INTRODUCING DEEP REINFORCEMENT LEARNING TO NLU RANKING TASKS
2021 IEEE INTERNATIONAL CONFERENCE ON ACOUSTICS, SPEECH AND SIGNAL PROCESSING (ICASSP 2021)(2021)
Abstract
Natural language understanding (NLU) models in production systems rely heavily on human annotated data, which involves an expensive, time-consuming, and error-prone process. Moreover, the model release process requires offline supervised learning and human in-the-loop. Together, these factors prolong the model update cycle and result in sub-standard model performance in situations where usage behavior is non-stationary. In this paper, we address these issues with a deep reinforcement learning approach that ranks suggestions from multiple experts in an online fashion. Our proposed method removes the reliance on annotated data, and can effectively adapt to recent changes in the data distribution. The efficiency of the new approach is demonstrated through simulation experiments using logged data from voice-based virtual assistants. Our results show that our algorithm, without any reliance on annotation, outperforms offline supervised learning methods.
MoreTranslated text
Key words
ranking, natural language understanding, reinforcement learning
AI Read Science
Must-Reading Tree
Example
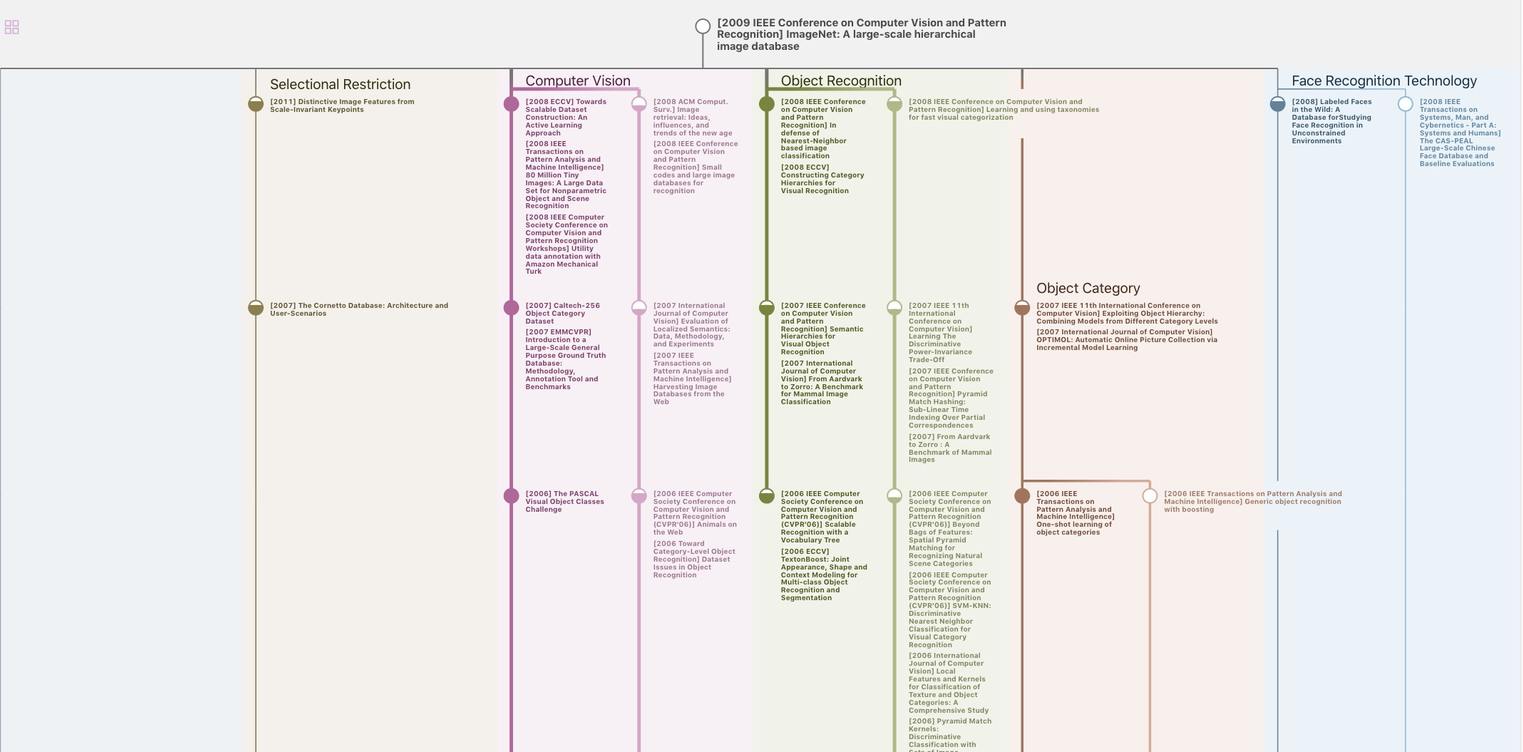
Generate MRT to find the research sequence of this paper
Chat Paper
Summary is being generated by the instructions you defined