Satellite Flood Inundation Assessment And Forecast Using Smap And Landsat
IEEE JOURNAL OF SELECTED TOPICS IN APPLIED EARTH OBSERVATIONS AND REMOTE SENSING(2021)
摘要
The capability and synergistic use of multisource satellite observations for flood monitoring and forecasts is crucial for improving disaster preparedness and mitigation. Here, surface fractional water cover (FW) retrievals derived from Soil Moisture Active Passive (SMAP) L-band (1.4 GHz) brightness temperatures were used for flood assessment over southeast Africa during the Cyclone Idai event. We then focused on five subcatchments of the Pungwe basin and developed a machine learning based approach with the support of Google Earth Engine for daily (24-h) forecasting of FW and 30-m inundation downscaling and mapping. The Classification and Regression Trees model was selected and trained using retrievals derived from SMAP and Landsat coupled with rainfall forecasts from the NOAA Global Forecast System. Independent validation showed that FW predictions over randomly selected dates are highly correlated (R = 0.87) with the Landsat observations. The forecast results captured the flood temporal dynamics from the Idai event; and the associated 30-m downscaling results showed inundation spatial patterns consistent with independent satellite synthetic aperture radar observations. The data-driven approach provides new capacity for flood monitoring and forecasts leveraging synergistic satellite observations and big data analysis, which is particularly valuable for data sparse regions.
更多查看译文
关键词
Floods, Remote sensing, Satellites, Earth, Predictive models, Microwave theory and techniques, Artificial satellites, Flood, Global Forecast System (GFS), Google Earth Engine (GEE), Landsat, Soil Moisture Active Passive (SMAP)
AI 理解论文
溯源树
样例
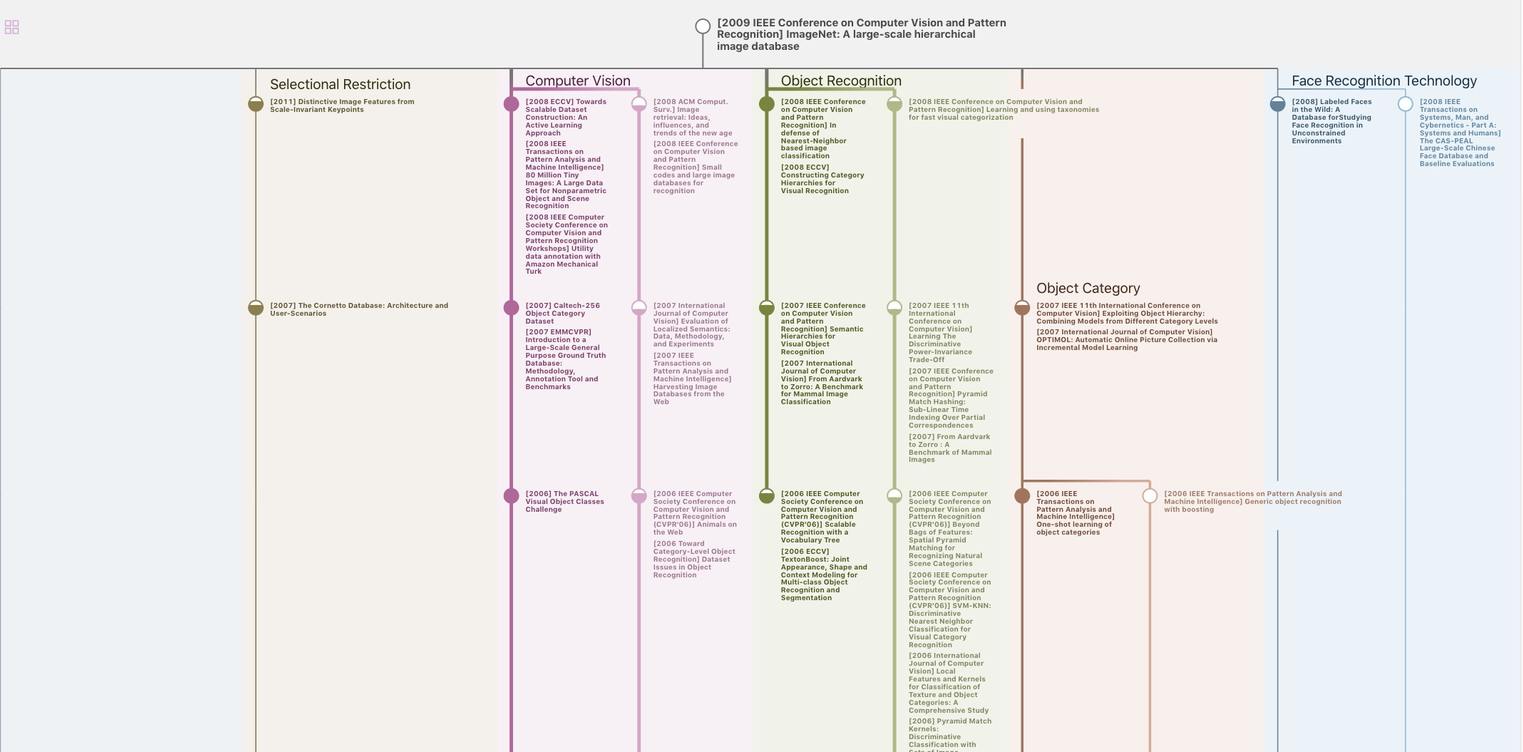
生成溯源树,研究论文发展脉络
Chat Paper
正在生成论文摘要