Deep Gaussian Process Emulation using Stochastic Imputation
arxiv(2022)
摘要
Deep Gaussian processes (DGPs) provide a rich class of models that can better represent functions with varying regimes or sharp changes, compared to conventional GPs. In this work, we propose a novel inference method for DGPs for computer model emulation. By stochastically imputing the latent layers, our approach transforms a DGP into a linked GP: a novel emulator developed for systems of linked computer models. This transformation permits an efficient DGP training procedure that only involves optimizations of conventional GPs. In addition, predictions from DGP emulators can be made in a fast and analytically tractable manner by naturally utilizing the closed form predictive means and variances of linked GP emulators. We demonstrate the method in a series of synthetic examples and empirical applications, and show that it is a competitive candidate for DGP surrogate inference, combining efficiency that is comparable to doubly stochastic variational inference and uncertainty quantification that is comparable to the fully-Bayesian approach. A $\texttt{Python}$ package $\texttt{dgpsi}$ implementing the method is also produced and available at https://github.com/mingdeyu/DGP.
更多查看译文
关键词
Elliptical slice sampling,Linked Gaussian processes,Option Greeks,Surrogate model,Stochastic expectation maximization
AI 理解论文
溯源树
样例
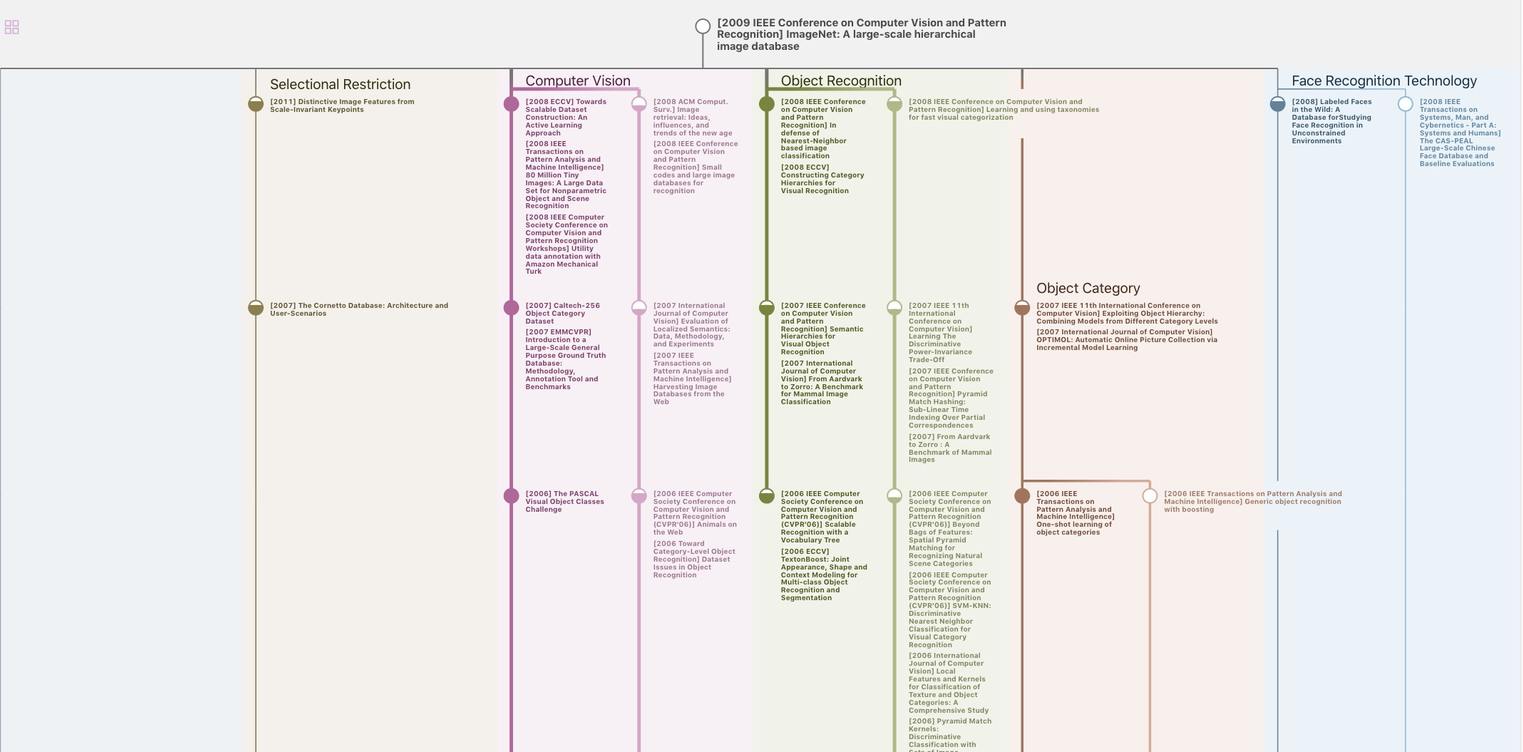
生成溯源树,研究论文发展脉络
Chat Paper
正在生成论文摘要