Distributed adaptive Huber regression
COMPUTATIONAL STATISTICS & DATA ANALYSIS(2022)
摘要
Distributed data naturally arise in scenarios involving multiple sources of observations, each stored at a different location. Directly pooling all the data together is often prohibited due to limited bandwidth and storage, or due to privacy protocols. A new robust distributed algorithm is introduced for fitting linear regressions when data are subject to heavy-tailed and/or asymmetric errors with finite second moments. The algorithm only communicates gradient information at each iteration, and therefore is communication-efficient. To achieve the bias-robustness tradeoff, the key is a novel double-robustification approach that applies on both the local and global objective functions. Statistically, the resulting estimator achieves the centralized nonasymptotic error bound as if all the data were pooled together and came from a distribution with sub-Gaussian tails. Under a finite (2 + delta)-th moment condition, a Berry-Esseen bound for the distributed estimator is established, based on which robust confidence intervals are constructed. In high dimensions, the proposed doubly-robustified loss function is complemented with l(1) -penalization for fitting sparse linear models with distributed data. Numerical studies further confirm that compared with extant distributed methods, the proposed methods achieve near-optimal accuracy with low variability and better coverage with tighter confidence width. (C) 2021 Elsevier B.V. All rights reserved.
更多查看译文
关键词
Adaptive Huber regression,Communication efficiency,Distributed inference,Heavy-tailed distribution,Nonasymptotic analysis
AI 理解论文
溯源树
样例
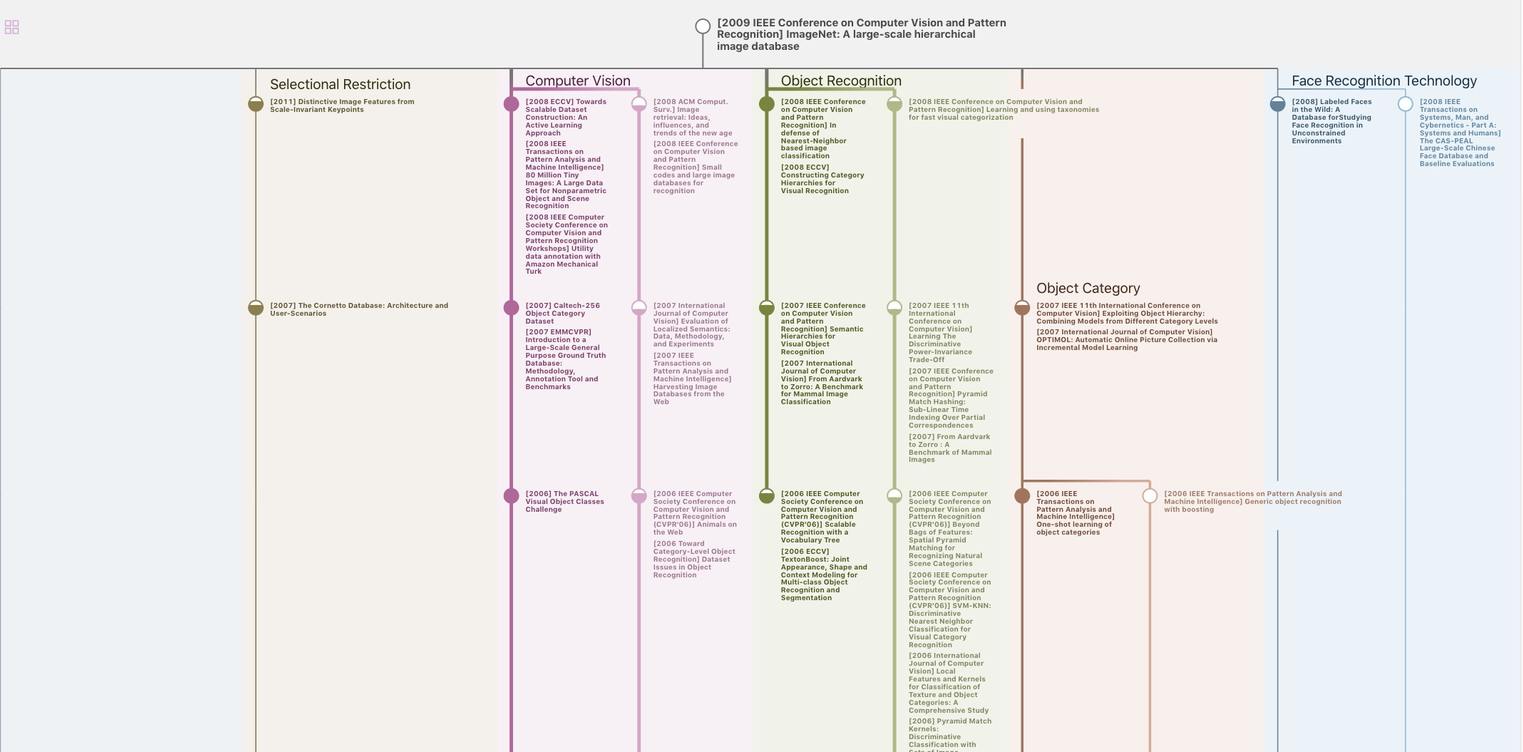
生成溯源树,研究论文发展脉络
Chat Paper
正在生成论文摘要