Rapid Parameter Estimation Of A Two-Component Neutron Star Model With Spin Wandering Using A Kalman Filter
MONTHLY NOTICES OF THE ROYAL ASTRONOMICAL SOCIETY(2021)
Abstract
The classic, two-component, crust-superfluid model of a neutron star can be formulated as a noise-driven, linear dynamical system, in which the angular velocities of the crust and superfluid are tracked using a Kalman filter applied to electromagnetic pulse timing data and gravitational-wave data, when available. Here it is shown how to combine the marginal likelihood of the Kalman filter and nested sampling to estimate full posterior distributions of the six model parameters, extending previous analyses based on a maximum-likelihood approach. The method is tested across an astrophysically plausible parameter domain using Monte Carlo simulations. It recovers the injected parameters to less than or similar to 10 percent for time series containing similar to 10(3) samples, typical of long-term pulsar timing campaigns. It runs efficiently in O(1) CPU-hr for data sets of the above size. In a present-day observational scenario, when electromagnetic data are available only, the method accurately estimates three parameters: the relaxation time, the ensemble-averaged spin-down of the system, and the amplitude of the stochastic torques applied to the crust. In a future observational scenario, where gravitational-wave data are also available, the method also estimates the ratio between the moments of inertia of the crust and the superfluid, the amplitude of the stochastic torque applied to the superfluid, and the crust-superfluid lag. These empirical results are consistent with a formal identifiability analysis of the linear dynamical system.
MoreTranslated text
Key words
methods: data analysis, stars: neutron, pulsars: general
AI Read Science
Must-Reading Tree
Example
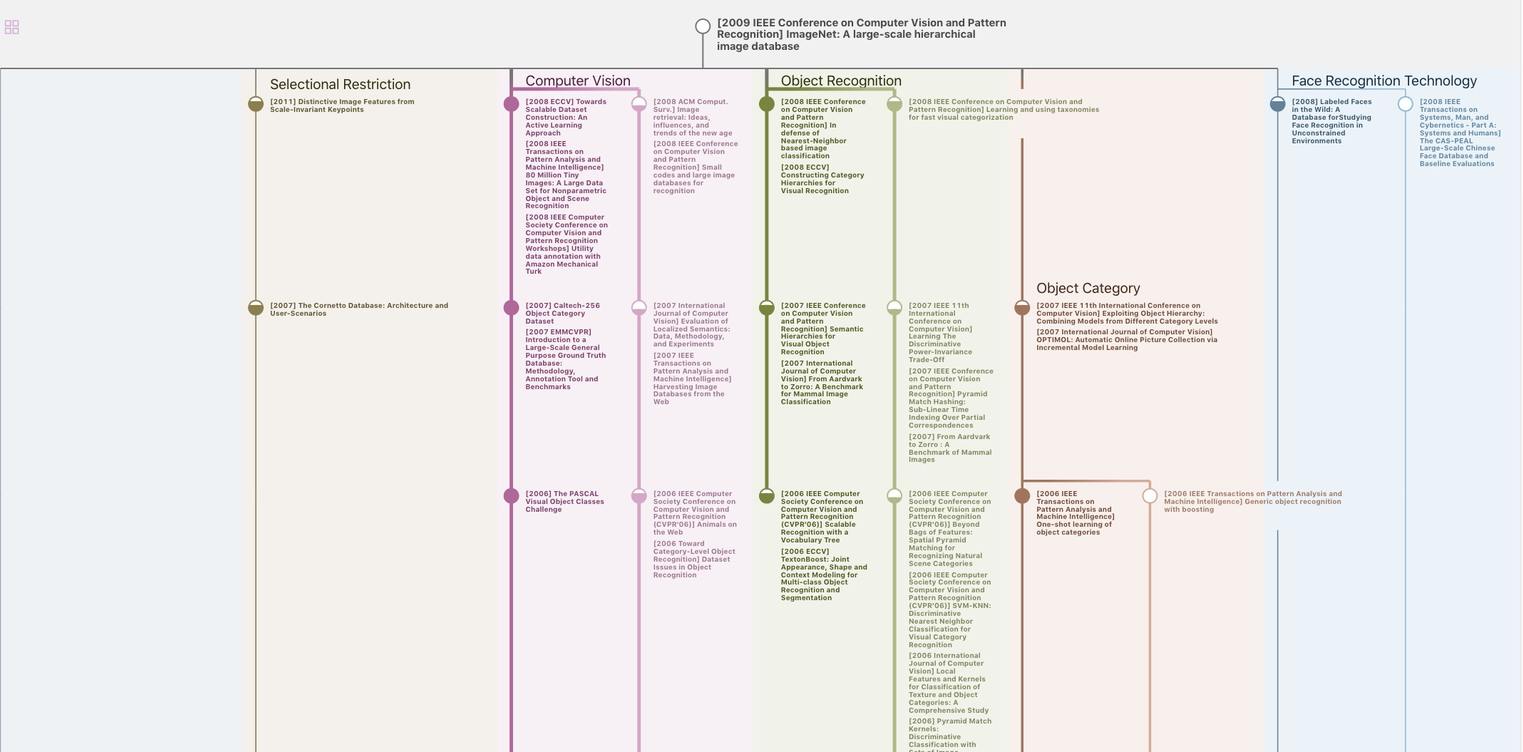
Generate MRT to find the research sequence of this paper
Chat Paper
Summary is being generated by the instructions you defined