Creating Unbiased Public Benchmark Datasets with Data Leakage Prevention for Predictive Process Monitoring
BUSINESS PROCESS MANAGEMENT WORKSHOPS, BPM 2021(2022)
摘要
Advances in AI, and especially machine learning, are increasingly drawing research interest and efforts towards predictive process monitoring, the subfield of process mining (PM) that concerns predicting next events, process outcomes and remaining execution times. Unfortunately, researchers use a variety of datasets and ways to split them into training and test sets. The documentation of these preprocessing steps is not always complete. Consequently, research results are hard or even impossible to reproduce and to compare between papers. At times, the use of non-public domain knowledge further hampers the fair competition of ideas. Often the training and test sets are not completely separated, a data leakage problem particular to predictive process monitoring. Moreover, test sets usually suffer from bias in terms of both the mix of case durations and the number of running cases. These obstacles pose a challenge to the field's progress. The contribution of this paper is to identify and demonstrate the importance of these obstacles and to propose preprocessing steps to arrive at unbiased benchmark datasets in a principled way, thus creating representative test sets without data leakage with the aim of levelling the playing field, promoting open science and contributing to more rapid progress in predictive process monitoring.
更多查看译文
关键词
Predictive process monitoring, Remaining time prediction, Bias, Benchmarking, Reproducibility, Datasets, Preprocessing
AI 理解论文
溯源树
样例
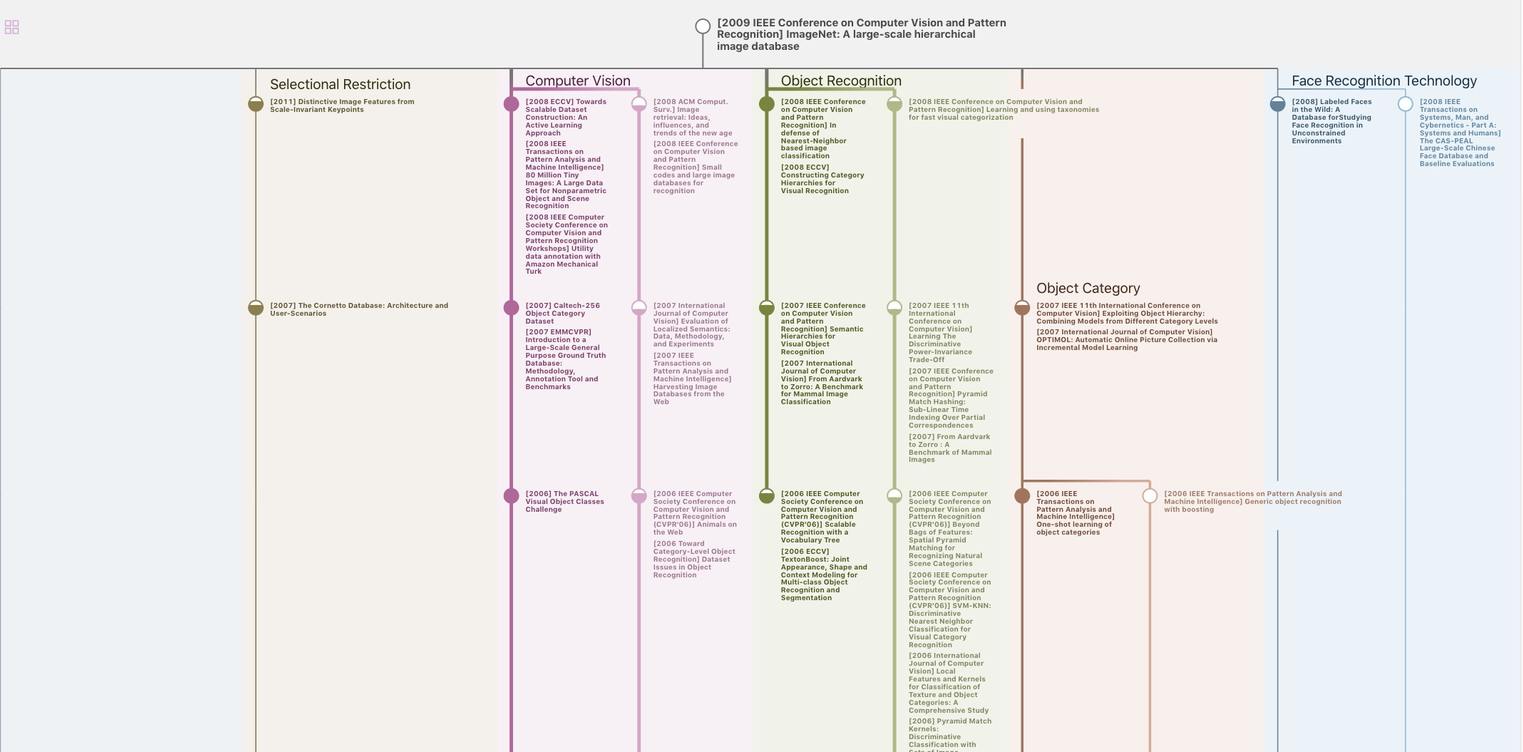
生成溯源树,研究论文发展脉络
Chat Paper
正在生成论文摘要