Hyperparameter Optimization: Foundations, Algorithms, Best Practices and Open Challenges
arxiv(2021)
摘要
Most machine learning algorithms are configured by one or several hyperparameters that must be carefully chosen and often considerably impact performance. To avoid a time consuming and unreproducible manual trial-and-error process to find well-performing hyperparameter configurations, various automatic hyperparameter optimization (HPO) methods, e.g., based on resampling error estimation for supervised machine learning, can be employed. After introducing HPO from a general perspective, this paper reviews important HPO methods such as grid or random search, evolutionary algorithms, Bayesian optimization, Hyperband and racing. It gives practical recommendations regarding important choices to be made when conducting HPO, including the HPO algorithms themselves, performance evaluation, how to combine HPO with ML pipelines, runtime improvements, and parallelization.
更多查看译文
关键词
automl,hyperparameter optimization,machine learning,model selection,tuning
AI 理解论文
溯源树
样例
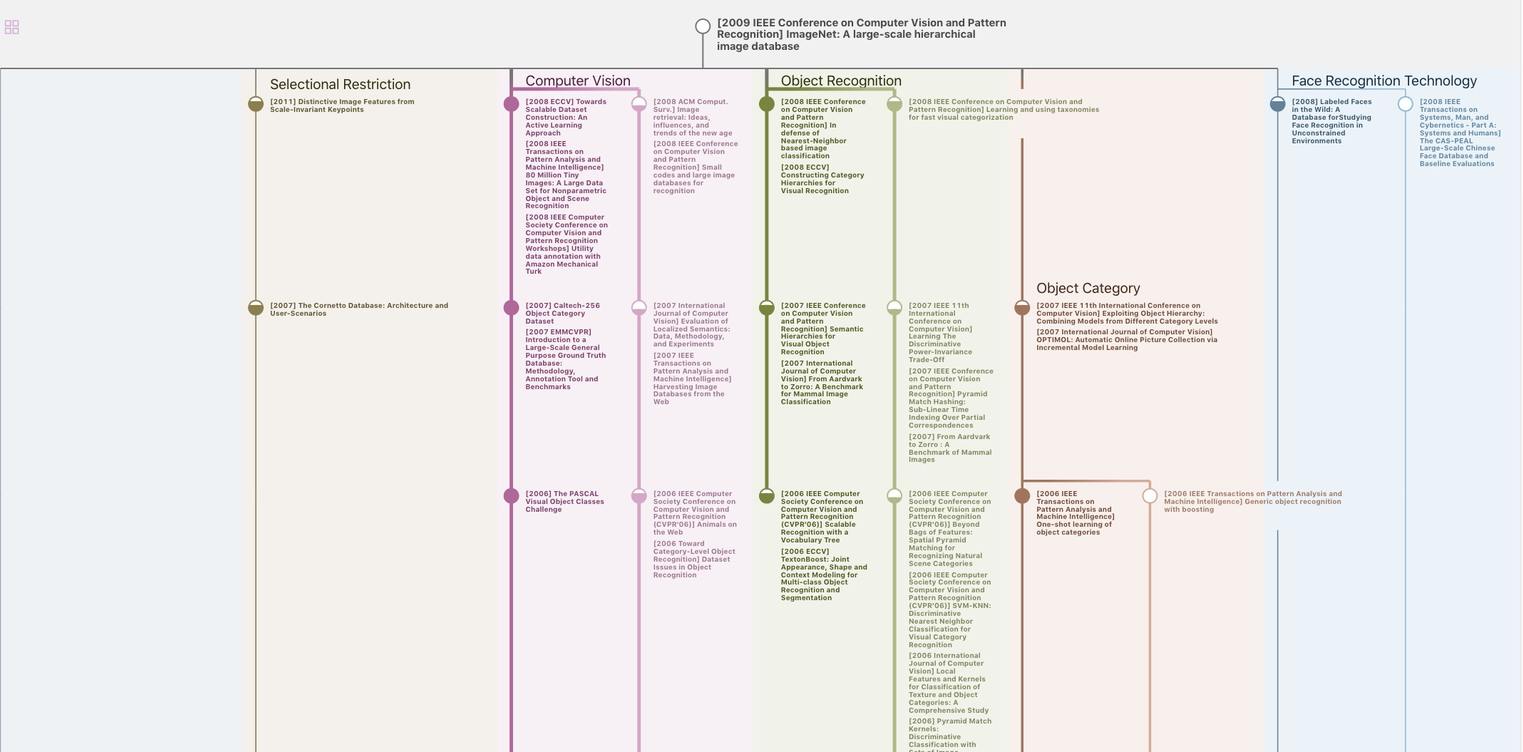
生成溯源树,研究论文发展脉络
Chat Paper
正在生成论文摘要