Machine-learned potentials for next-generation matter simulations
NATURE MATERIALS(2021)
摘要
The choice of simulation methods in computational materials science is driven by a fundamental trade-off: bridging large time- and length-scales with highly accurate simulations at an affordable computational cost. Venturing the investigation of complex phenomena on large scales requires fast yet accurate computational methods. We review the emerging field of machine-learned potentials, which promises to reach the accuracy of quantum mechanical computations at a substantially reduced computational cost. This Review will summarize the basic principles of the underlying machine learning methods, the data acquisition process and active learning procedures. We highlight multiple recent applications of machine-learned potentials in various fields, ranging from organic chemistry and biomolecules to inorganic crystal structure predictions and surface science. We furthermore discuss the developments required to promote a broader use of ML potentials, and the possibility of using them to help solve open questions in materials science and facilitate fully computational materials design.
更多查看译文
关键词
Atomistic models,Computational methods,Molecular dynamics,Theory and computation,Materials Science,general,Optical and Electronic Materials,Biomaterials,Nanotechnology,Condensed Matter Physics
AI 理解论文
溯源树
样例
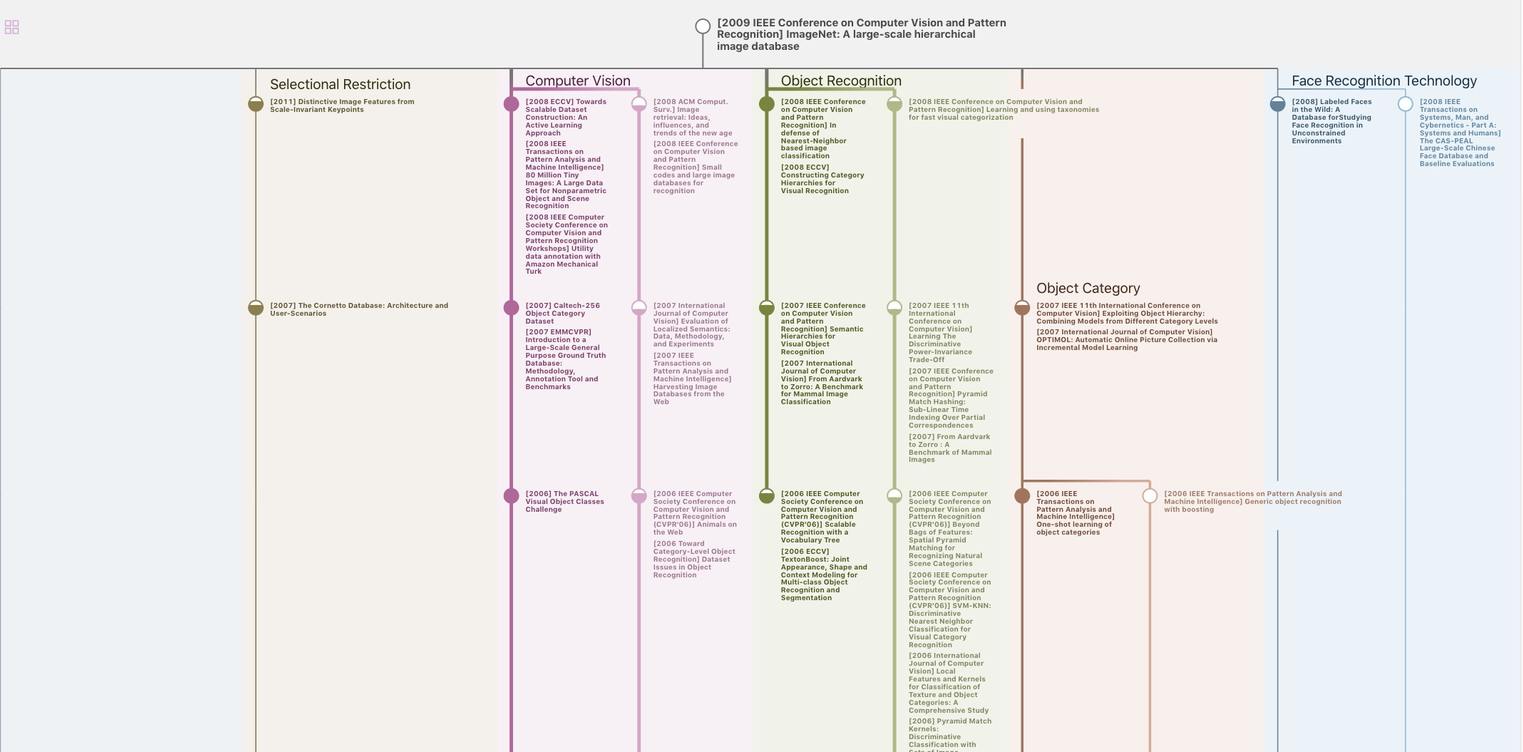
生成溯源树,研究论文发展脉络
Chat Paper
正在生成论文摘要