End-To-End Deep Neural Network Architectures For Speed And Steering Wheel Angle Prediction In Autonomous Driving
ELECTRONICS(2021)
摘要
The complex decision-making systems used for autonomous vehicles or advanced driver-assistance systems (ADAS) are being replaced by end-to-end (e2e) architectures based on deep-neural-networks (DNN). DNNs can learn complex driving actions from datasets containing thousands of images and data obtained from the vehicle perception system. This work presents the classification, design and implementation of six e2e architectures capable of generating the driving actions of speed and steering wheel angle directly on the vehicle control elements. The work details the design stages and optimization process of the convolutional networks to develop six e2e architectures. In the metric analysis the architectures have been tested with different data sources from the vehicle, such as images, XYZ accelerations and XYZ angular speeds. The best results were obtained with a mixed data e2e architecture that used front images from the vehicle and angular speeds to predict the speed and steering wheel angle with a mean error of 1.06%. An exhaustive optimization process of the convolutional blocks has demonstrated that it is possible to design lightweight e2e architectures with high performance more suitable for the final implementation in autonomous driving.
更多查看译文
关键词
autonomous driving, end-to-end architecture, speed and steering wheel angle prediction, DNN for regression
AI 理解论文
溯源树
样例
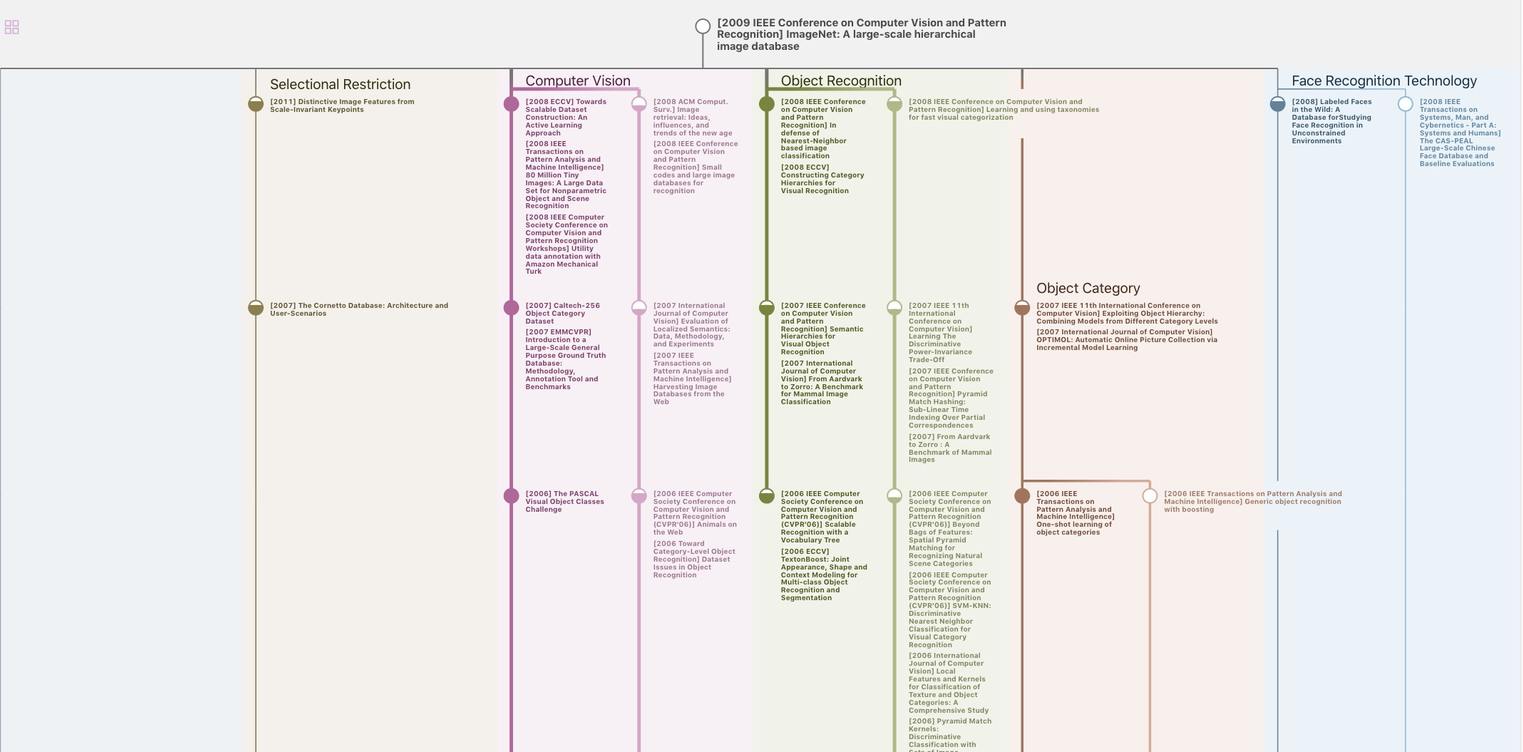
生成溯源树,研究论文发展脉络
Chat Paper
正在生成论文摘要