LightFuse: Lightweight CNN based Dual-exposure Fusion
ArXiv(2022)
摘要
Deep convolutional neural networks (DCNNs) have aided high dynamic range (HDR) imaging recently and have received a lot of attention. The quality of DCNN-generated HDR images has overperformed the traditional counterparts. However, DCNNs are prone to be computationally intensive and power-hungry, and hence cannot be implemented on various embedded computing platforms with limited power and hardware resources. Embedded systems have a huge market, and utilizing DCNNs' powerful functionality into them will further reduce human intervention. To address the challenge, we propose LightFuse, a lightweight CNN-based algorithm for extreme dual-exposure image fusion, which achieves better functionality than a conventional DCNN and can be deployed in embedded systems. Two sub-networks are utilized: a GlobalNet (G) and a DetailNet (D). The goal of G is to learn the global illumination information on the spatial dimension, whereas D aims to enhance local details on the channel dimension. Both G and D are based solely on depthwise convolution (D_Conv) and pointwise convolution (P_Conv) to reduce required parameters and computations. Experimental results show that this proposed technique could generate HDR images in extremely exposed regions with sufficient details to be legible. Our model outperforms other state-of-the-art approaches in peak signal-to-noise ratio (PSNR) score by 0.9 to 8.7 while achieving 16.7 to 306.2 times parameter reduction.
更多查看译文
关键词
lightweight cnn,fusion,lightfuse,dual-exposure
AI 理解论文
溯源树
样例
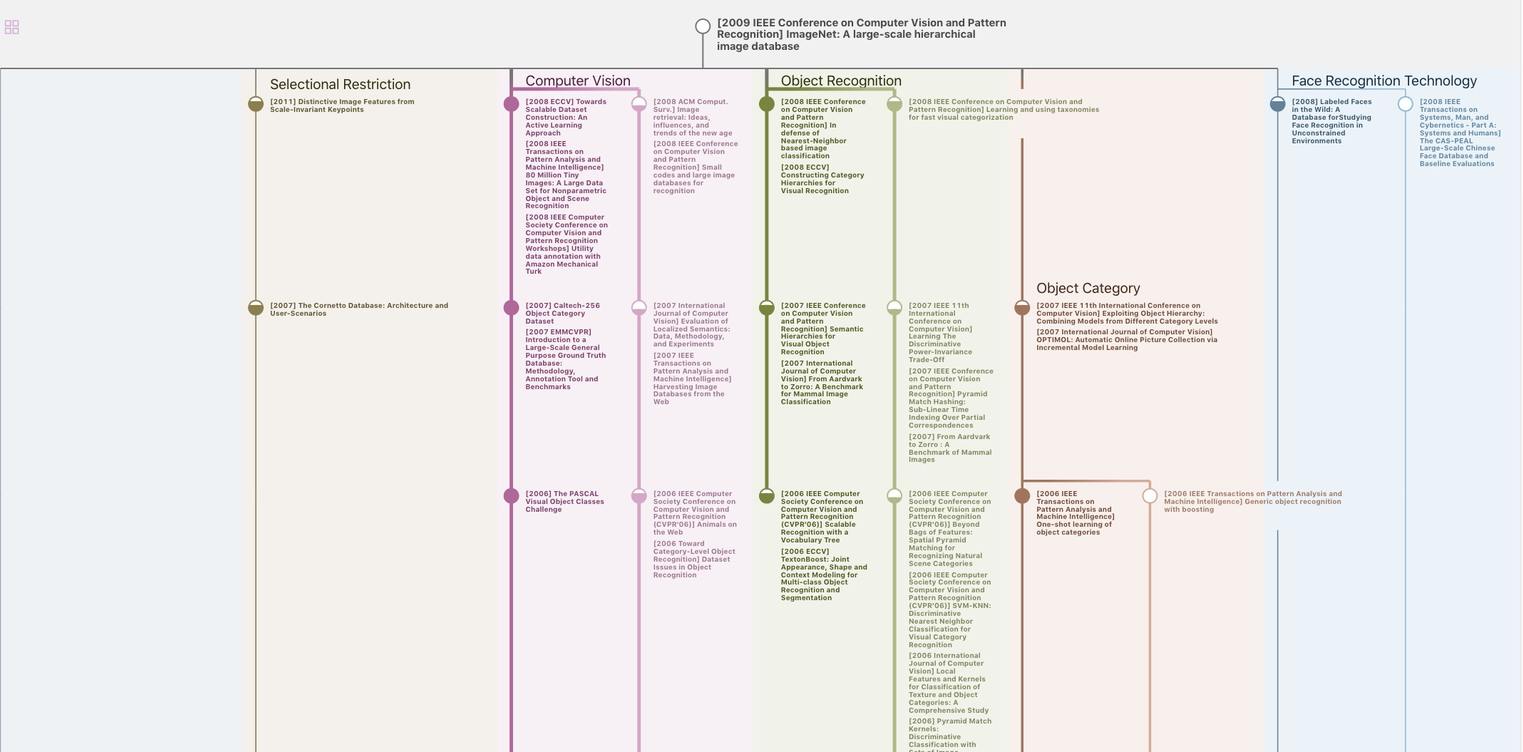
生成溯源树,研究论文发展脉络
Chat Paper
正在生成论文摘要