Using the learnings of machine learning to distill cloud controlling environmental regimes from satellite observations
user-5dd52aee530c701191bf1b99(2021)
摘要
<div> <div> <div> <div> <p>Cloud processes are the leading source of uncertainty in our current global climate models. Therefore understanding cloud formation, lifetime, and decay remains pivotal in order to reduce uncertainty in our global climate models future projections. Exploiting over ten years of satellite observations, the relationships between cloud properties and environmental factors, including aerosols, can be better understood and clustered into environmental regimes. We cluster regimes based on the regional strength of the relationships between the environment and cloud properties revealed using a random forest. Numerous processes, such as stratocumulus to cumulus transitions, may be constrained by the environmental regimes revealed by our analysis. Our results show that depending on the region, aerosol and the environment work to determine the baseline cloud properties. These observation based regimes can be compared to regimes derived from global climate models to understand how well model parameterizations capture the cloud controlling factors.</p> </div> </div> </div> </div>
更多查看译文
关键词
Cloud computing,Satellite,Remote sensing,Computer science
AI 理解论文
溯源树
样例
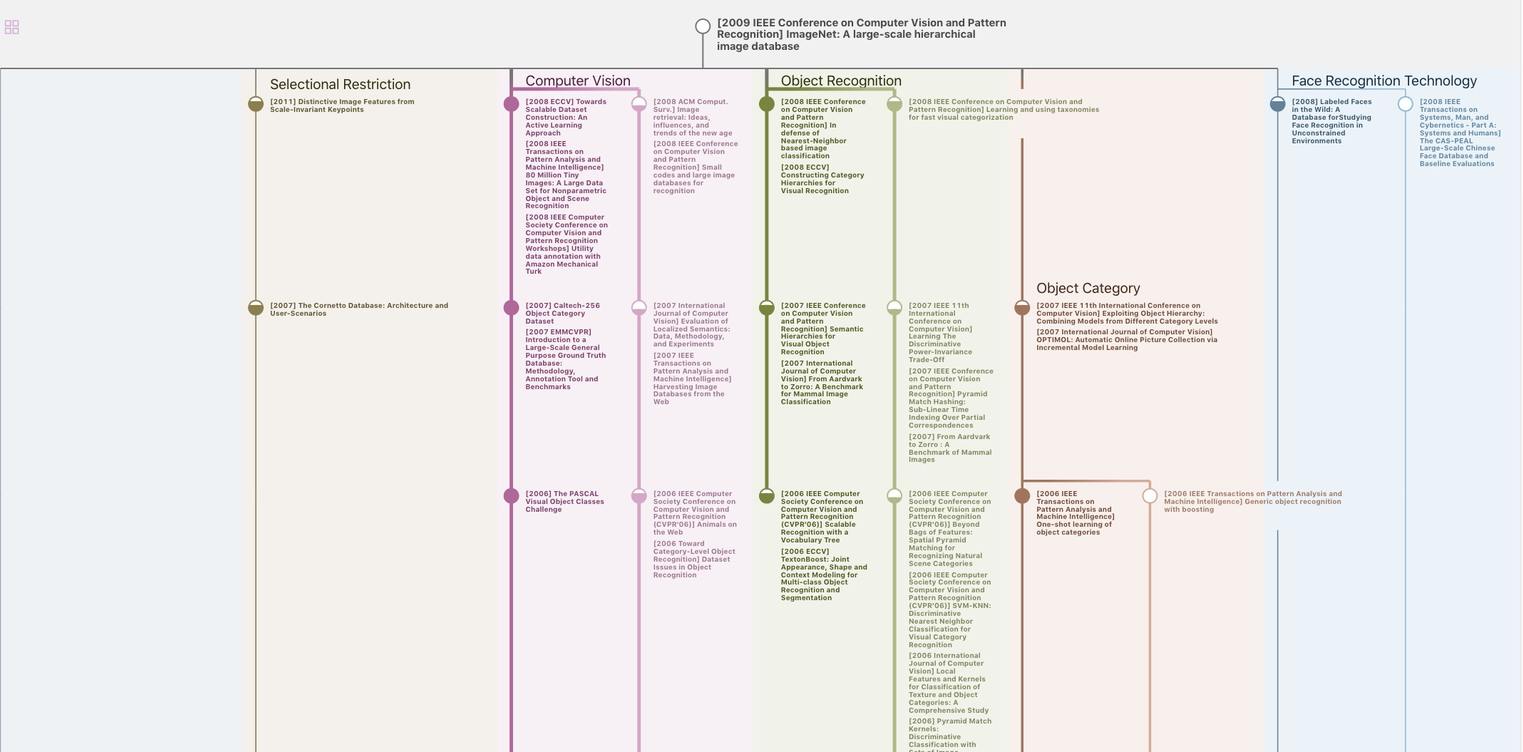
生成溯源树,研究论文发展脉络
Chat Paper
正在生成论文摘要