Interactive Causal Structure Discovery in Earth System Sciences.
ACM SIGKDD Conference on Knowledge Discovery and Data Mining(2021)
Abstract
Causal structure discovery (CSD) models are making inroads into several domains, including Earth system sciences. Their widespread adaptation is however hampered by the fact that the resulting models often do not take into account the domain knowledge of the experts and that it is often necessary to modify the resulting models iteratively. We present a workflow that is required to take this knowledge into account and to apply CSD algorithms in Earth system sciences. At the same time, we describe open research questions that still need to be addressed. We present a way to interactively modify the outputs of the CSD algorithms and argue that the user interaction can be modelled as a greedy finding of the local maximum-a-posteriori solution of the likelihood function, which is composed of the likelihood of the causal model and the prior distribution representing the knowledge of the expert user. We use a real-world data set for examples constructed in collaboration with our co-authors, who are the domain area experts. We show that finding maximally usable causal models in the Earth system sciences or other similar domains is a difficult task which contains many interesting open research questions. We argue that taking the domain knowledge into account has a substantial effect on the final causal models discovered.
MoreTranslated text
Key words
earth system sciences,causal,discovery,structure
AI Read Science
Must-Reading Tree
Example
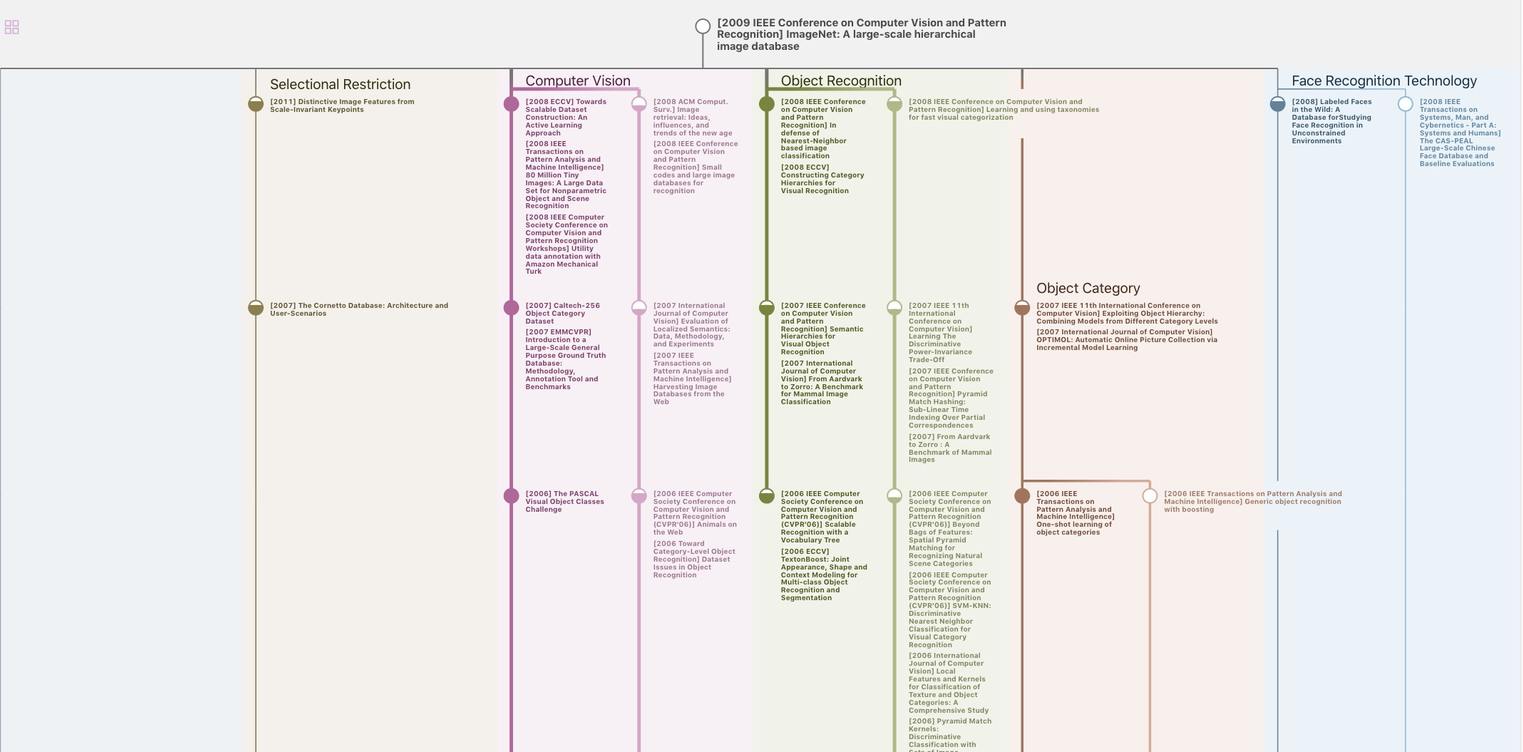
Generate MRT to find the research sequence of this paper
Chat Paper
Summary is being generated by the instructions you defined