Deep Learning Techniques For Predicting Nonlinear Multi-Component Seismic Responses Of Structural Buildings
COMPUTERS & STRUCTURES(2021)
摘要
This paper presents a new approach for nonlinear multi-component seismic response prediction of structures using hybrid deep learning techniques. Modern recurrent neural networks map the relationship between acceleration time-series of the base/ground of a building and the superstructure, as a form of nonlinear time-history analysis method. Seismic responses were measured in three components which enables multi-component seismic predictions with adequate deep learning architectures. While long short-term memory (LSTM) neural networks can obtain data from a single component per surrogate model, hybrid convolutional-LSTMs (ConvLSTM) neural networks are utilized for multi-component purposes. A guide for pre-processing data and structuring the architecture of deep neural networks are proposed. Also, two filtering methods are compared, Fast Fourier Transform (FFT) Butterworth filter and discrete wavelet transform (DWT) decomposition. Decimation is implemented to reduce the features to useful values, as a dimension reduction approach. With enhancements to the architecture of the network, training time can be reduced significantly, and accuracy could be further improved. A challenging case study is addressed that covers an industrial level practical building. Results show that proposed hybrid models can even predict the capacity curves of a structure indirectly, providing new prospects for engineers to evaluate the seismic performance of a building. (C) 2021 Elsevier Ltd. All rights reserved.
更多查看译文
关键词
Deep neural networks, Convolutional long short-term memory neural networks, Nonlinear seismic response prediction, Multi-component response, Discrete wavelet transforms
AI 理解论文
溯源树
样例
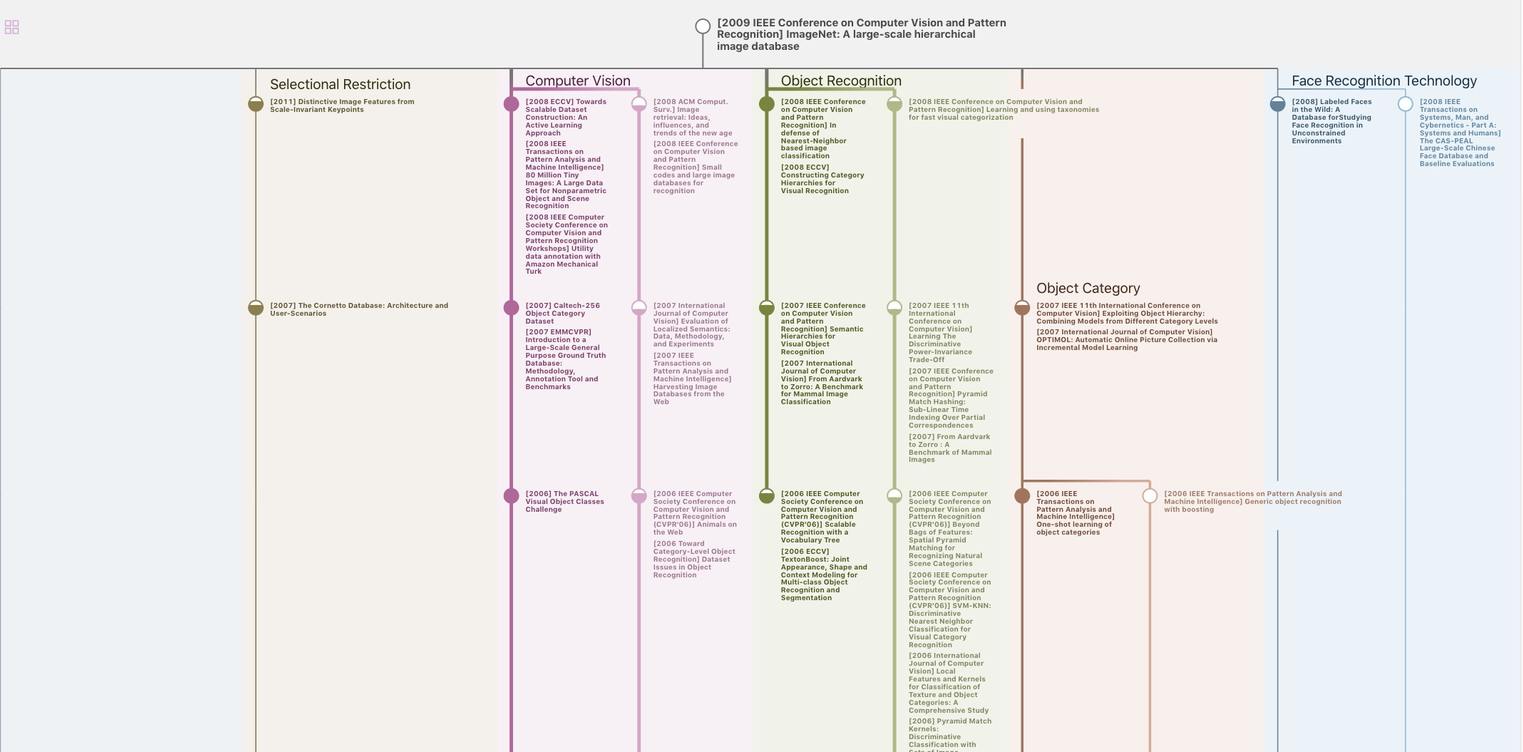
生成溯源树,研究论文发展脉络
Chat Paper
正在生成论文摘要