Generalizable Sample-Efficient Siamese Autoencoder For Tinnitus Diagnosis In Listeners With Subjective Tinnitus
IEEE TRANSACTIONS ON NEURAL SYSTEMS AND REHABILITATION ENGINEERING(2021)
Abstract
Electroencephalogram (EEG)-based neurofeedback has been widely studied for tinnitus therapy in recent years. Most existing research relies on experts' cognitive prediction, and studies based on machine learning and deep learning are either data-hungry or not well generalizable to new subjects. In this paper, we propose a robust, data-efficient model for distinguishing tinnitus from the healthy state based on EEG-based tinnitus neurofeedback. We propose trend descriptor, a feature extractor with lower fineness, to reduce the effect of electrode noises on EEG signals, and a siamese encoder-decoder network boosted in a supervised manner to learn accurate alignment and to acquire high-quality transferable mappings across subjects and EEG signal channels. Our experiments show the proposed method significantly outperforms state-of-the-art algorithms when analyzing subjects' EEG neurofeedback to 90dB and 100dB sound, achieving an accuracy of 91.67%-94.44% in predicting tinnitus and control subjects in a subject-independent setting. Our ablation studies on mixed subjects and parameters show the method's stability in performance.
MoreTranslated text
Key words
Electroencephalography, Brain modeling, Market research, Training, Neurofeedback, Medical treatment, Auditory system, EEG, subject-independent, siamese autoencoder, domain alignment, trend descriptor, tinnitus
AI Read Science
Must-Reading Tree
Example
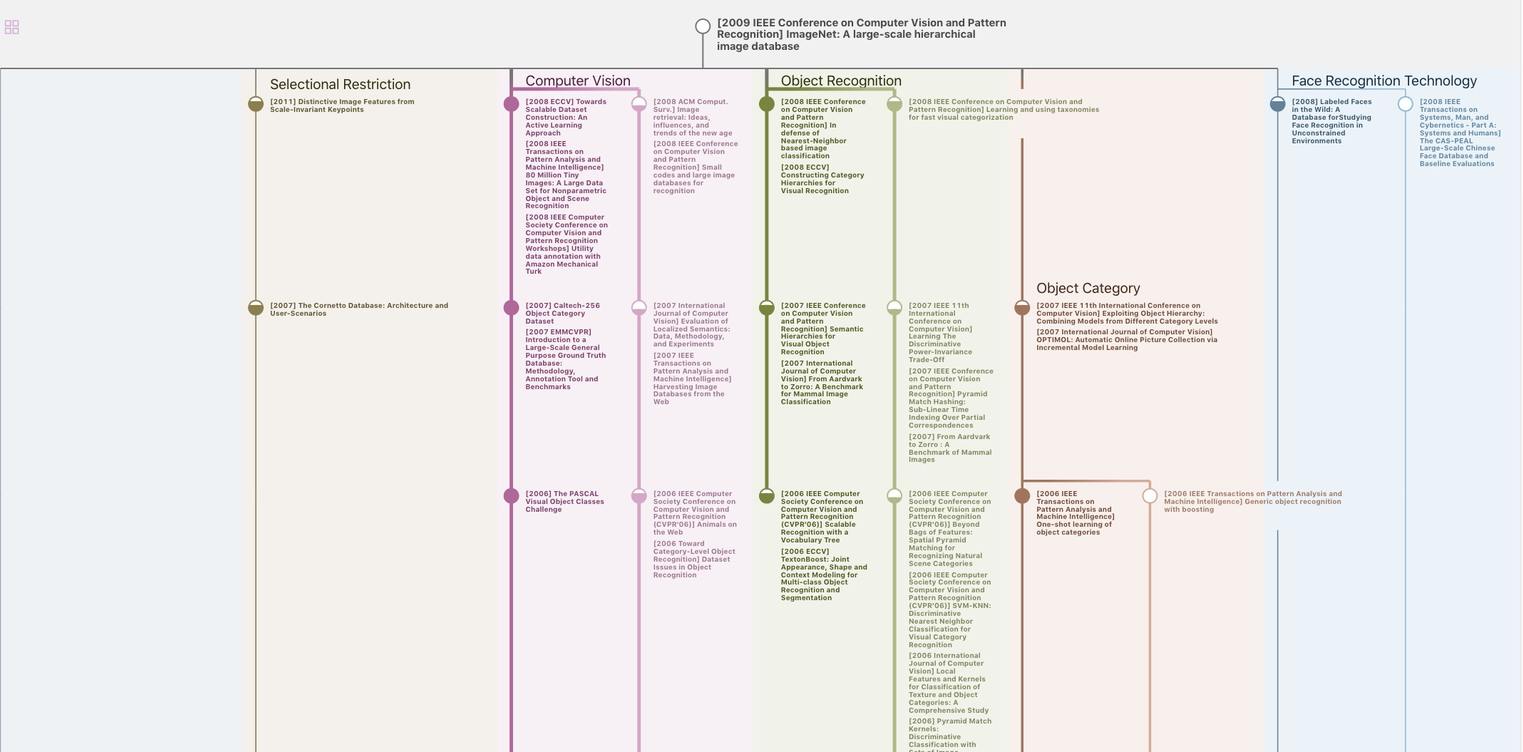
Generate MRT to find the research sequence of this paper
Chat Paper
Summary is being generated by the instructions you defined