Spatiotemporal Gene Expression Profiling and Network Inference: A Roadmap for Analysis, Visualization, and Key Gene Identification.
MODELING TRANSCRIPTIONAL REGULATION: Methods and Protocols(2021)
摘要
Gene expression data analysis and the prediction of causal relationships within gene regulatory networks (GRNs) have guided the identification of key regulatory factors and unraveled the dynamic properties of biological systems. However, drawing accurate and unbiased conclusions requires a comprehensive understanding of relevant tools, computational methods, and their workflows. The topics covered in this chapter encompass the entire workflow for GRN inference including: (1) experimental design; (2) RNA sequencing data processing; (3) differentially expressed gene (DEG) selection; (4) clustering prior to inference; (5) network inference techniques; and (6) network visualization and analysis. Moreover, this chapter aims to present a workflow feasible and accessible for plant biologists without a bioinformatics or computer science background. To address this need, TuxNet, a user-friendly graphical user interface that integrates RNA sequencing data analysis with GRN inference, is chosen for the purpose of providing a detailed tutorial.
更多查看译文
关键词
Bioinformatics,Gene regulatory network inference,Network visualization,RNA sequencing
AI 理解论文
溯源树
样例
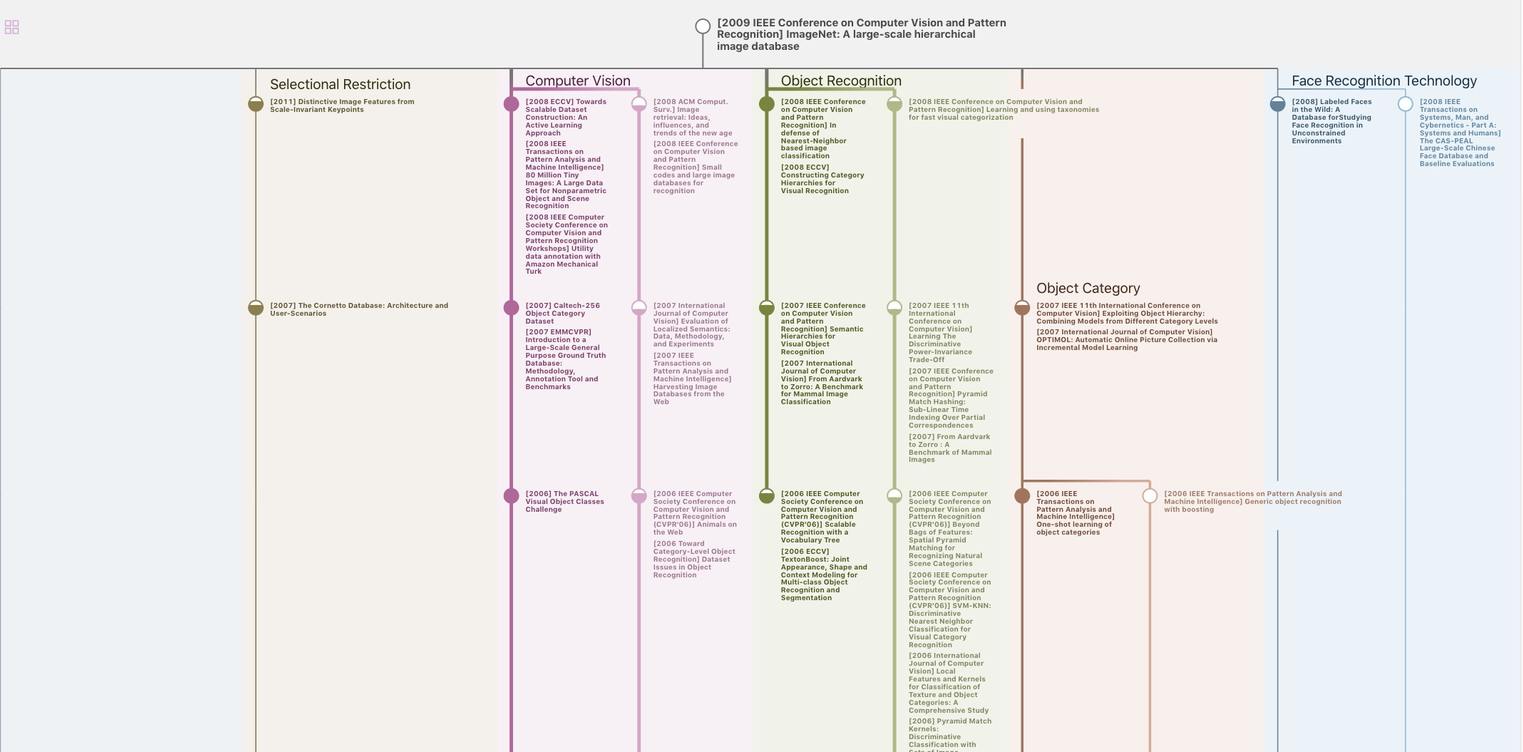
生成溯源树,研究论文发展脉络
Chat Paper
正在生成论文摘要