SGN: Sparse Gauss-Newton for Accelerated Sensitivity Analysis
ACM Transactions on Graphics(2022)
摘要
AbstractWe present a sparse Gauss-Newton solver for accelerated sensitivity analysis with applications to a wide range of equilibrium-constrained optimization problems. Dense Gauss-Newton solvers have shown promising convergence rates for inverse problems, but the cost of assembling and factorizing the associated matrices has so far been a major stumbling block. In this work, we show how the dense Gauss-Newton Hessian can be transformed into an equivalent sparse matrix that can be assembled and factorized much more efficiently. This leads to drastically reduced computation times for many inverse problems, which we demonstrate on a diverse set of examples. We furthermore show links between sensitivity analysis and nonlinear programming approaches based on Lagrange multipliers and prove equivalence under specific assumptions that apply for our problem setting.
更多查看译文
关键词
Sensitivity analysis, sparse Gauss-Newton, equilibrium-constrained optimization, nonlinear least-squares
AI 理解论文
溯源树
样例
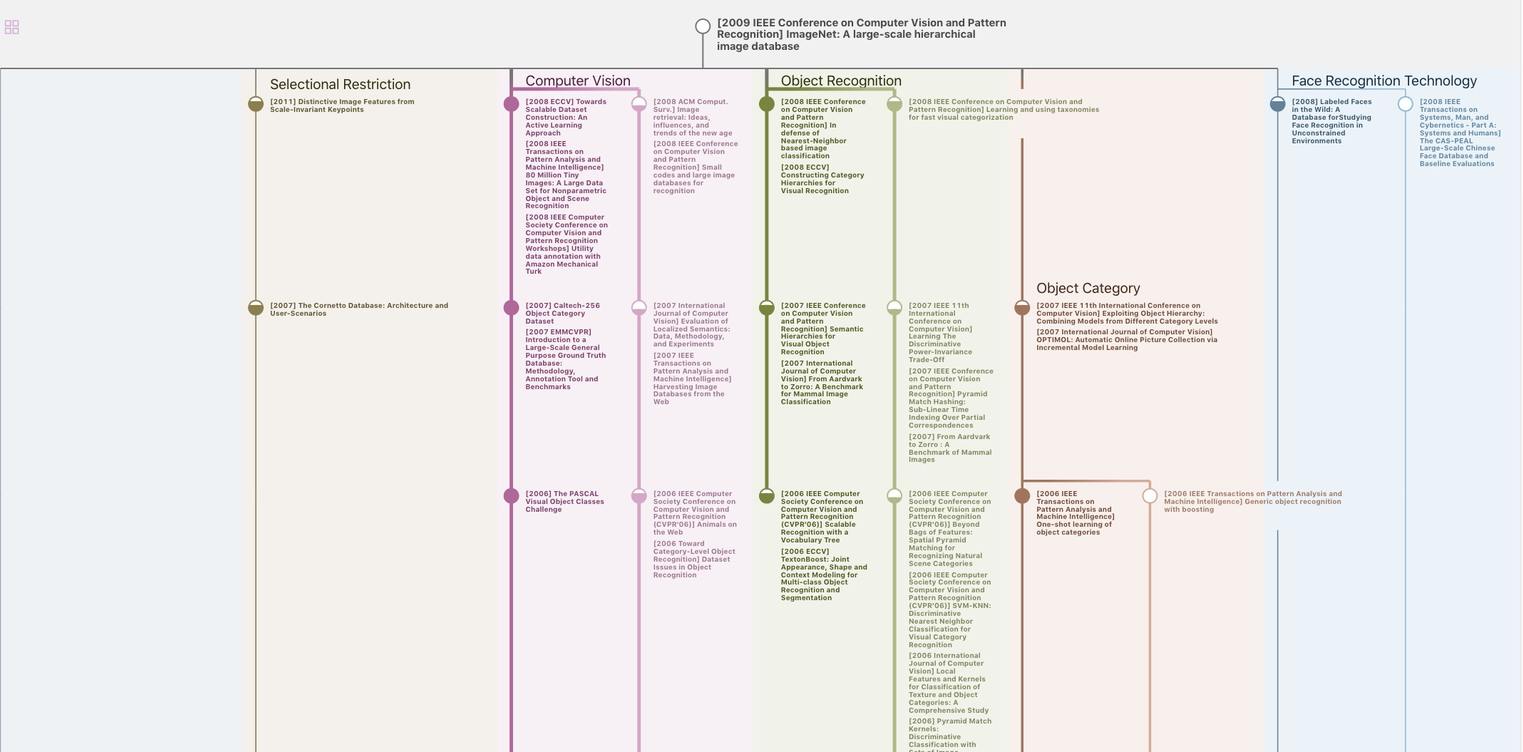
生成溯源树,研究论文发展脉络
Chat Paper
正在生成论文摘要