Implementation of a Generalized Additive Model (GAM) for Soybean Maturity Prediction in African Environments
AGRONOMY-BASEL(2021)
Key words
generalized additive model (GAM),soybean,Africa,temperature,photoperiod
AI Read Science
Must-Reading Tree
Example
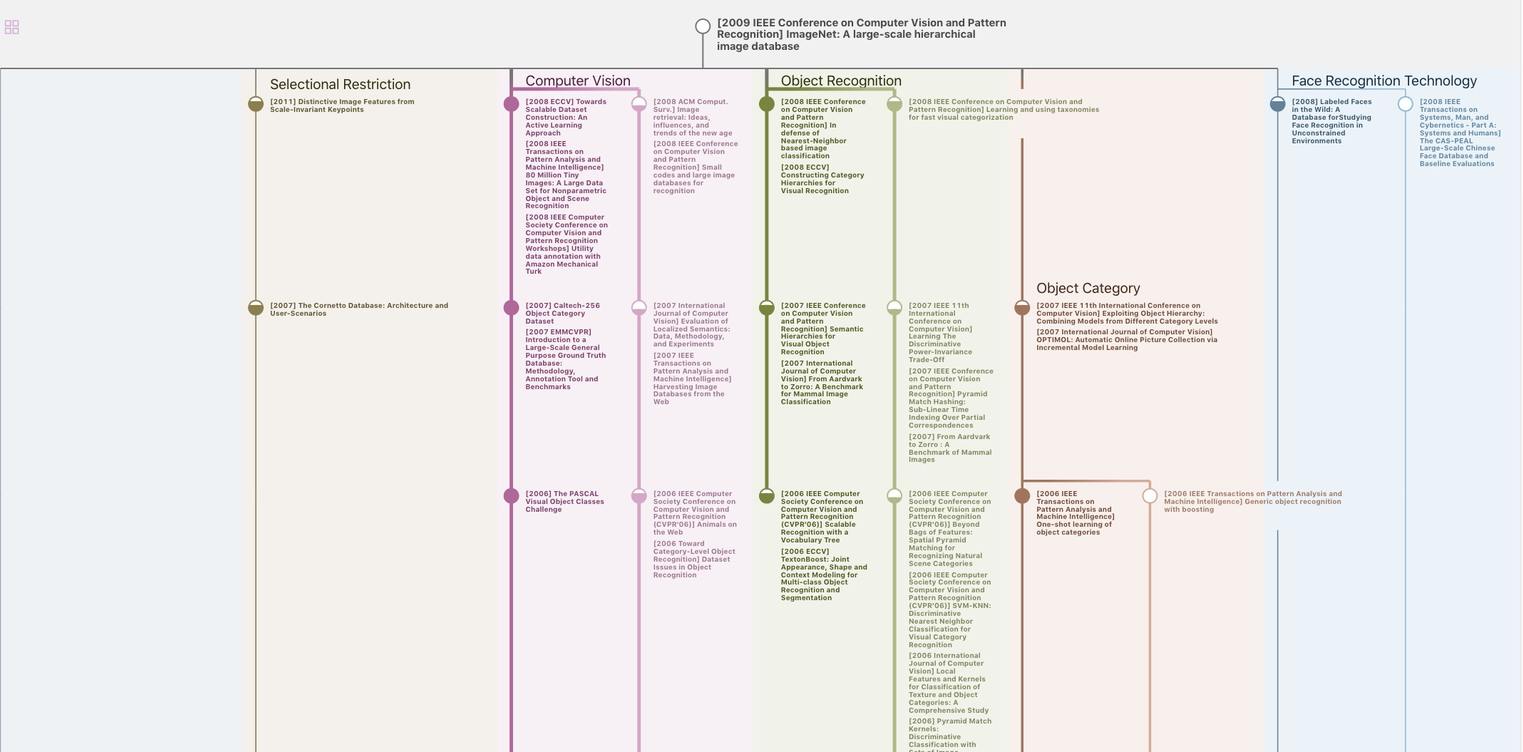
Generate MRT to find the research sequence of this paper
Chat Paper
Summary is being generated by the instructions you defined