Identifying quantum phases via disentanglement based on deep reinforcement learning
arXiv (Cornell University)(2021)
Abstract
Identifying phases of matter is a complicated process, especially in quantum theory, where the complexity of the ground state appears to rise exponentially with system size. Typically, physicists have been responsible for identifying suitable order parameters for the identification of the various phases. The entanglement of quantum many-body systems exhibits rich structures and can be determined over the phase diagram. The intriguing question of the relationship between entanglement and quantum phase change has recently been addressed. As a method that works directly on the entanglement structure, disentanglement can provide factual information on the entanglement structure. In this work, we follow a radically different approach to identifying quantum phases: we utilize reinforcement learning (RL) approaches to develop an efficient variational quantum circuit that disentangles the ground-state of Ising spin chain systems. We show that the specified quantum circuit structure of the tested models correlates to a phase transition in the behaviour of the entanglement. We show a similar universal quantum circuit structure for ground states in the same phase to reduce entanglement entropy. This study sheds light on characterizing quantum phases with the types of entanglement structures of the ground states.
MoreTranslated text
Key words
quantum phases,deep reinforcement learning,disentanglement
AI Read Science
Must-Reading Tree
Example
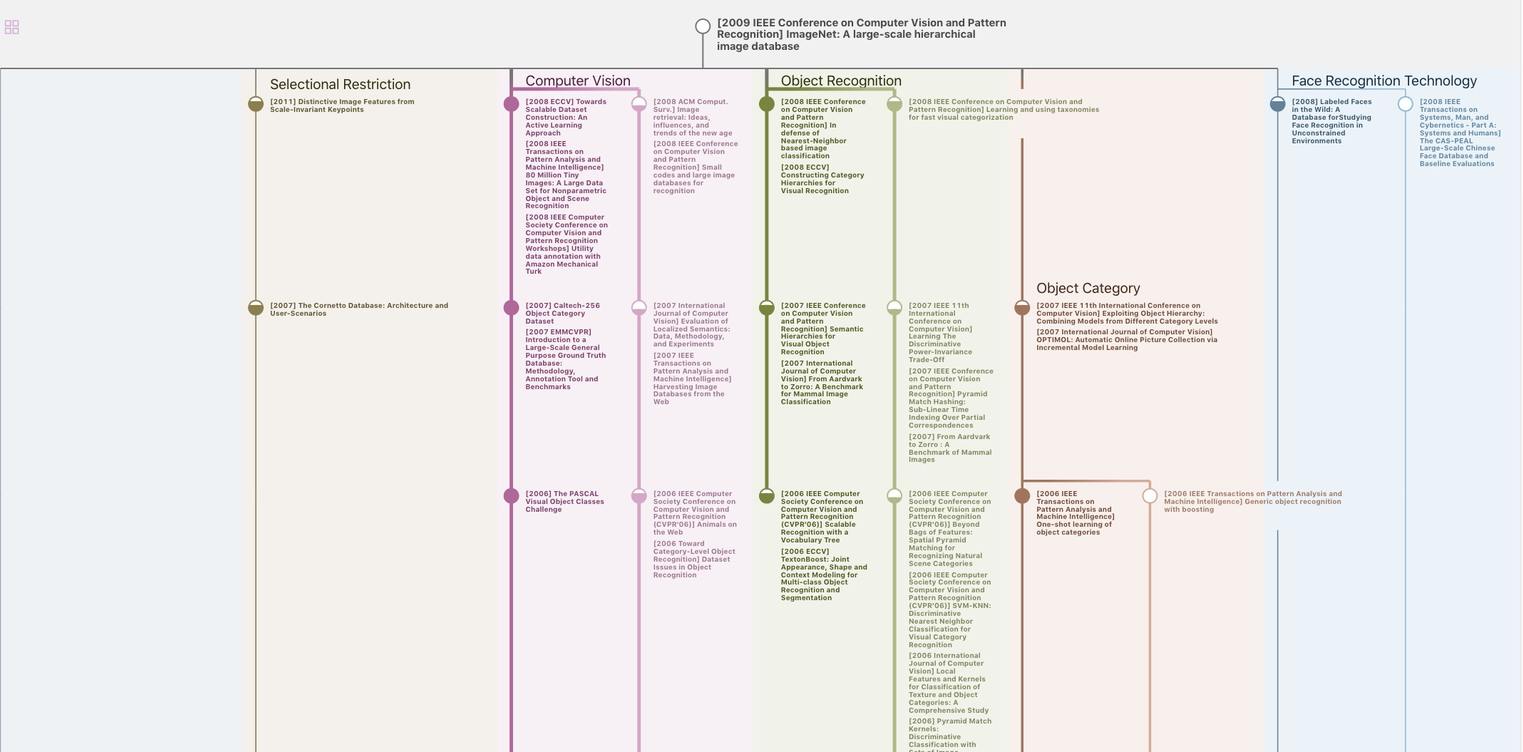
Generate MRT to find the research sequence of this paper
Chat Paper
Summary is being generated by the instructions you defined