MACCIF-TDNN: Multi Aspect Aggregation of Channel and Context Interdependence Features in TDNN-Based Speaker Verification
2021 IEEE Automatic Speech Recognition and Understanding Workshop (ASRU)(2021)
摘要
Most of the recent state-of-the-art results for speaker verification are achieved by X-vector and its subsequent variants. In this paper, we propose a new network architecture which aggregates the channel and context interdependence features from multi aspects based on Time Delay Neural Network (TDNN). Firstly, we use the SE-Res2Blocks as in ECAPA-TDNN to explicitly model the channel interdependence to realize adaptive calibration of channel features, and process local context features in a multi-scale way at a more granular level compared with conventional TDNN-based methods. Secondly, we explore to use the encoder structure of Transformer to model the global context interdependence features at an utterance level which can capture better long term temporal characteristics. Before the pooling layer, we aggregate the outputs of SE-Res2Blocks and Transformer Encoders to leverage the complementary channel and context interdependence features learned by themself respectively. Finally, instead of performing a single attentive statistics pooling, we also find it beneficial to extend the pooling method in a multi-head way which can discriminate features from multiple aspects. The proposed MACCIF-TDNN architecture can outperform most of the state-of-the-art TDNN based systems on VoxCeleb1 test sets.
更多查看译文
关键词
speaker verification,speaker recognition,x-vector,Transformer encoder,ECAPA
AI 理解论文
溯源树
样例
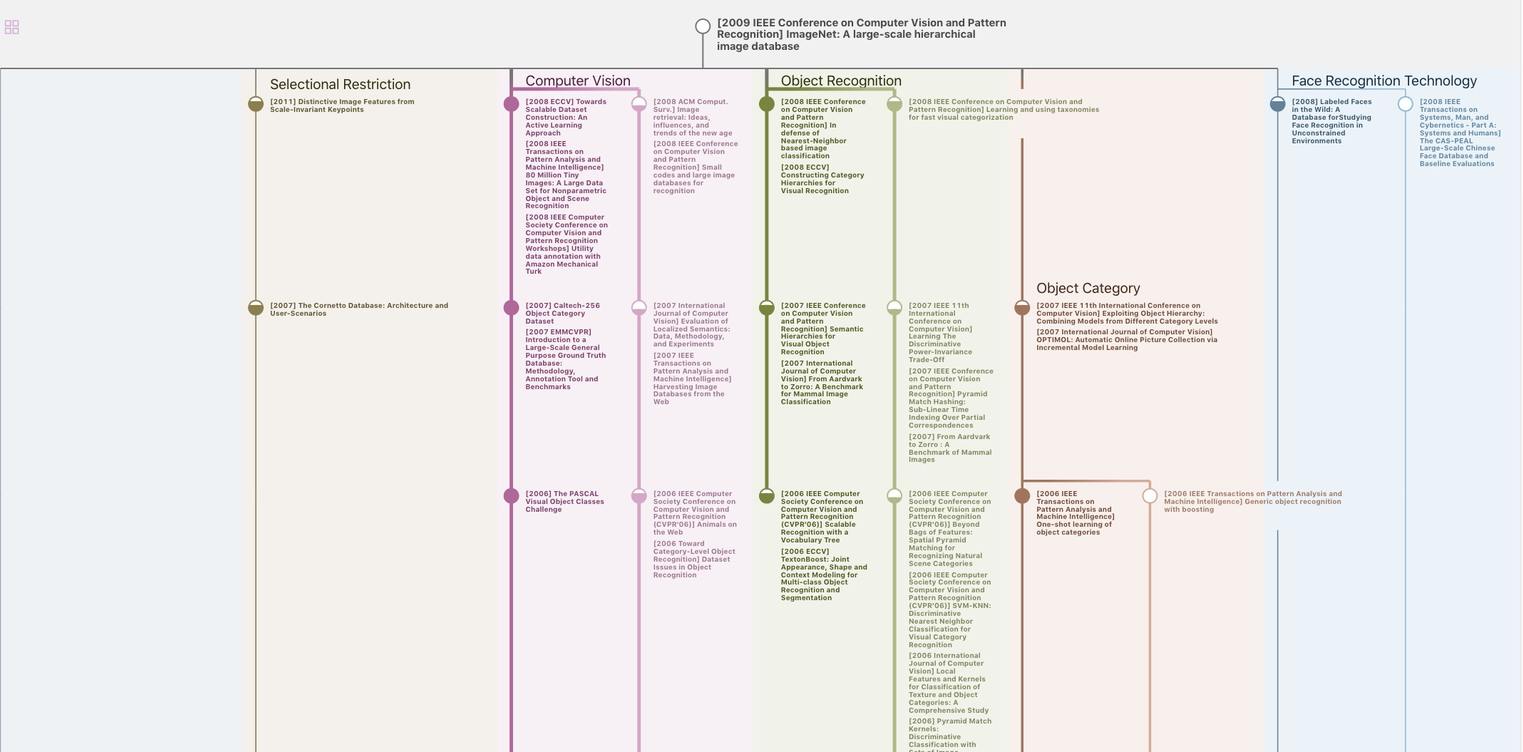
生成溯源树,研究论文发展脉络
Chat Paper
正在生成论文摘要