Correlation Analysis between the Robustness of Sparse Neural Networks and their Random Hidden Structural Priors
KNOWLEDGE-BASED AND INTELLIGENT INFORMATION & ENGINEERING SYSTEMS (KSE 2021)(2021)
摘要
Deep learning models have been shown to be vulnerable to adversarial attacks. This perception led to analyzing deep learning models not only from the perspective of their performance measures but also their robustness to certain types of adversarial attacks. We take another step forward in relating the architectural structure of neural networks from a graph theoretic perspective to their robustness. We aim to investigate any existing correlations between graph theoretic properties and the robustness of Sparse Neural Networks. Our hypothesis is, that graph theoretic properties as a prior of neural network structures are related to their robustness. To answer to this hypothesis, we designed an empirical study with neural network models obtained through random graphs used as sparse structural priors for the networks. We additionally investigated the evaluation of a randomly pruned fully connected network as a point of reference. We found that robustness measures are independent of initialization methods but show weak correlations with graph properties: higher graph densities correlate with lower robustness, but higher average path lengths and average node eccentricities show negative correlations with robustness measures. We hope to motivate further empirical and analytical research to tightening an answer to our hypothesis. (C) 2021 The Authors. Published by Elsevier B.V. This is an open access article under the CC BY-NC-ND license (https://creativecommons.org/licenses/by-nc-nd/4.0) Peer-review under responsibility of the scientific committee of KES International.
更多查看译文
关键词
Robustness, Adversarial Attacks, Sparse Neural Networks
AI 理解论文
溯源树
样例
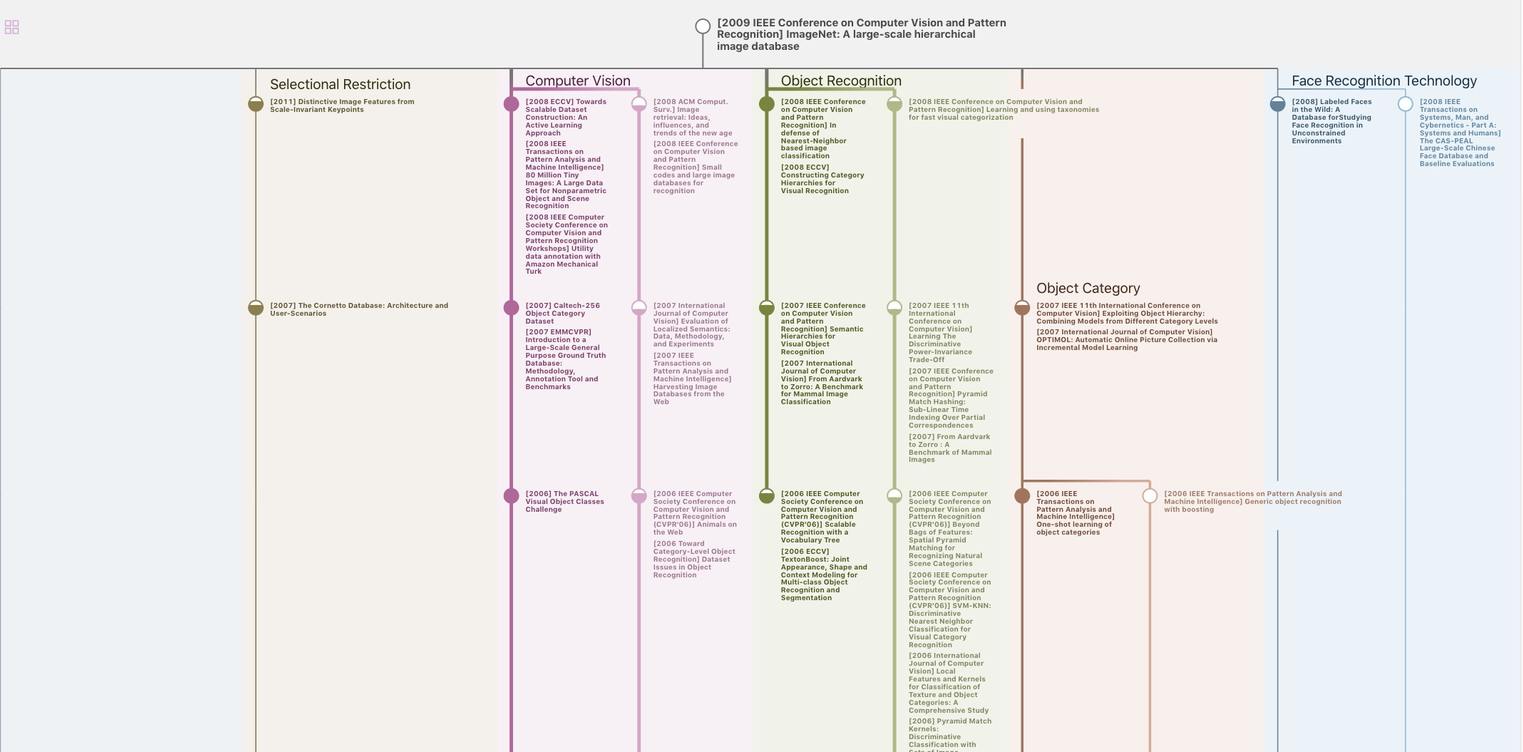
生成溯源树,研究论文发展脉络
Chat Paper
正在生成论文摘要