Investigating Particle Track Topology For Range Telescopes In Particle Radiography Using Convolutional Neural Networks
ACTA ONCOLOGICA(2021)
摘要
Background Proton computed tomography (pCT) and radiography (pRad) are proposed modalities for improved treatment plan accuracy and in situ treatment validation in proton therapy. The pCT system of the Bergen pCT collaboration is able to handle very high particle intensities by means of track reconstruction. However, incorrectly reconstructed and secondary tracks degrade the image quality. We have investigated whether a convolutional neural network (CNN)-based filter is able to improve the image quality. Material and methods The CNN was trained by simulation and reconstruction of tens of millions of proton and helium tracks. The CNN filter was then compared to simple energy loss threshold methods using the Area Under the Receiver Operating Characteristics curve (AUROC), and by comparing the image quality and Water Equivalent Path Length (WEPL) error of proton and helium radiographs filtered with the same methods. Results The CNN method led to a considerable improvement of the AUROC, from 74.3% to 97.5% with protons and from 94.2% to 99.5% with helium. The CNN filtering reduced the WEPL error in the helium radiograph from 1.03 mm to 0.93 mm while no improvement was seen in the CNN filtered pRads. Conclusion The CNN improved the filtering of proton and helium tracks. Only in the helium radiograph did this lead to improved image quality.
更多查看译文
关键词
Proton computed tomography, machine learning, Monte Carlo simulation, track reconstruction, convolutional neural network, secondary particles
AI 理解论文
溯源树
样例
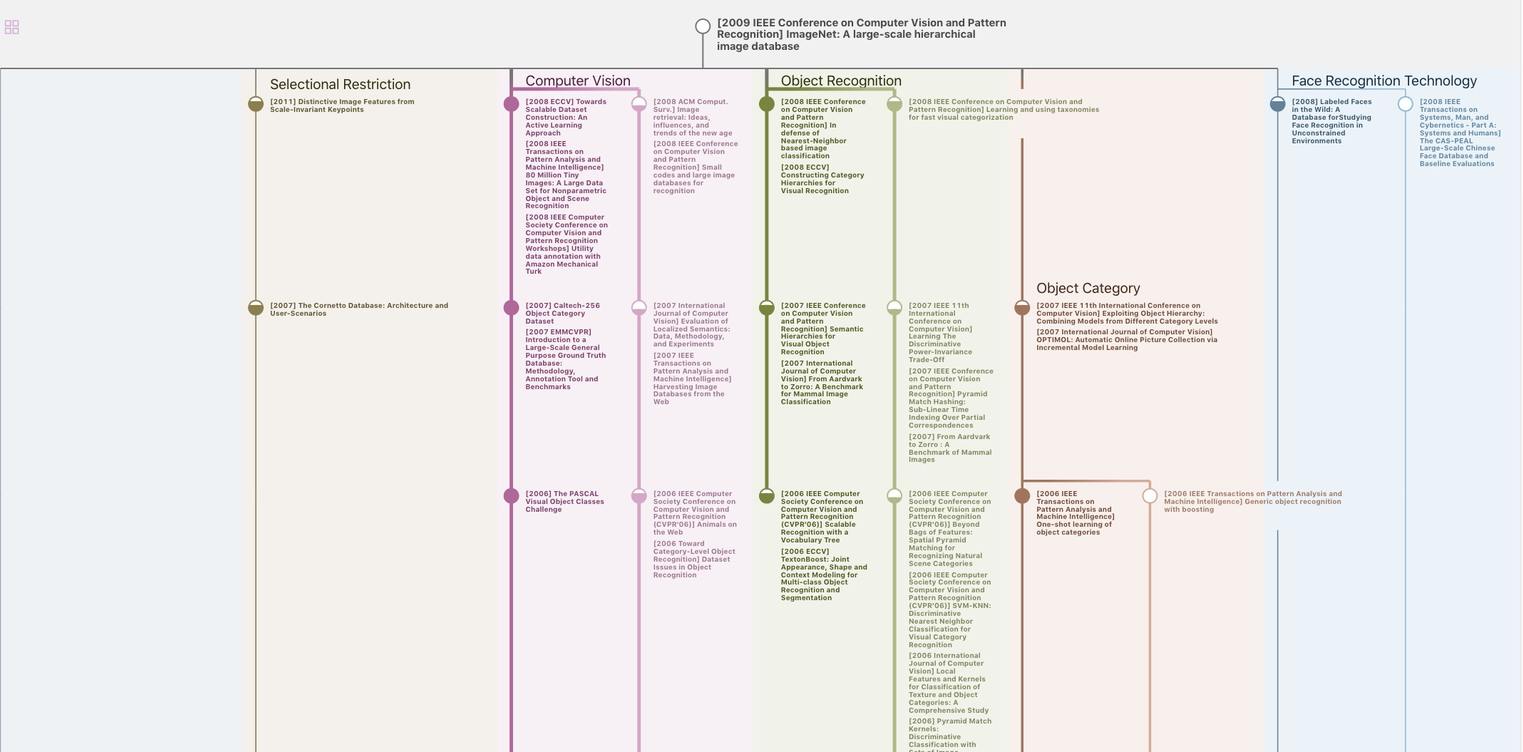
生成溯源树,研究论文发展脉络
Chat Paper
正在生成论文摘要