Classification Of Red Blood Cell Rigidity From Sequence Data Of Blood Flow Simulations Using Neural Networks
SYMMETRY-BASEL(2021)
摘要
Numerical models for the flow of blood and other fluids can be used to design and optimize microfluidic devices computationally and thus to save time and resources needed for production, testing, and redesigning of the physical microfluidic devices. Like biological experiments, computer simulations have their limitations. Data from both the biological and the computational experiments can be processed by machine learning methods to obtain new insights which then can be used for the optimization of the microfluidic devices and also for diagnostic purposes. In this work, we propose a method for identifying red blood cells in flow by their stiffness based on their movement data processed by neural networks. We describe the performed classification experiments and evaluate their accuracy in various modifications of the neural network model. We outline other uses of the model for processing data from video recordings of blood flow. The proposed model and neural network methodology classify healthy and more rigid (diseased) red blood cells with the accuracy of about 99.5% depending on the selected dataset that represents the flow of a suspension of blood cells of various levels of stiffness.
更多查看译文
关键词
neural networks, microfluidic devices, red blood cells classification, sequential data
AI 理解论文
溯源树
样例
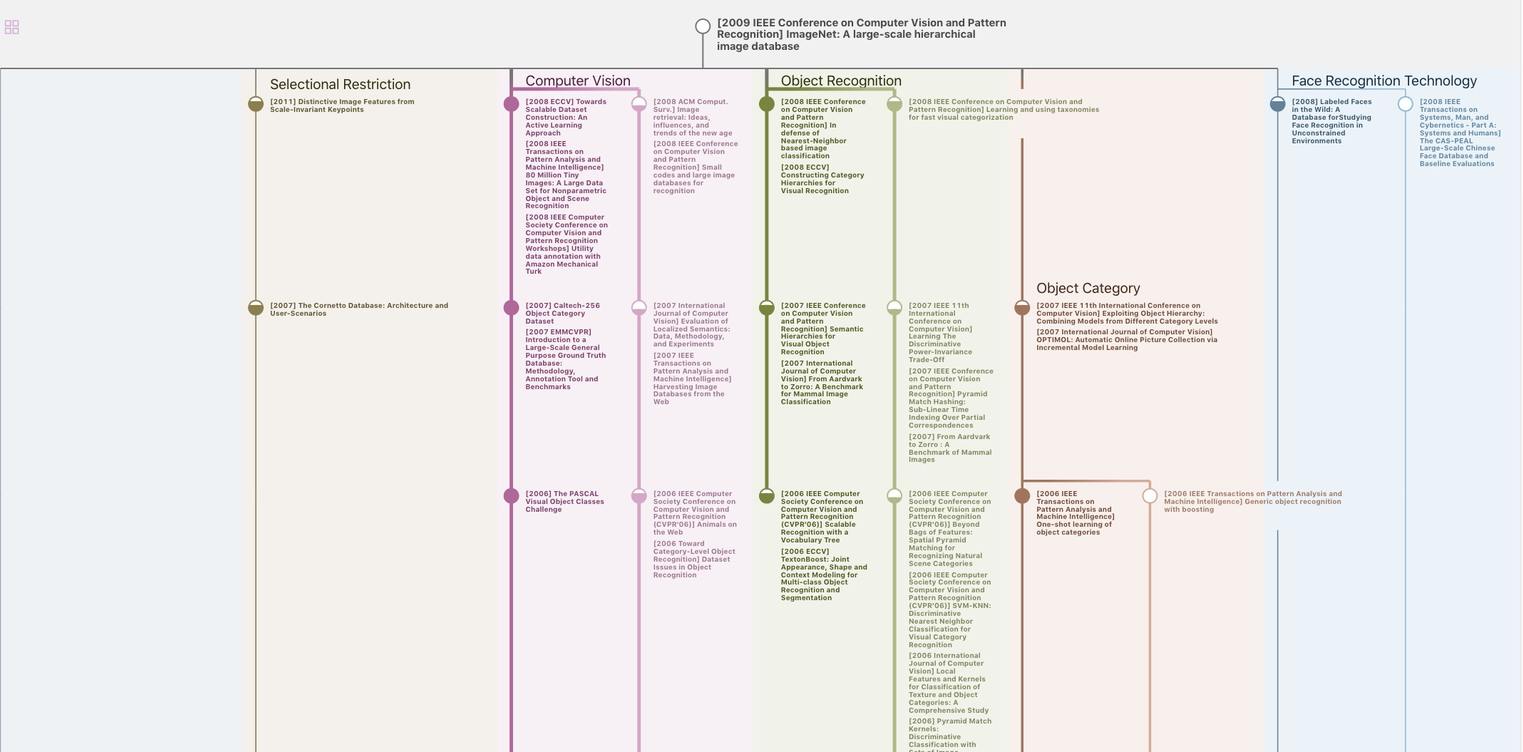
生成溯源树,研究论文发展脉络
Chat Paper
正在生成论文摘要