Prototyping Machine Learning Through Diffractive Art Practice
PROCEEDINGS OF THE 2021 ACM DESIGNING INTERACTIVE SYSTEMS CONFERENCE (DIS 2021)(2021)
摘要
In this paper, we outline a diffractive practice of machine learning (ML) in the frame of material-centered interaction design. To this aim, we review related work in ML, HCI, design, new interfaces for musical expression, and computational art, and introduce two practice-based studies of music performance and robotic art based on interactive machine learning tools, with the hope of revealing the computational materiality of ML, and the potential of embodiment to craft prototypes of ML that reconfigure conceptual or technical approaches to ML. We derive five interference conditions for such art-based ML prototypes-situational whole, small data, shallow model, learnable algorithm, and somaesthetic behaviour- and describe their widening of design and engineering practices of ML prototyping. Finally, we sketch how a process of intra-active machine learning could complement that of interactive machine learning to take materiality as an entry point for ML design within HCI.
更多查看译文
关键词
Machine Learning, Art Practice, Design, Diffractive Methods
AI 理解论文
溯源树
样例
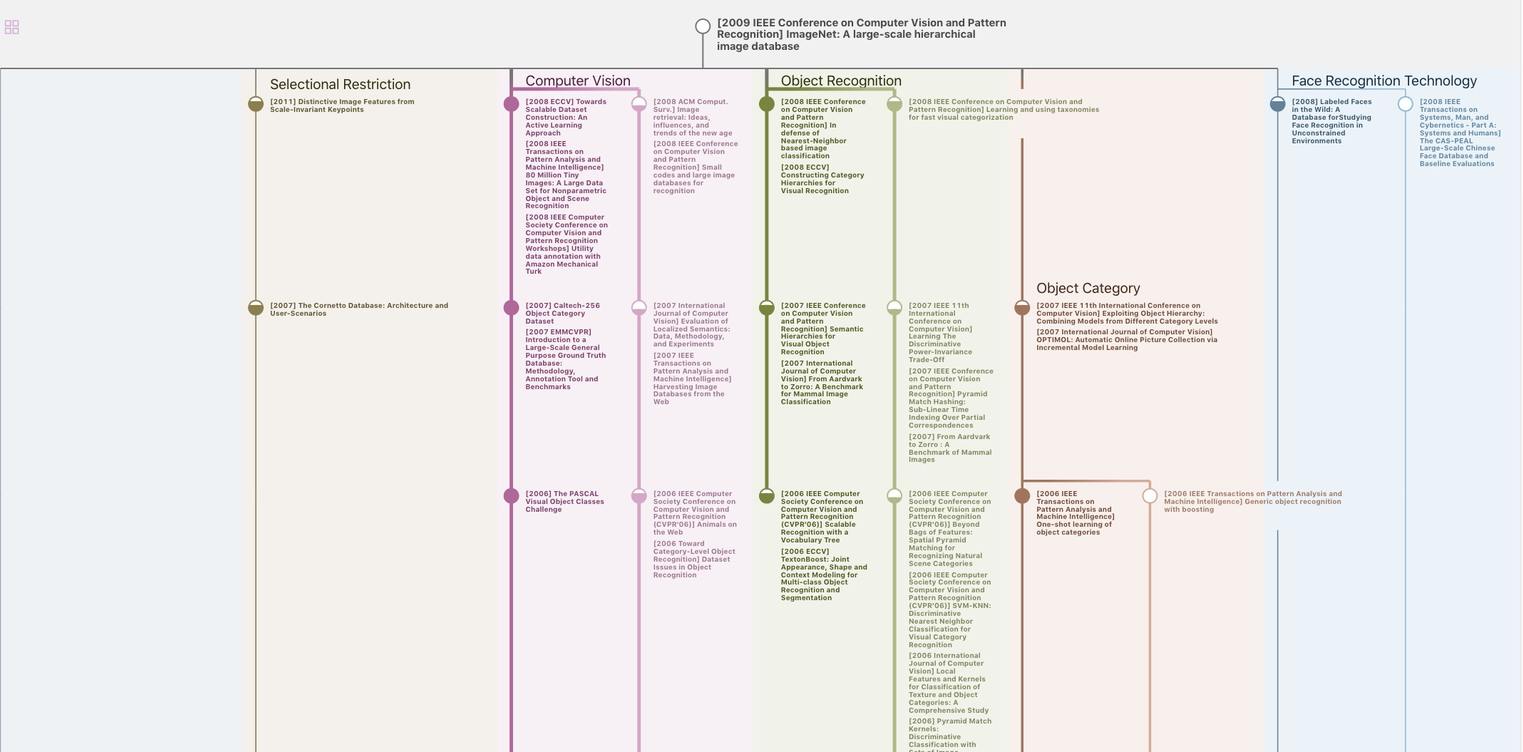
生成溯源树,研究论文发展脉络
Chat Paper
正在生成论文摘要