Cartesian Message Passing Neural Networks For Directional Properties: Fast And Transferable Atomic Multipoles
JOURNAL OF CHEMICAL PHYSICS(2021)
摘要
The message passing neural network (MPNN) framework is a promising tool for modeling atomic properties but is, until recently, incompatible with directional properties, such as Cartesian tensors. We propose a modified Cartesian MPNN (CMPNN) suitable for predicting atom-centered multipoles, an essential component of ab initio force fields. The efficacy of this model is demonstrated on a newly developed dataset consisting of 46 623 chemical structures and corresponding high-quality atomic multipoles, which was deposited into the publicly available Molecular Sciences Software Institute QCArchive server. We show that the CMPNN accurately predicts atom-centered charges, dipoles, and quadrupoles and that errors in the predicted atomic multipoles have a negligible effect on multipole-multipole electrostatic energies. The CMPNN is accurate enough to model conformational dependencies of a molecule's electronic structure. This opens up the possibility of recomputing atomic multipoles on the fly throughout a simulation in which they might exhibit strong conformational dependence. Published under an exclusive license by AIP Publishing.
更多查看译文
关键词
transferable atomic multipoles,neural networks,cartesian message,directional properties
AI 理解论文
溯源树
样例
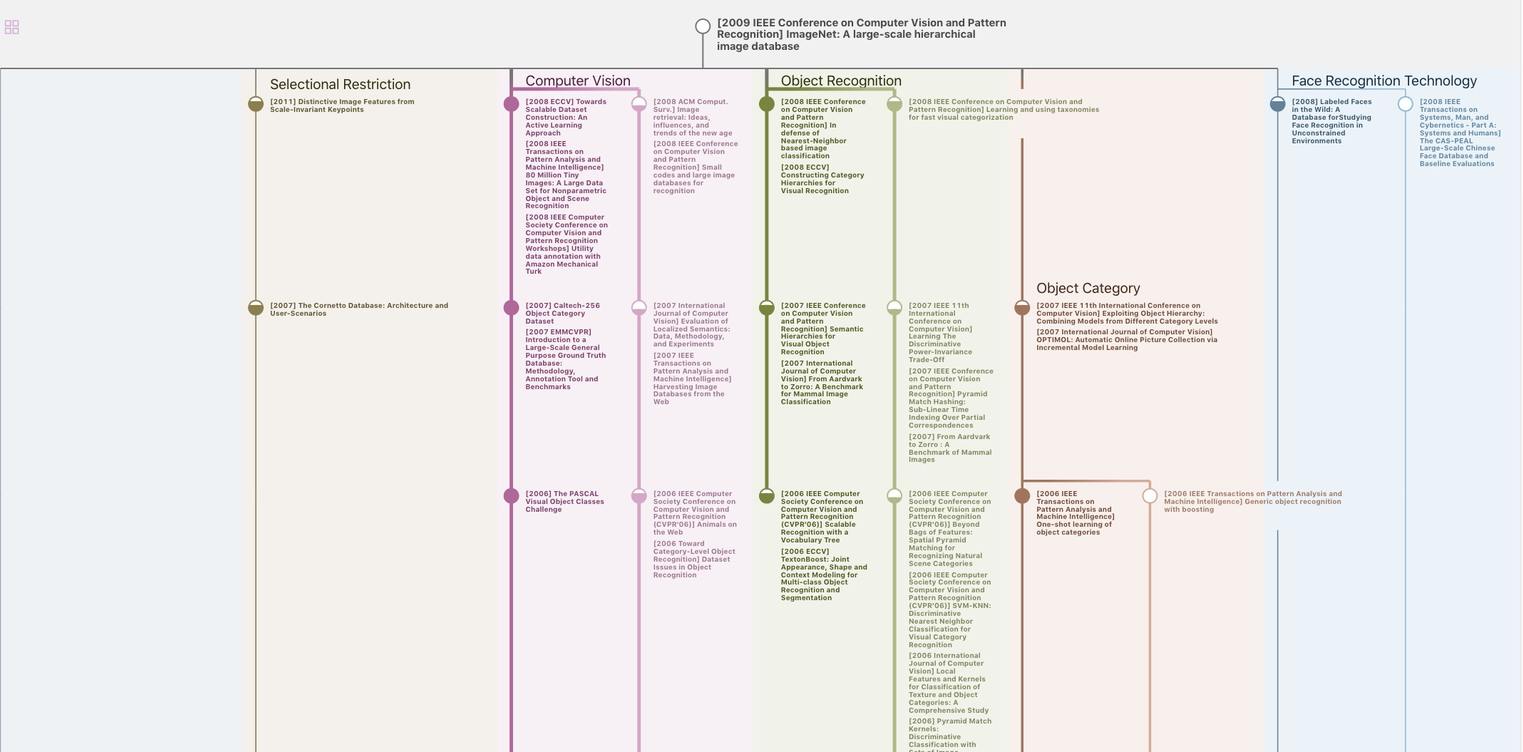
生成溯源树,研究论文发展脉络
Chat Paper
正在生成论文摘要