COST AFFINITY LEARNING NETWORK FOR STEREO MATCHING
2021 IEEE INTERNATIONAL CONFERENCE ON ACOUSTICS, SPEECH AND SIGNAL PROCESSING (ICASSP 2021)(2021)
Abstract
Existing stereo matching methods mainly tend to directly aggregate features output from Convolutional Neural Network to obtain more discriminative cost features, but ignore the affinity of each element in the cost feature which also plays a key role in enhancing the cost feature. In this work, we propose a novel cost affinity learning network(CAL-Net) whose Affinity Enhanced Module(AEM) extracts the affinity of the elements in the cost feature and reconstructs a more discriminative feature. In addition, CAL-Net designs a Disparity Weight Loss(DWL) to guide training. Specifically, AEM takes the advatange of the self-attention mechanism to learn internal affinity between different elements and exploits it to reconstruct the cost feature for emphasizing informative elements. DWL calculates the adaptive weight according to disparity error. As the error decreases, the weight gradually increases and enables the network to gradually transit from the pixel level disparity to sub-pixel level. Experiments demonstrate that CAL-Net boosts the performance, especially in textureless and reflective regions, and achieves better results on Scene Flow and KITTI 2012 benchmarks than some typical related methods.
MoreTranslated text
Key words
Deep Learning, Stereo Matching, Attention Mechanism, Weighted Loss Function
AI Read Science
Must-Reading Tree
Example
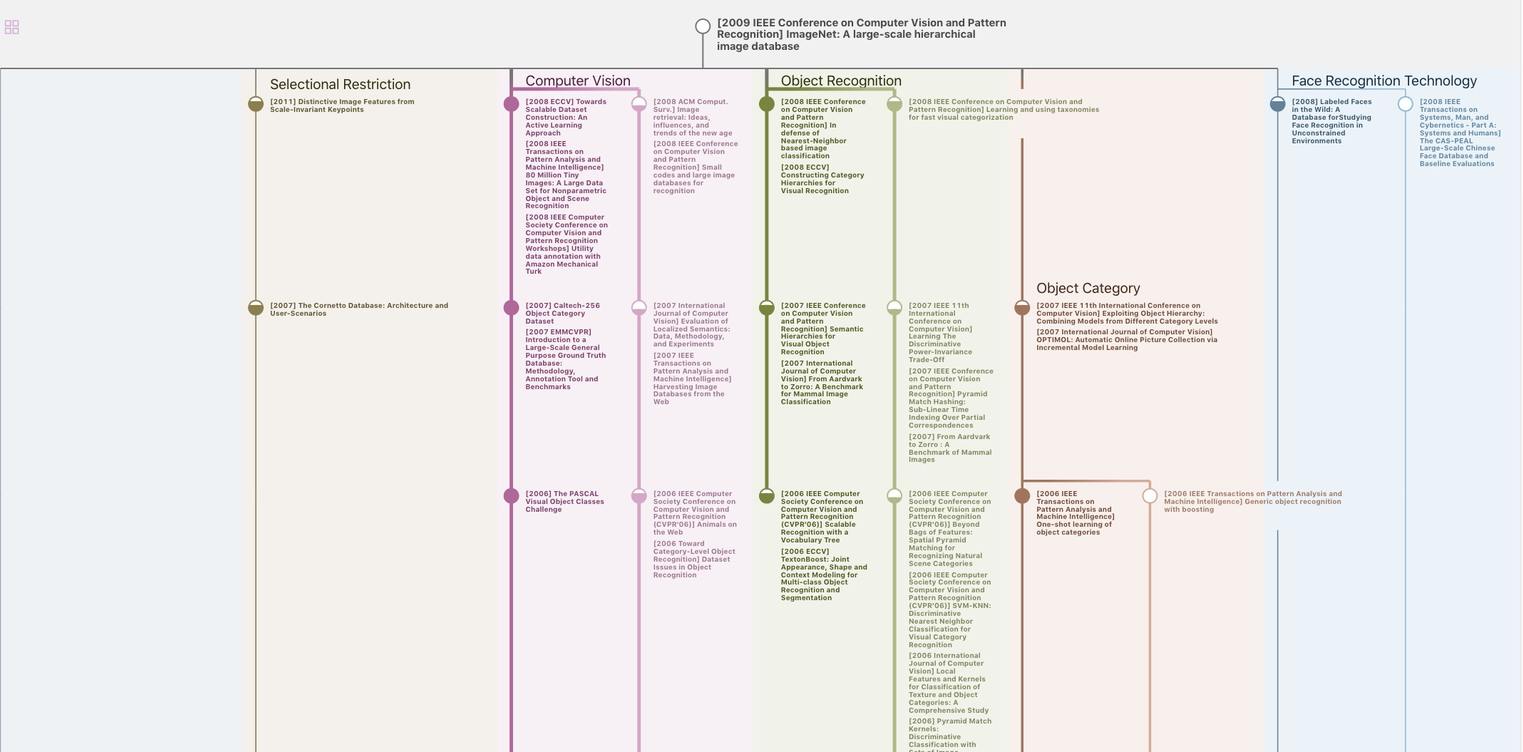
Generate MRT to find the research sequence of this paper
Chat Paper
Summary is being generated by the instructions you defined