Functional Connectivity Network Based Emotion Recognition Combining Sample Entropy
IFAC-PapersOnLine(2020)
摘要
Emotion recognition plays an indispensable role in the field of brain-computer interaction. Many researchers perform emotion recognition based on single channel feature extraction, which ignores the information interaction between different brain regions. In order to surmount this limitation, we propose a functional connectivity network based emotion recognition combining sample entropy (SE). Firstly, EEG data of DEAP is decomposed into four frequency bands of θ, α, β, and γ with WPT. Secondly, we build a functional connection network based on the phase synchronization index (PSI) and extract five features, namely global clustering coefficient, local clustering coefficient, global efficiency, character path length, and degree, then SE is extracted and combined with them. Finally, the extracted features are input into the random forest (RF) classifier for emotion classification. The experimental results on DEAP demonstrate that our proposed method is more effective for emotion recognition, and the best classification accuracy reaches 88.58%.
更多查看译文
关键词
Emotion recognition,EEG,Functional connection,Sample entropy,Phase synchronization,Wavelet packet transformation
AI 理解论文
溯源树
样例
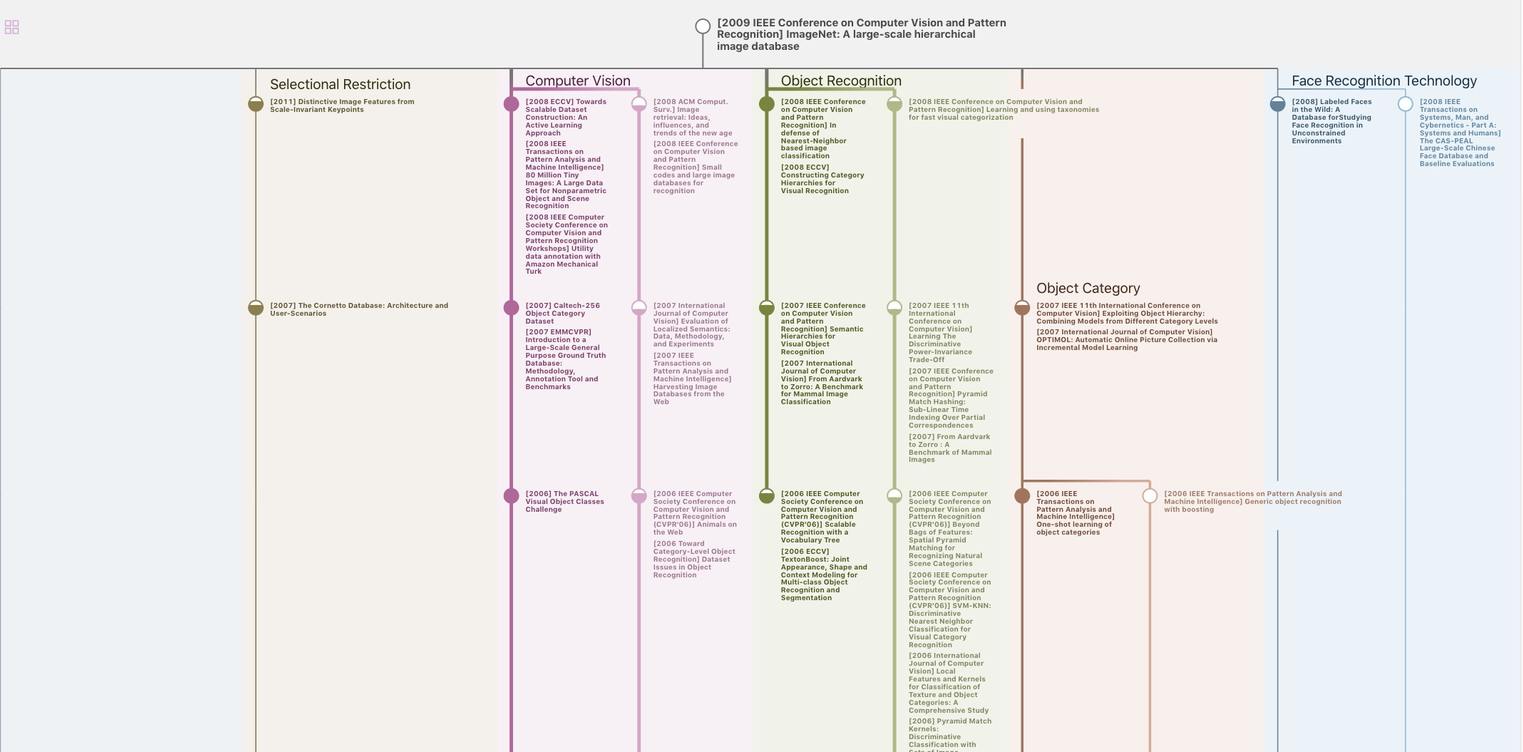
生成溯源树,研究论文发展脉络
Chat Paper
正在生成论文摘要