Machine Learning Using Host/Guest Energy Histograms To Predict Adsorption In Metal-Organic Frameworks: Application To Short Alkanes And Xe/Kr Mixtures
JOURNAL OF CHEMICAL PHYSICS(2021)
摘要
A machine learning (ML) methodology that uses a histogram of interaction energies has been applied to predict gas adsorption in metal-organic frameworks (MOFs) using results from atomistic grand canonical Monte Carlo (GCMC) simulations as training and test data. In this work, the method is first extended to binary mixtures of spherical species, in particular, Xe and Kr. In addition, it is shown that single-component adsorption of ethane and propane can be predicted in good agreement with GCMC simulation using a histogram of the adsorption energies felt by a methyl probe in conjunction with the random forest ML method. The results for propane can be improved by including a small number of MOF textural properties as descriptors. We also discuss the most significant features, which provides physical insight into the most beneficial adsorption energy sites for a given application.
更多查看译文
AI 理解论文
溯源树
样例
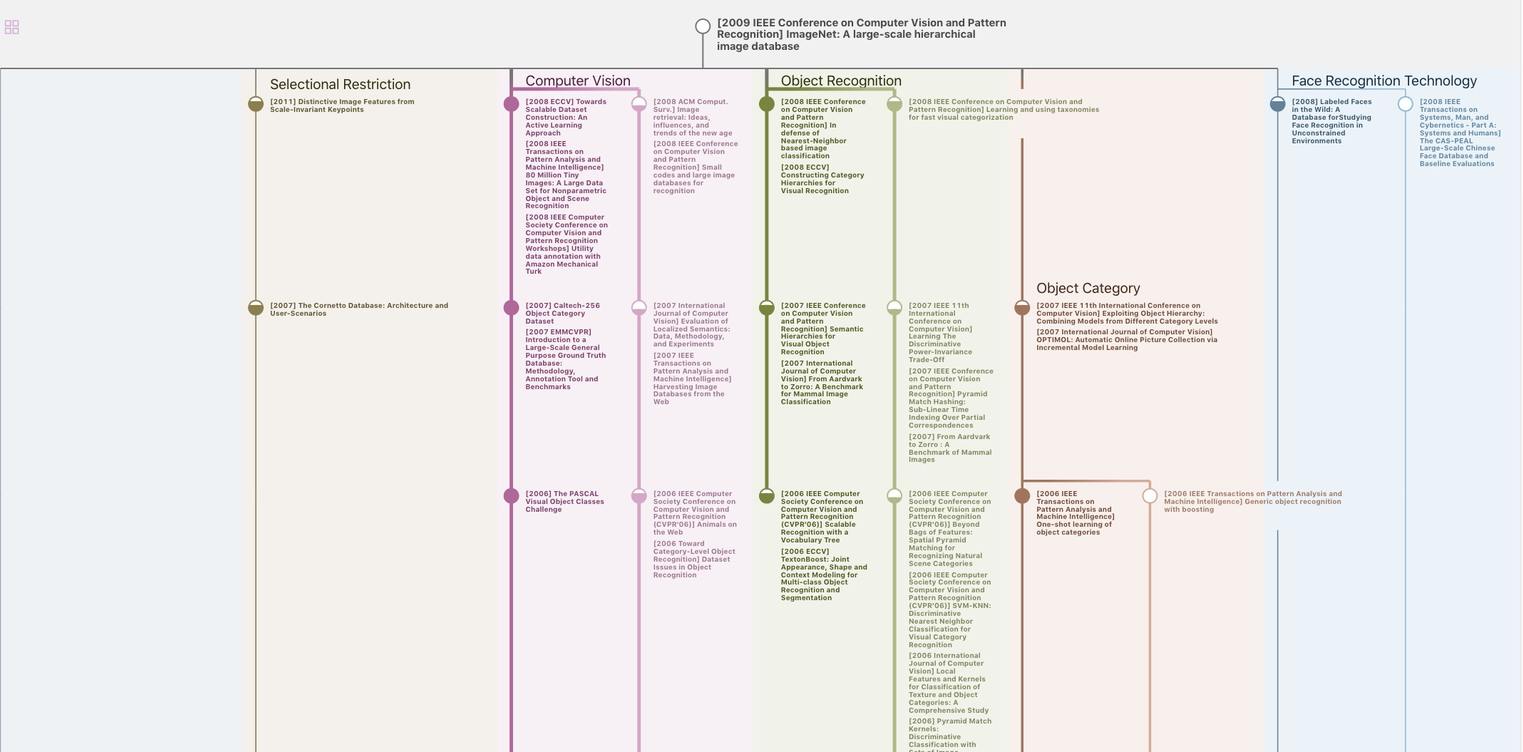
生成溯源树,研究论文发展脉络
Chat Paper
正在生成论文摘要