Soft Computing Based Closed Form Equations Correlating L And N-Type Schmidt Hammer Rebound Numbers Of Rocks
TRANSPORTATION GEOTECHNICS(2021)
Abstract
This paper reports the results of soft computing-based models correlating L and N-type Schmidt hammer rebound numbers of rock. A data-independent database was compiled from available measurements reported in the literature, which was used to train and develop back propagating neural networks, genetic programming and least square method models for the prediction of L-type Schmidt hammer rebound numbers. The results show that the highest predictive accuracy was obtained for the neural network model, which predicts the L type Schmidt hammer rebound number, with less than +/- 20% deviation from the experimental data for 97.27% of the samples. The optimum neural network is presented as a closed form equation and is also incorporated into an Excel-based graphical user interface, which directly calculates the Rn(L) number for any input Rn(N) = 12.40-75.97 and which is made available as supplementary material.
MoreTranslated text
Key words
Artificial neural networks, Genetic programming, Machine learning, Metaheuristic algorithms, Non-destructive testing, Rocks, Schmidt hammer rebound number
AI Read Science
Must-Reading Tree
Example
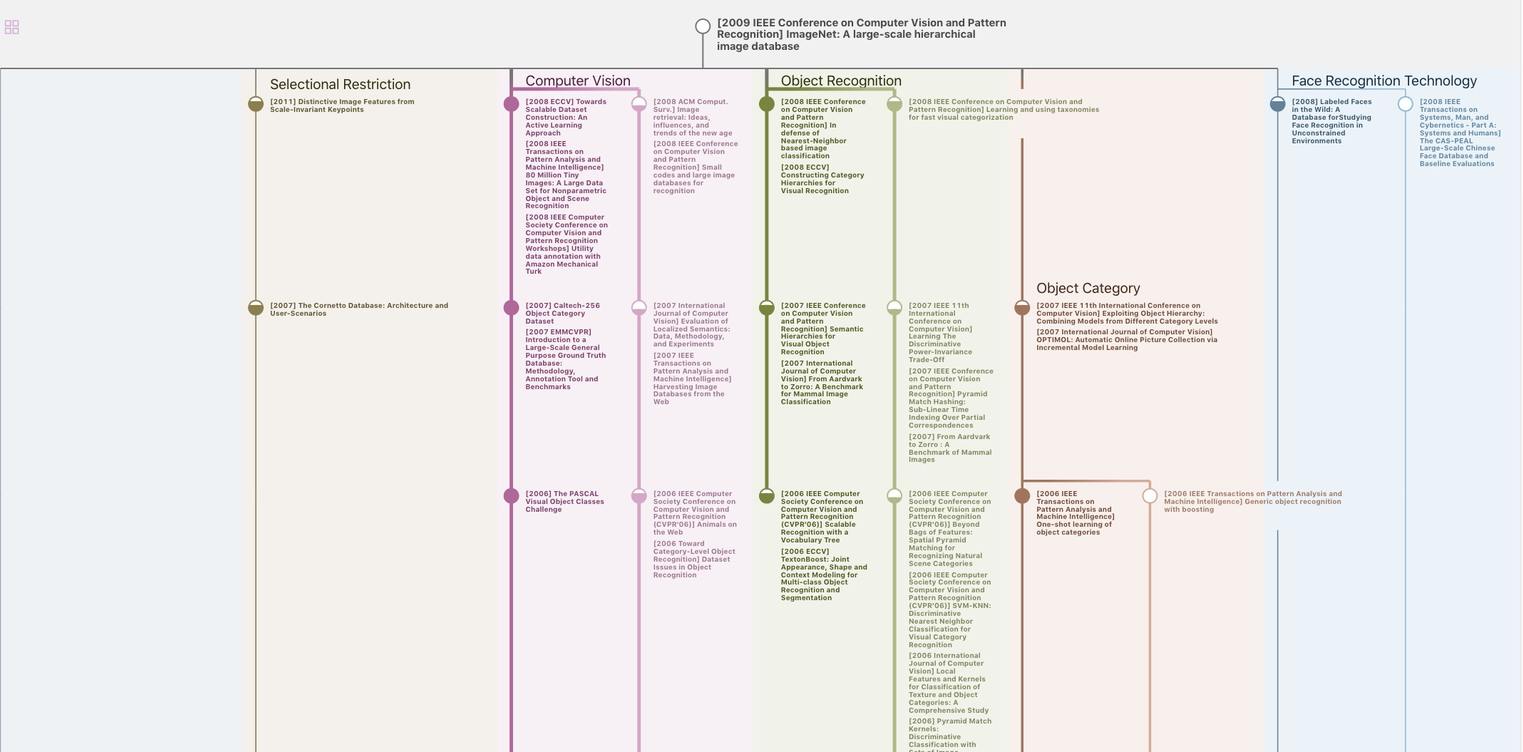
Generate MRT to find the research sequence of this paper
Chat Paper
Summary is being generated by the instructions you defined