Dielectric spectroscopy coupled with artificial neural network for classification and quantification of sesame oil adulteration
Information Processing in Agriculture(2022)
摘要
Adulteration using cheap vegetable oils into expensive oils such as sesame oil is a considerable challenge in the edible oil market. To discriminate pure and adulterated sesame oil with sunflower and canola oils (commonly used as an adulterant to the high-price oils), dielectric spectroscopy was applied in the range of 40 kHz–20 MHz. The principal component analysis (PCA) plots were able to distinguish the pure sesame oil, while it was impossible to separate the adulterated oils based on the kind of adulteration. The correlation-based feature selection (CFS) method was used to select the more relevant dielectric data within the spectrum and to reduce the dimensionality of the input vector belongs to the artificial neural network (ANN). The ANN classifier with topology of 19-5-4 structure showed a perfect accuracy of 100% in detecting the authentic and the adulterated sesame oil. The regression ANN with the topology of 15-5-1, 21-8-1 and 10-11-1 were the most robust models in quantifying the amount of adulteration in sesame oil generated by sunflower oil, canola oil and sunflower + canola oils, with R2Test of 1, 1 and 0.999 9, respectively. The proposed technique is a powerful and simple method to detect and quantify adulteration of sesame oil. The novelty of this research is capability of used system for authentication of adulterated sesame oil using low frequency. Furthermore, the developed system has a good capability for other types of sesame oil adulterations as well as to detect adulteration in other expensive edible oils.
更多查看译文
关键词
Adulteration,Artificial neural network,Chemometrics,Sesame oil,Correlation-based feature selection,Principal component analysis
AI 理解论文
溯源树
样例
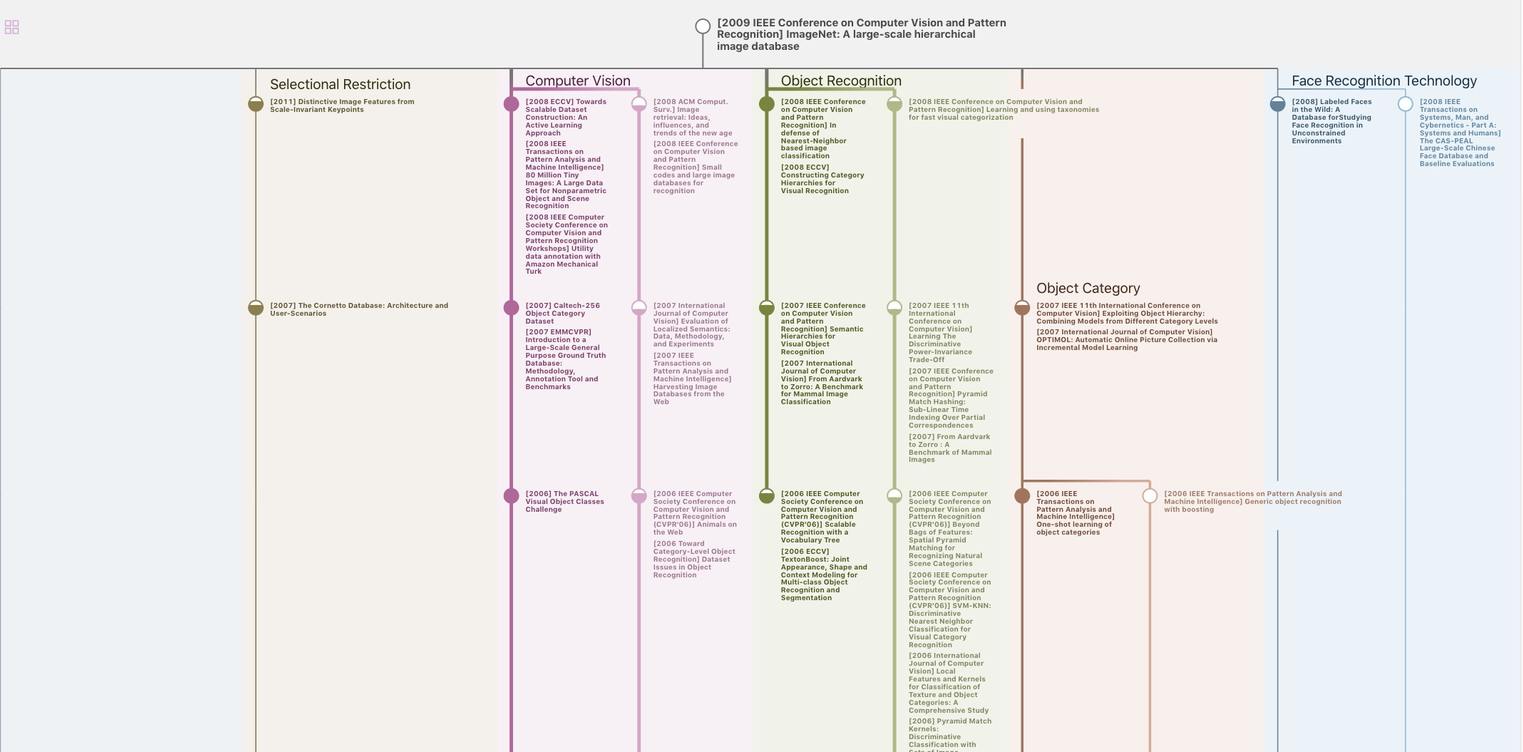
生成溯源树,研究论文发展脉络
Chat Paper
正在生成论文摘要