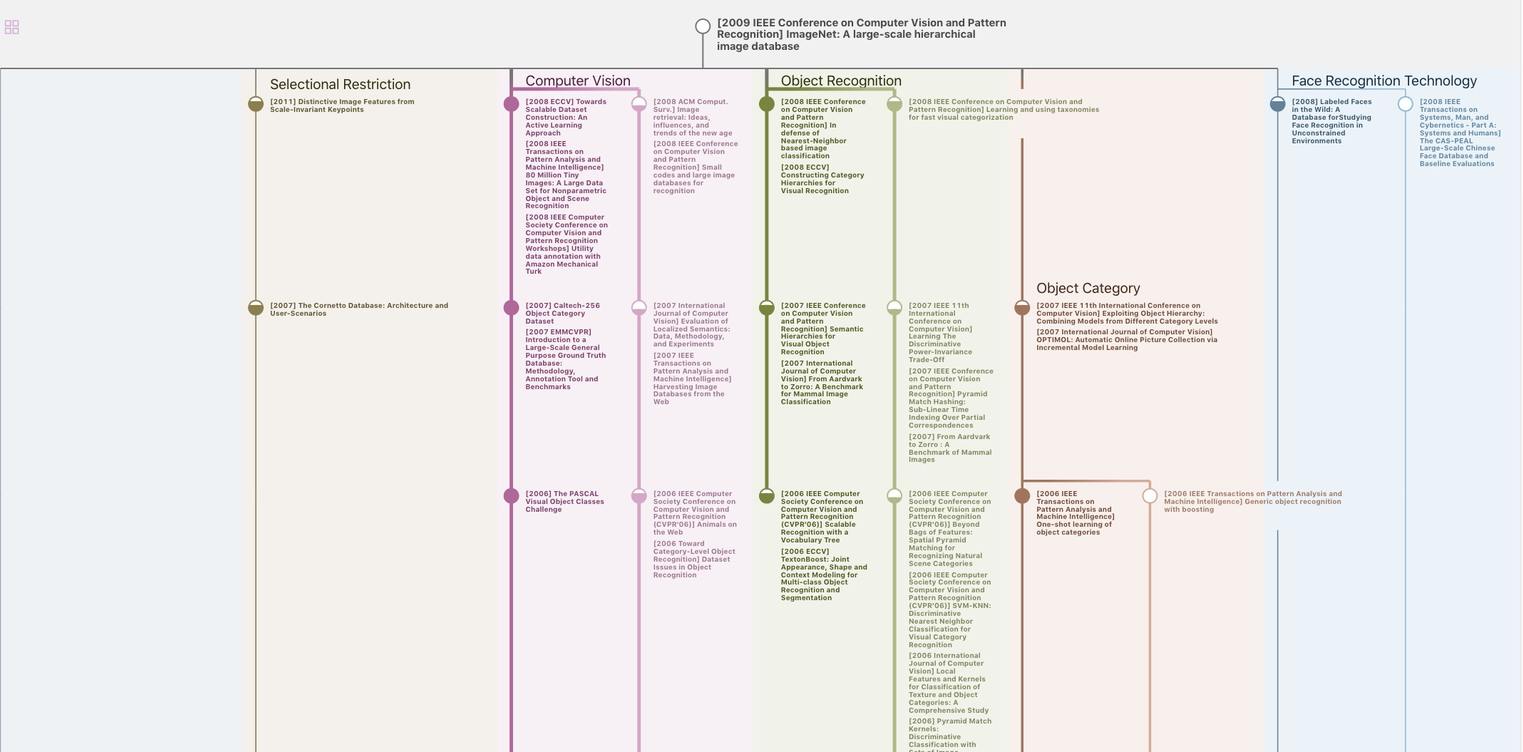
生成溯源树,研究论文发展脉络
Chat Paper
正在生成论文摘要