Methodology for a global sensitivity analysis with machine learning on an energy system planning model in the context of thermal networks
ENERGY(2021)
Abstract
ABSTR A C T Thermal networks have gained attention in recent research as a means for the European Union to reach its climate targets. The potential has been well established for a multitude of regions. However, there is more to learn regarding the quantity and behaviour of the decision boundary between the use of thermal networks and competing alternatives. This study aims to describe that decision boundary with a parameter analysis of an energy system planning model. First, parameters of the energy system planning model (e.g. the linear heat density) describe a situation. Second, an energy system planning model finds the optimal (economic) energy supply mix for a given situation. Third, a sampling method with machine learning manipulates the values of the parameters to generate new situations, which improves the in-formation on the as of yet unknown decision boundary. And fourth, the final location of the decision boundary is then predicted with machine learning from the optimised set of situations. Illustration of the concept is provided by means of a simplified case study. For that case study it is found that the decision for district heating is bound by a minimum value of several parameters instead of a single parameter. (c) 2021 Elsevier Ltd. All rights reserved.
MoreTranslated text
Key words
Global sensitivity analysis, Parameter analysis, Machine learning, Energy system planning model, District heating
AI Read Science
Must-Reading Tree
Example
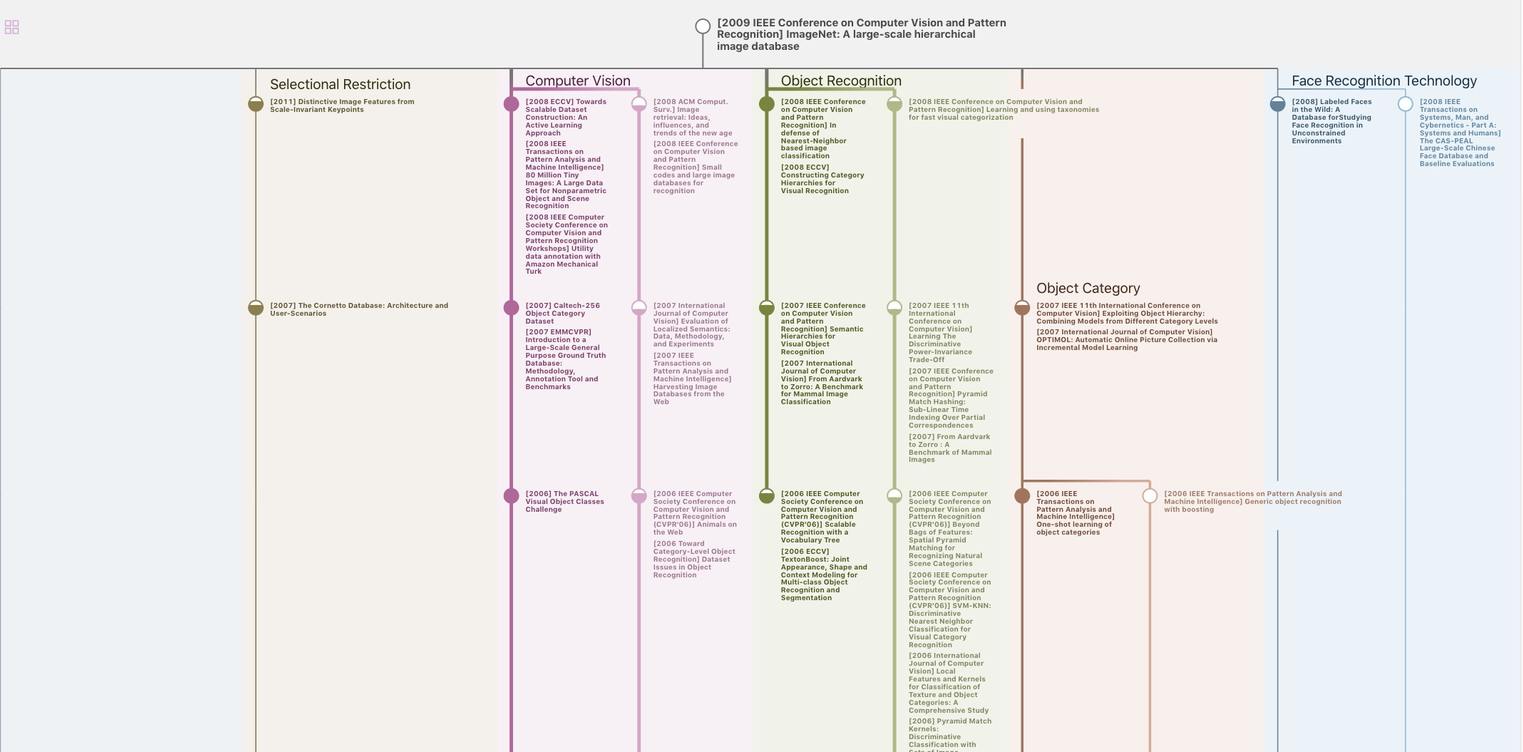
Generate MRT to find the research sequence of this paper
Chat Paper
Summary is being generated by the instructions you defined