GEOMETRY AND GENERALIZATION: EIGENVALUES AS PREDICTORS OF WHERE A NETWORK WILL FAIL TO GENERALIZE
FOUNDATIONS OF DATA SCIENCE(2022)
摘要
We study the deformation of the input space by a trained autoenco der via the Jacobians of the trained weight matrices. In doing so, we prove bounds for the mean squared errors for points in the input space, under assumptions regarding the orthogonality of the eigenvectors. We also show that the trace and the product of the eigenvalues of the Jacobian matrices is a good predictor of the mean squared errors on test points. This is a dataset independent means of testing an autoencoder's ability to generalize on new input. Namely, no knowledge of the dataset on which the network was trained is needed, only the parameters of the trained model.
更多查看译文
关键词
Data representation theory, neural networks, differential geometry, eigenvalues of local Jacobians
AI 理解论文
溯源树
样例
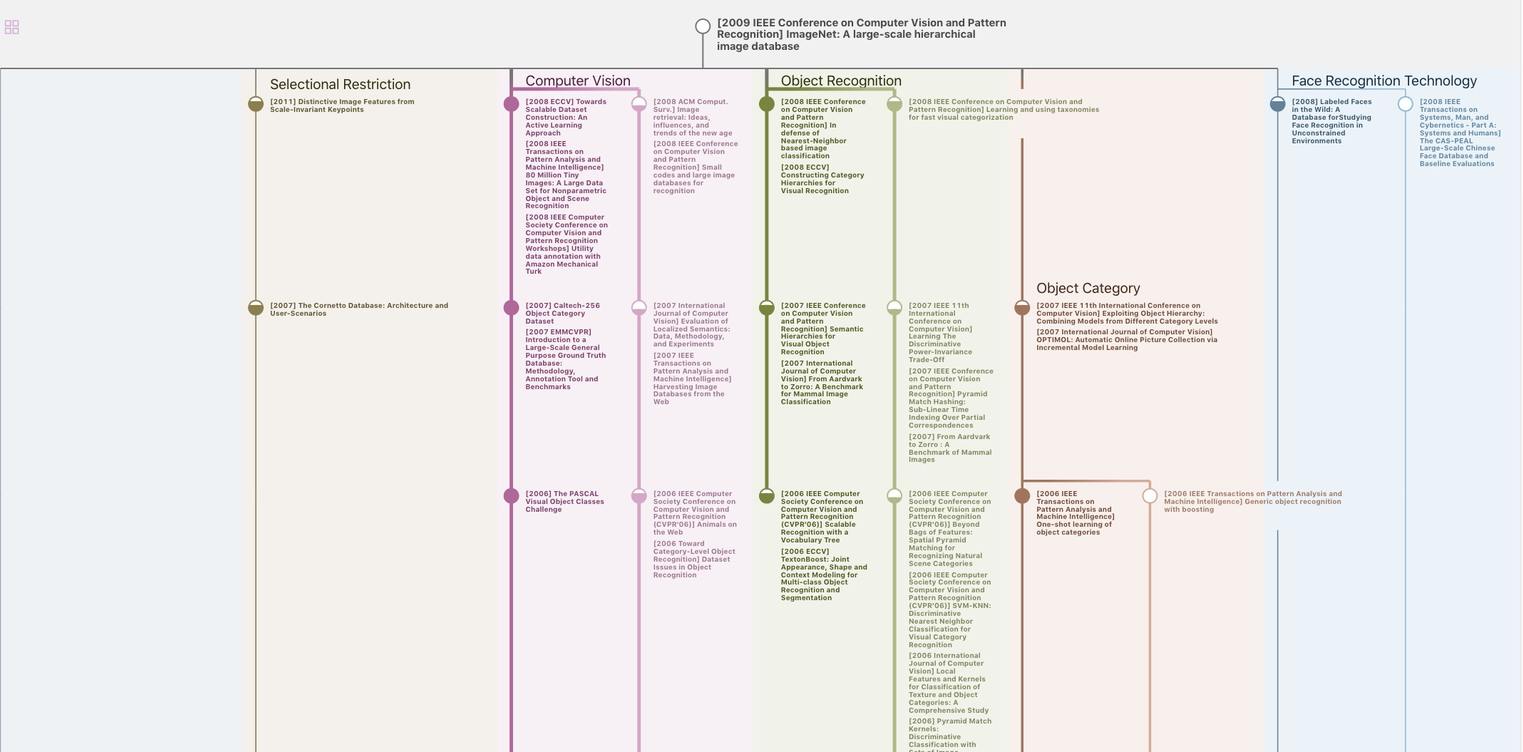
生成溯源树,研究论文发展脉络
Chat Paper
正在生成论文摘要