Learning a Discriminant Latent Space with Neural Discriminant Analysis
arxiv(2021)
摘要
Discriminative features play an important role in image and object classification and also in other fields of research such as semi-supervised learning, fine-grained classification, out of distribution detection. Inspired by Linear Discriminant Analysis (LDA), we propose an optimization called Neural Discriminant Analysis (NDA) for Deep Convolutional Neural Networks (DCNNs). NDA transforms deep features to become more discriminative and, therefore, improves the performances in various tasks. Our proposed optimization has two primary goals for inter- and intra-class variances. The first one is to minimize variances within each individual class. The second goal is to maximize pairwise distances between features coming from different classes. We evaluate our NDA optimization in different research fields: general supervised classification, fine-grained classification, semi-supervised learning, and out of distribution detection. We achieve performance improvements in all the fields compared to baseline methods that do not use NDA. Besides, using NDA, we also surpass the state of the art on the four tasks on various testing datasets.
更多查看译文
关键词
discriminant latent space,neural discriminant analysis,learning
AI 理解论文
溯源树
样例
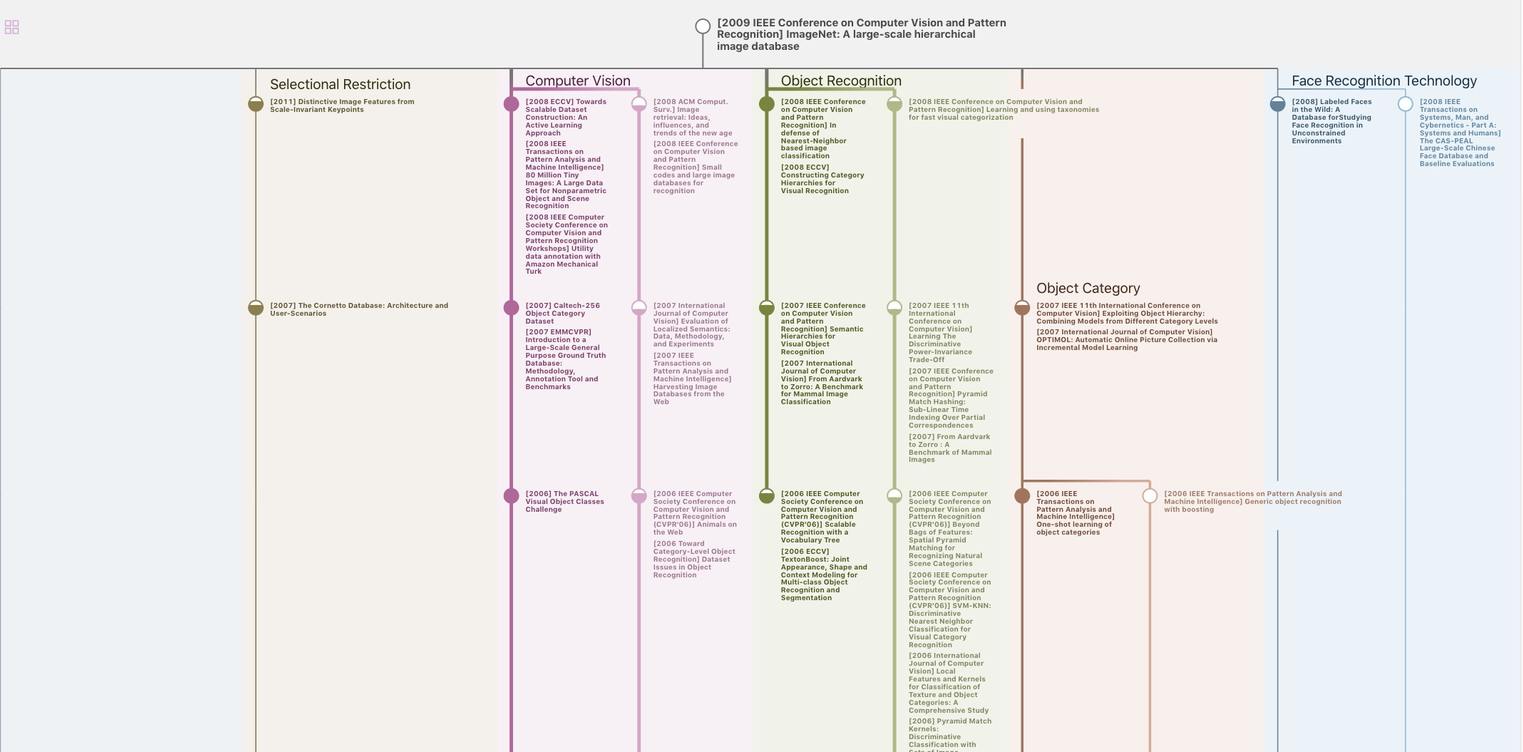
生成溯源树,研究论文发展脉络
Chat Paper
正在生成论文摘要