Comparison By Multivariate Auto-Regressive Method Of Seizure Prediction For Real Patients And Virtual Patients
BIOMEDICAL SIGNAL PROCESSING AND CONTROL(2021)
Abstract
Epilepsy is one of the most widespread neurological pathologies, which is characterized by localized or generalized brain dysfunction due to abnormal or excessive paroxysmal electrical discharges. These discharges lead to neuronal hyperactivities causing prolonged and involuntary muscle contractions or periods of loss or altered consciousness. A patient's quality of life could be greatly improved with an effective alarm system able to predict future crises. Our article presents a fully specified model for automated seizure prediction based on autoregressive multivariate modeling and stability state analysis from clinical and simulated electroencephalography (EEG) data. Our model allows the calculation of a stability index whose analysis in content makes it possible to predict crises. The approach is validated on 29 crises recorded for 8 patients from a database available in free access and 14 simulated subjects also called virtual patients generated by neuro-computer platform TVB (The Virtual Brain:www.thevirtualbrain.org) using Epileptor as mass neural model for gray matter and john Doe" matrix as white matter. Our preliminary results exhibit a detection accuracy of 97.87 %, a sensitivity of 100 % and an average prediction time for the eight real patients of 237.21 s and a detection accuracy of 100 %, a sensitivity of 93 % and an average prediction time of 4.427 s for the simulated subjects.
MoreTranslated text
Key words
Electroencephalography, Epileptic seizures, MVAR, Prediction, Epileptor
AI Read Science
Must-Reading Tree
Example
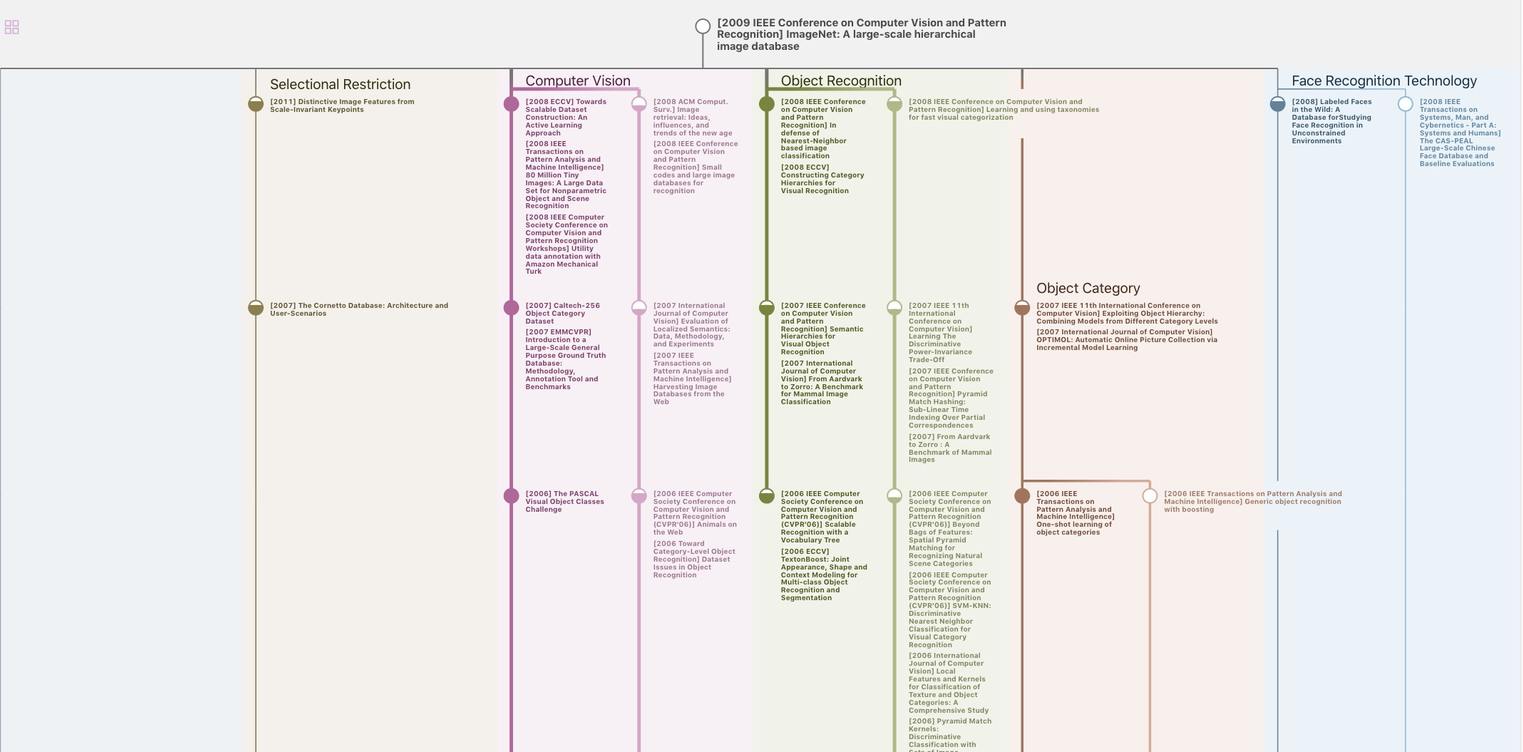
Generate MRT to find the research sequence of this paper
Chat Paper
Summary is being generated by the instructions you defined