Double Differencing By Demeaning: Applications To Hypocenter Location And Wavespeed Tomography
BULLETIN OF THE SEISMOLOGICAL SOCIETY OF AMERICA(2021)
摘要
Double differencing of body-wave arrival times has proved to be a useful technique for increasing the resolution of earthquake locations and elastic wavespeed images, primarily because (1) differences in arrival times often can be determined with much greater precision than absolute onset times and (2) differencing reduces the effects of unknown, unmodeled, or otherwise unconstrained variables on the arrival times, at least to the extent that those effects are common to the observations in question. A disadvantage of double differencing is that the system of linearized equations that must be iteratively solved generally is much larger than the undifferenced set of equations, in terms of both the number of rows and the number of nonzero elements. In this article, a procedure based on demeaning subsets of the system of equations for hypocenters and wavespeeds that preserves the advantages of double differencing is described; it is significantly more efficient for both wavespeed-only tomography and joint hypocenter location-wavespeed tomography. Tests suggest that such demeaning is more efficient than double differencing for hypocenter location as well, despite double-differencing kernels having fewer nonzeros. When these subsets of the demeaned system are appropriately scaled and simplified estimates of observational uncertainty are used, the least-squares estimate of the perturbations to hypocenters and wavespeeds from demeaning are identical to those obtained by double differencing. This equivalence breaks down in the case of general, observation-specific weighting, but tests suggest that the resulting differences in least-squares estimates are likely to be inconsequential. Hence, demeaning offers clear advantages in efficiency and tractability over double differencing, particularly for wavespeed tomography.
更多查看译文
AI 理解论文
溯源树
样例
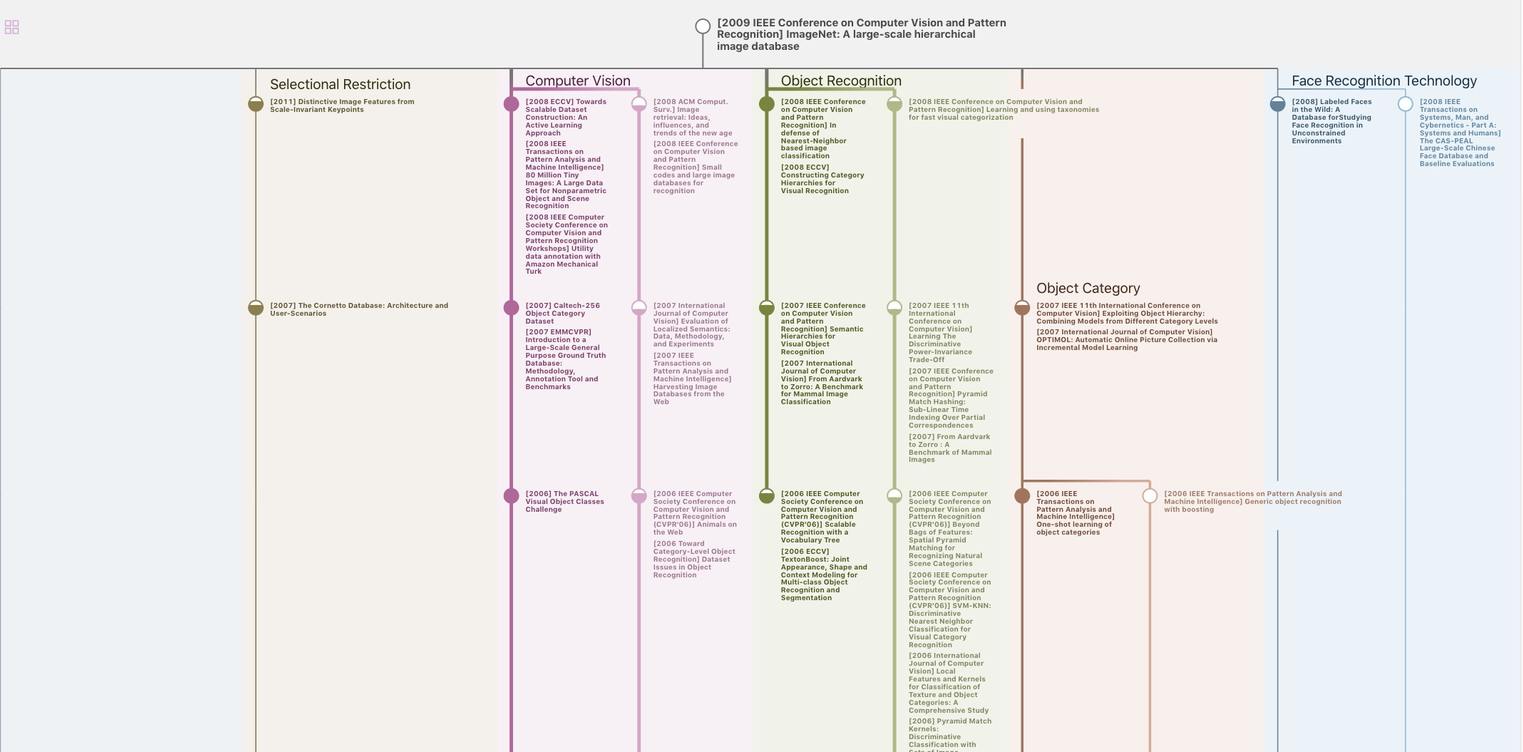
生成溯源树,研究论文发展脉络
Chat Paper
正在生成论文摘要