Out-of-Distribution Dynamics Detection: RL-Relevant Benchmarks and Results
arxiv(2021)
摘要
We study the problem of out-of-distribution dynamics (OODD) detection, which involves detecting when the dynamics of a temporal process change compared to the training-distribution dynamics. This is relevant to applications in control, reinforcement learning (RL), and multi-variate time-series, where changes to test time dynamics can impact the performance of learning controllers/predictors in unknown ways. This problem is particularly important in the context of deep RL, where learned controllers often overfit to the training environment. Currently, however, there is a lack of established OODD benchmarks for the types of environments commonly used in RL research. Our first contribution is to design a set of OODD benchmarks derived from common RL environments with varying types and intensities of OODD. Our second contribution is to design a strong OODD baseline approach based on recurrent implicit quantile networks (RIQNs), which monitors autoregressive prediction errors for OODD detection. Our final contribution is to evaluate the RIQN approach on the benchmarks to provide baseline results for future comparison.
更多查看译文
关键词
dynamics,detection,out-of-distribution,rl-relevant
AI 理解论文
溯源树
样例
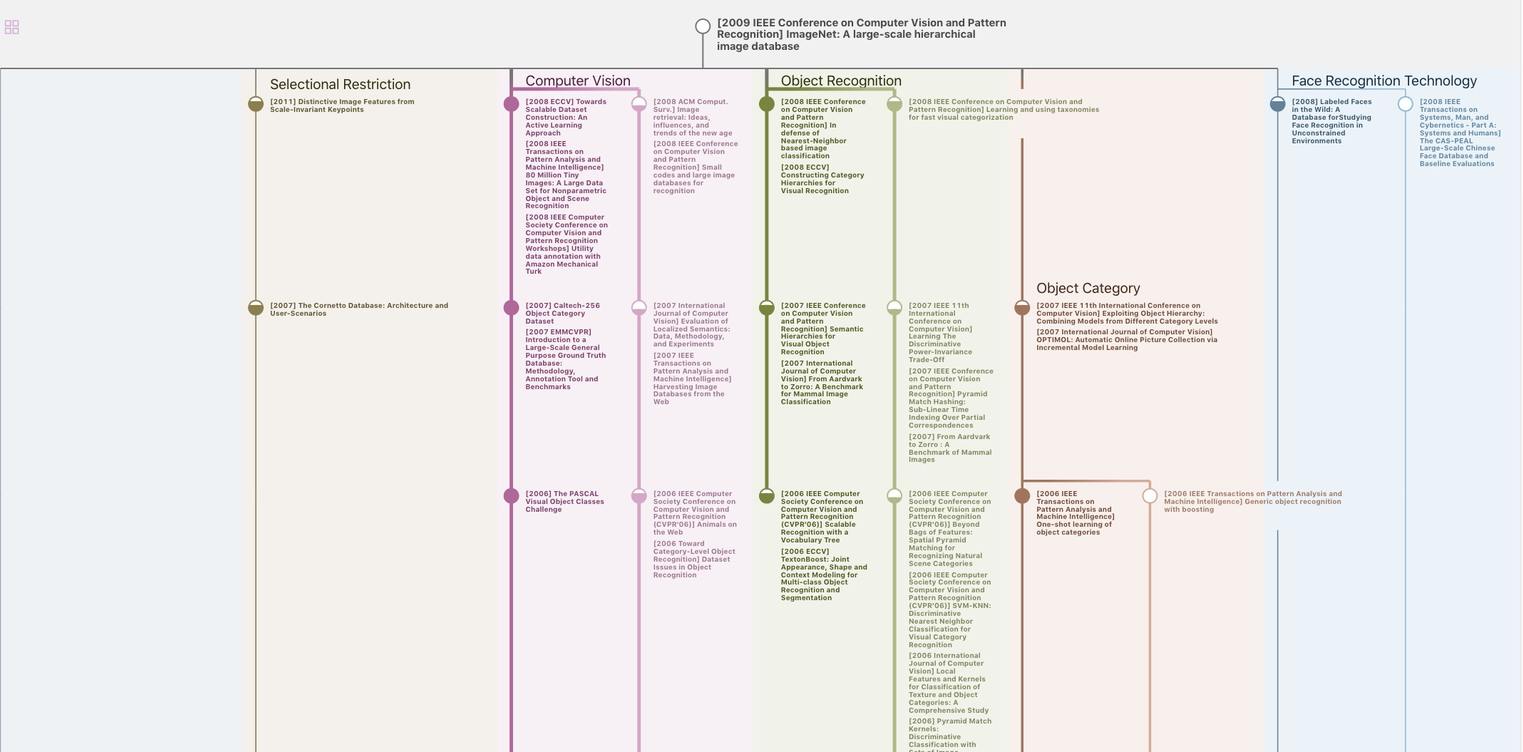
生成溯源树,研究论文发展脉络
Chat Paper
正在生成论文摘要