Coded Privacy-Preserving Computation at Edge Networks
arxiv(2021)
摘要
Multi-party computation (MPC) is promising for privacy-preserving machine learning algorithms at edge networks, like federated learning. Despite their potential, existing MPC algorithms fail short of adapting to the limited resources of edge devices. A promising solution, and the focus of this work, is coded computation, which advocates the use of error-correcting codes to improve the performance of distributed computing through "smart" data redundancy. In this paper, we focus on coded privacy-preserving computation using Shamir's secret sharing. In particular, we design novel coded privacy-preserving computation mechanisms; MatDot coded MPC (MatDot-CMPC) and PolyDot coded MPC (PolyDot-CMPC) by employing recently proposed coded computation algorithms; MatDot and PolyDot. We take advantage of the "garbage terms" that naturally arise when polynomials are constructed in the design of MatDot-CMPC and PolyDot-CMPC to reduce the number of workers needed for privacy-preserving computation. Also, we analyze MatDot-CMPC and PolyDot-CMPC in terms of their computation, storage, communication overhead as well as recovery threshold, so they can easily adapt to the limited resources of edge devices.
更多查看译文
关键词
efficient matrix multiplication,novel CMPC algorithm,PolyDot-CMPC,PolyDot codes,privacy-preserving computation,entangled polynomial codes,coded computation setup,MPC setting,PolyDot coded privacy preserving multiparty computation,edge networks,coded multiparty computation,emerging approach,CMPC approaches,computation algorithms
AI 理解论文
溯源树
样例
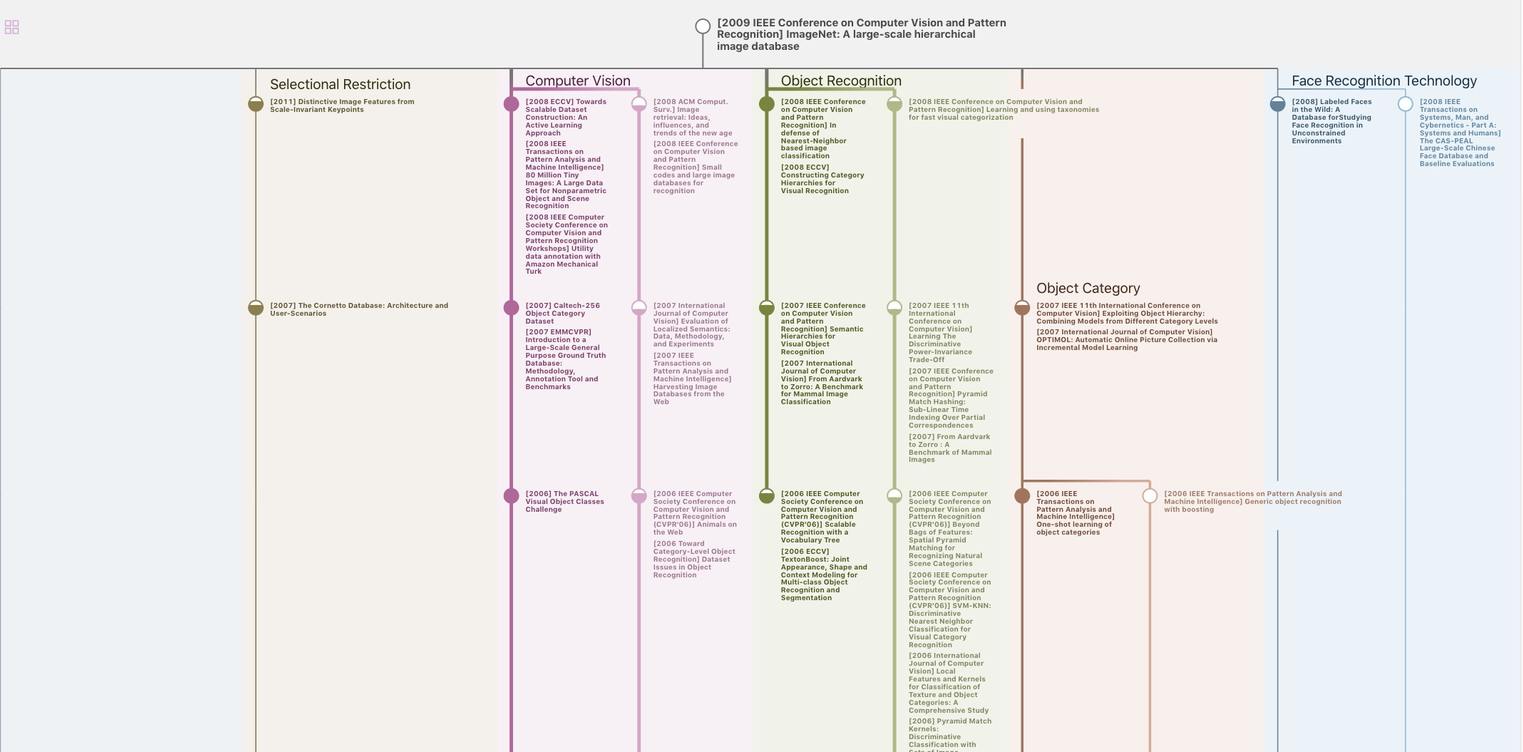
生成溯源树,研究论文发展脉络
Chat Paper
正在生成论文摘要