Polynomial Time Reinforcement Learning in Correlated FMDPs with Linear Value Functions
arxiv(2021)
摘要
Many reinforcement learning (RL) environments in practice feature enormous state spaces that may be described compactly by a "factored" structure, that may be modeled by Factored Markov Decision Processes (FMDPs). We present the first polynomial-time algorithm for RL with FMDPs that does not rely on an oracle planner, and instead of requiring a linear transition model, only requires a linear value function with a suitable local basis with respect to the factorization. With this assumption, we can solve FMDPs in polynomial time by constructing an efficient separation oracle for convex optimization. Importantly, and in contrast to prior work, we do not assume that the transitions on various factors are independent.
更多查看译文
关键词
correlated fmdps,reinforcement learning,linear value functions
AI 理解论文
溯源树
样例
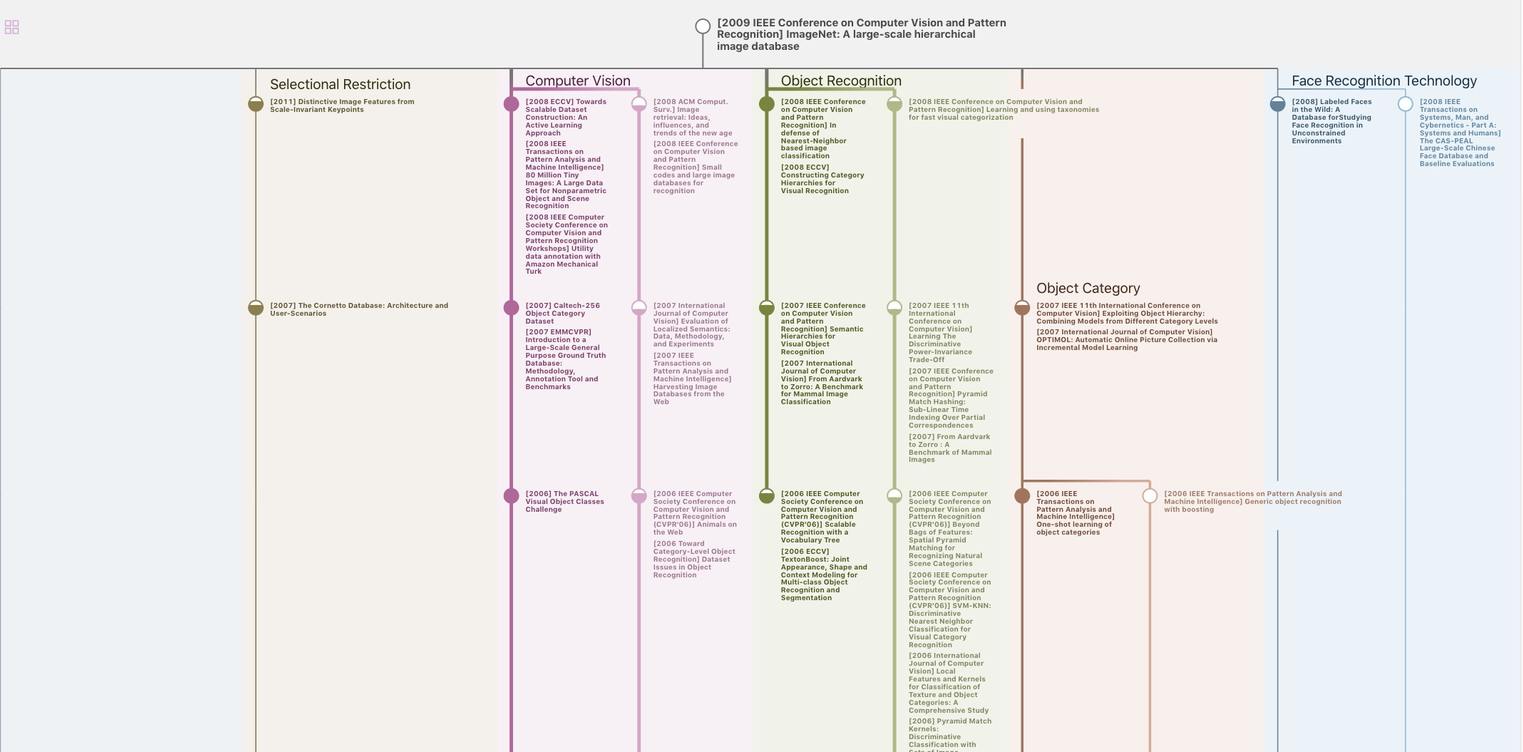
生成溯源树,研究论文发展脉络
Chat Paper
正在生成论文摘要