Sequential Fusion via Bounding Box and Motion PointPainting for 3D Objection Detection
2021 18th Conference on Robots and Vision (CRV)(2021)
摘要
Due to the complementary characteristics of camera and LiDAR data, recent research efforts have been focused on designing 3D object detectors capable of fusing images and point clouds. However, LiDAR-based detectors currently achieve better performance on KITTI and Waymo benchmark datasets [1], [2] when compared to fusion methods. This result is counter-intuitive, as fusing information from the two modalities should result in performance that at least matches the performance of LiDAR-only methods. Pointpainting [3] attempts to address this gap by sequential fusion, which solves the issue of misalignment between image view and LiDAR BEV. In this paper, we propose class-aware and class-agnostic point painting methods which employ predicted bounding boxes from image-based 2D object detectors to extract coarse image semantics instead of full scene semantic segmentation used in [3]. In addition, a motion point painting method is proposed to fuse motion cues as a way to focus attention on dynamic objects when they can be reliably distinguished from the scene, as is the case when the sensors are static. Our experiments on KITTI [1] show a 3% mAP improvement on car class for bounding box methods compared to PointPainting [3]. In addition, motion painting shows an improvement of 1:45% mAP for car class and 2:99% for pedestrian class on our proprietary traffic dataset. Finally, we conduct a range-binned evaluation on KITTI dataset using two different LiDAR stream and show that relative gain of sequential fusion methods is dependent on the selected LiDAR stream.
更多查看译文
关键词
3D object detection,pointpainting,camera/lidar,sensor fusion,sequential fusion,motion cues,temporal aggregation
AI 理解论文
溯源树
样例
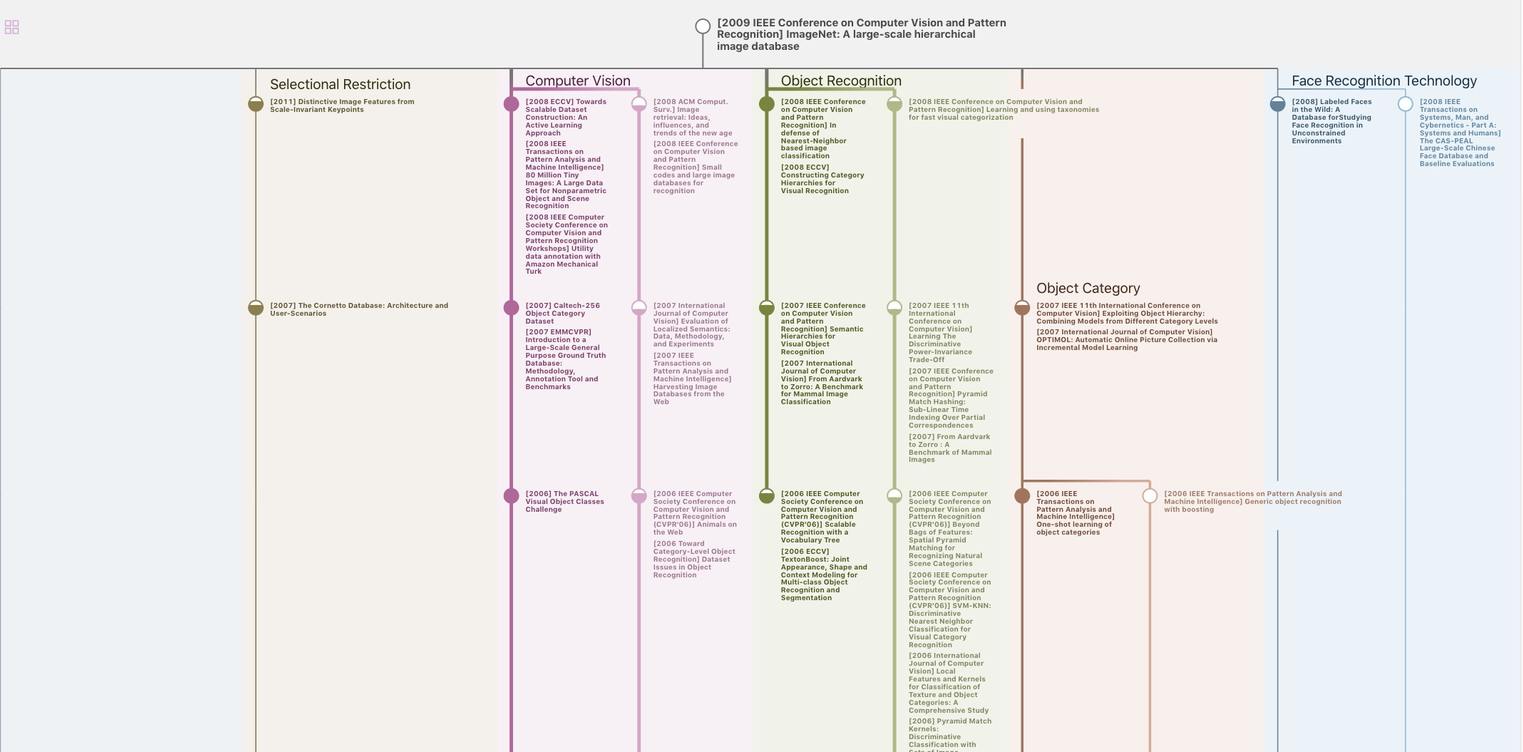
生成溯源树,研究论文发展脉络
Chat Paper
正在生成论文摘要